The Magazine
👩‍💻 Welcome to OPIT’s blog! You will find relevant news on the education and computer science industry.
Search inside The Magazine
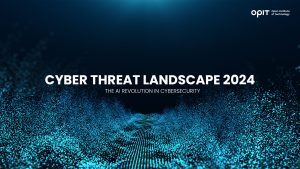
There’s no doubt about it – artificial intelligence has revolutionized almost every aspect of modern life. Healthcare, finance, and manufacturing are just some of the sectors that have been virtually turned upside down by this powerful new force. Cybersecurity also ranks high on this list.
But as much as AI can benefit cybersecurity, it also presents new challenges. Or – to be more direct –new threats.
To understand just how serious these threats are, we’ve enlisted the help of two prominent figures in the cybersecurity world – Tom Vazdar and Venicia Solomons. Tom is the chair of the Master’s Degree in Enterprise Cybersecurity program at the Open Institute of Technology (OPIT). Venicia, better known as the “Cyber Queen,” runs a widely successful cybersecurity community looking to empower women to succeed in the industry.
Together, they held a master class titled “Cyber Threat Landscape 2024: Navigating New Risks.” In this article, you get the chance to hear all about the double-edged sword that is AI in cybersecurity.
How Can Organizations Benefit From Using AI in Cybersecurity?
As with any new invention, AI has primarily been developed to benefit people. In the case of AI, this mainly refers to enhancing efficiency, accuracy, and automation in tasks that would be challenging or impossible for people to perform alone.
However, as AI technology evolves, its potential for both positive and negative impacts becomes more apparent.
But just because the ugly side of AI has started to rear its head more dramatically, it doesn’t mean we should abandon the technology altogether. The key, according to Venicia, is in finding a balance. And according to Tom, this balance lies in treating AI the same way you would cybersecurity in general.
Keep reading to learn what this means.
Top of Form
Implement a Governance Framework
In cybersecurity, there is a governance framework called ISO/IEC 27000, whose goal is to provide a systematic approach to managing sensitive company information, ensuring it remains secure. A similar framework has recently been created for AI— ISO/IEC 42001.
Now, the trouble lies in the fact that many organizations “don’t even have cybersecurity, not to speak artificial intelligence,” as Tom puts it. But the truth is that they need both if they want to have a chance at managing the risks and complexities associated with AI technology, thus only reaping its benefits.
Implement an Oversight Mechanism
Fearing the risks of AI in cybersecurity, many organizations chose to forbid the usage of this technology outright within their operations. But by doing so, they also miss out on the significant benefits AI can offer in enhancing cybersecurity defenses.
So, an all-out ban on AI isn’t a solution. A well-thought-out oversight mechanism is.
According to Tom, this control framework should dictate how and when an organization uses cybersecurity and AI and when these two fields are to come in contact. It should also answer the questions of how an organization governs AI and ensures transparency.
With both of these frameworks (governance and oversight), it’s not enough to simply implement new mechanisms. Employees should also be educated and regularly trained to uphold the principles outlined in these frameworks.
Control the AI (Not the Other Way Around!)
When it comes to relying on AI, one principle should be every organization’s guiding light. Control the AI; don’t let the AI control you.
Of course, this includes controlling how the company’s employees use AI when interacting with client data, business secrets, and other sensitive information.
Now, the thing is – people don’t like to be controlled.
But without control, things can go off the rails pretty quickly.
Tom gives just one example of this. In 2022, an improperly trained (and controlled) chatbot gave an Air Canada customer inaccurate information and a non-existing discount. As a result, the customer bought a full-price ticket. A lawsuit ensued, and in 2024, the court ruled in the customer’s favor, ordering Air Canada to pay compensation.
This case alone illustrates one thing perfectly – you must have your AI systems under control. Tom hypothesizes that the system was probably affordable and easy to implement, but it eventually cost Air Canada dearly in terms of financial and reputational damage.
How Can Organizations Protect Themselves Against AI-Driven Cyberthreats?
With well-thought-out measures in place, organizations can reap the full benefits of AI in cybersecurity without worrying about the threats. But this doesn’t make the threats disappear. Even worse, these threats are only going to get better at outsmarting the organization’s defenses.
So, what can the organizations do about these threats?
Here’s what Tom and Venicia suggest.
Fight Fire With Fire
So, AI is potentially attacking your organization’s security systems? If so, use AI to defend them. Implement your own AI-enhanced threat detection systems.
But beware – this isn’t a one-and-done solution. Tom emphasizes the importance of staying current with the latest cybersecurity threats. More importantly – make sure your systems are up to date with them.
Also, never rely on a single control system. According to our experts, “layered security measures” are the way to go.
Never Stop Learning (and Training)
When it comes to AI in cybersecurity, continuous learning and training are of utmost importance – learning for your employees and training for the AI models. It’s the only way to ensure all system aspects function properly and your employees know how to use each and every one of them.
This approach should also alleviate one of the biggest concerns regarding an increasing AI implementation. Namely, employees fear that they will lose their jobs due to AI. But the truth is, the AI systems need them just as much as they need those systems.
As Tom puts it, “You need to train the AI system so it can protect you.”
That’s why studying to be a cybersecurity professional is a smart career move.
However, you’ll want to find a program that understands the importance of AI in cybersecurity and equips you to handle it properly. Get a master’s degree in Enterprise Security from OPIT, and that’s exactly what you’ll get.
Join the Bigger Fight
When it comes to cybersecurity, transparency is key. If organizations fail to report cybersecurity incidents promptly and accurately, they not only jeopardize their own security but also that of other organizations and individuals. Transparency builds trust and allows for collaboration in addressing cybersecurity threats collectively.
So, our experts urge you to engage in information sharing and collaborative efforts with other organizations, industry groups, and governmental bodies to stay ahead of threats.
How Has AI Impacted Data Protection and Privacy?
Among the challenges presented by AI, one stands out the most – the potential impact on data privacy and protection. Why? Because there’s a growing fear that personal data might be used to train large AI models.
That’s why European policymakers sprang into action and introduced the Artificial Intelligence Act in March 2024.
This regulation, implemented by the European Parliament, aims to protect fundamental rights, democracy, the rule of law, and environmental sustainability from high-risk AI. The act is akin to the well-known General Data Protection Regulation (GDPR) passed in 2016 but exclusively targets the use of AI. The good news for those fearful of AI’s potential negative impact is that every requirement imposed by this act is backed up with heavy penalties.
But how can organizations ensure customers, clients, and partners that their data is fully protected?
According to our experts, the answer is simple – transparency, transparency, and some more transparency!
Any employed AI system must be designed in a way that doesn’t jeopardize anyone’s privacy and freedom. However, it’s not enough to just design the system in such a way. You must also ensure all the stakeholders understand this design and the system’s operation. This includes providing clear information about the data being collected, how it’s being used, and the measures in place to protect it.
Beyond their immediate group of stakeholders, organizations also must ensure that their data isn’t manipulated or used against people. Tom gives an example of what must be avoided at all costs. Let’s say a client applies for a loan in a financial institution. Under no circumstances should that institution use AI to track the client’s personal data and use it against them, resulting in a loan ban. This hypothetical scenario is a clear violation of privacy and trust.
And according to Tom, “privacy is more important than ever.” The same goes for internal ethical standards organizations must develop.
Keeping Up With Cybersecurity
Like most revolutions, AI has come in fast and left many people (and organizations) scrambling to keep up. However, those who recognize that AI isn’t going anywhere have taken steps to embrace it and fully benefit from it. They see AI for what it truly is – a fundamental shift in how we approach technology and cybersecurity.
Those individuals have also chosen to advance their knowledge in the field by completing highly specialized and comprehensive programs like OPIT’s Enterprise Cybersecurity Master’s program. Coincidentally, this is also the program where you get to hear more valuable insights from Tom Vazdar, as he has essentially developed this course.
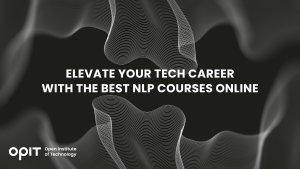
Natural language processing, or NLP for short, has been making waves for years, but as of late, it has caught the attention of even non-tech enthusiasts. Why is that? Simply put, natural language processing bridges human language and computer understanding to make interactions feel more natural and less like talking to machines.
For tech professionals, NLP skills have grown from “nice-to-have” status a few years ago to some of the most significant skills of the decade. The field is growing quickly, and many are interested in taking on the challenge. Luckily, many resources online can get you there and are flexible, in-depth, and practical.
Understanding Natural Language Processing
Natural language processing is an element of artificial intelligence (AI) that deals with the interaction between computers and humans using natural language.
Traditionally, human-computer interactions have largely been done through predefined commands within terminals or graphical user interfaces that obscure the “commands” behind graphical interactions. These interactions work well and aren’t going away. However, instructing a computer by “speaking” to it has been within the realm of science fiction for decades but has also been a research goal of many computer scientists for a long while.
The goal of natural language processing is to read, decipher, understand, and make sense of human language in a valuable way. Recently, it has been integrated into everything from search engines figuring out what users are searching for to translating languages on the fly. It’s even a part of predictive text that finishes sentences, particularly on mobile phone keyboards. Taking a course in NLP gives you a new skill for the CV that opens doors to many employment positions across sectors.
Choosing the Right NLP Course Online
When searching for the right NLP course, consider what you need. Specifically, focus on these:
- Curriculum
- Instructors
- Recognition
- Experience
Does the curriculum cover the latest in NLP technology? The field evolves fast, sometimes with several breakthroughs or at least advancements a year. Course material and curriculum that’s several years behind might miss some of the new developments.
Are the instructors seasoned professionals? The more experience one has in the field, the better equipped they are to pass that knowledge down.
Is the NLP course recognized by industry leaders? It isn’t a matter of appealing to authority but rather knowing that the course is of high enough quality to be considered valuable and useful.
And let’s not forget about the hands-on experience. You can’t really learn NLP just by reading about it. It would be best to try your hands in real-life projects and workshops.
Most NLP courses will walk you through the basics of machine learning, algorithms that power NLP, and hands-on projects that solidify your knowledge.
OPIT offers a full NLP course as a part of the Master of Science (MSc) in Responsible Artificial Intelligence program. The course gives you a solid theoretical foundation and plenty of hands-on experience, presented by instructors who are experts in the field. The degree teaches you how to use NLP and use it ethically and responsibly.
A List of the Best NLP Online Courses
Here are some standout NLP online courses:
- Coursera’s Natural Language Processing Specialization is for intermediate learners and spans over four months. It covers logistic regression, naive Bayes, word vectors, sentiment analysis, and more. The program is a comprehensive one that combines theory with practical assignments.
- Stanford Online’s Natural Language Processing with Deep Learning focuses on the cutting-edge intersection of NLP and deep learning. It’s an in-depth exploration of NLP’s fundamental concepts and its role in emerging technologies. This course is perfect for those looking to get a solid foundation in NLP from one of the leading institutions in the world.
- edX Natural Language Processing Courses & Programs: edX provides an intro to NLP that covers core techniques and computational linguistics. Topics include text processing, text mining, sentiment analysis, and topic modeling. It’s a great starting point for beginners and offers a broad overview of what NLP entails.
- DeepLearning.AI’s Natural Language Processing in TensorFlow on Coursera was designed by one of the pioneers in AI education. It offers practical insights into implementing NLP techniques using TensorFlow. The course covers processing text, representing sentences as vectors, and building NLP models.
- Udacity’s Natural Language Processing Nanodegree is project-focused with hands-on NLP learning. It covers foundational NLP concepts, including text processing, part-of-speech tagging, and sentiment analysis.
While these are the best natural language processing courses online, OPIT’s MSc in Responsible Artificial Intelligence, including NLP as part of its curriculum, is unique. This program teaches you NLP and embeds it within the broader context of artificial intelligence development, AI ethics, and responsible use. It’s excellent for those who want to go beyond the technical aspects and consider the societal impacts of their work in AI.
Benefits of Enrolling in an NLP Online Course
Attending an NLP course online might give you more than traditional on-site education. One of the biggest advantages is flexibility. You can learn at your own pace and on your schedule.
Online courses also open up networking opportunities with peers and mentors from around the globe. These are connections that on-site education would not have the scope to provide. Moreover, completing an NLP course can significantly boost your career prospects, potentially leading to job promotions and salary increases.
NLP Certification and Career Opportunities
With this certification, you’re proving your ability to understand and manipulate natural language data, making you invaluable in roles from data science and AI development to UX/UI design and content strategy.
Companies, from tech giants to startups, are on the lookout for professionals who can bridge the gap between human communication and machine understanding. The demand also translates into diverse job opportunities, competitive salaries, and the potential to work on groundbreaking projects in AI, machine learning, marketing, research, finance, and customer experience, among others.
Natural Machine Communication
NLP leads many of today’s technological advancements, making skills in this area more valuable than ever. Natural language processing courses that equip students with skills in natural language processing, AI, and related fields are growing in both offer and popularity. Completing one of these NLP courses sets you on a course for a financially promising career path within one of the most prestigious tech fields.
Get the right education and get ready for the future. Check out OPIT’s NLP course offerings within the MSc in Responsible Artificial Intelligence program or as a subject within our other computer science degrees.
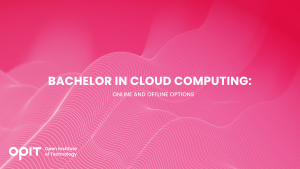
Cloud computing has quickly become one of the fastest-growing industries. The U.S. Bureau of Labor Statistics estimates that the demand for roles in the industry will grow much faster than the average for all occupations. This means that students today will likely be able to find a career in cloud computing much faster than usual. To further illustrate the point, Indeed currently lists more than 8,000 job openings for cloud computing roles.
Despite that, many companies are seeking only top talent, which quickly reduces the available options and drives up demands (as well as salaries). If you want to get a lucrative job in the industry, you must have the appropriate skillset to match.
However, a general background in IT may no longer be enough. A dedicated cloud computing bachelor degree will provide you with the exact abilities you need to excel in these roles and will allow you to upskill to senior positions quickly.
Why Choose a Bachelor in Cloud Computing?
One of the most common misconceptions about programming jobs—and, by extension, cloud computing—is that you don’t need a degree to land a job.
While you can technically get a job in IT without a degree and go from there, the path to success through independent learning is often rocky. You may need to spend multiple years honing your skills through non-accredited courses and self-learning videos. Even if you do manage to get a role close to cloud computing, you may have a more difficult time acclimating to specific job requirements, and your progression may be limited without a degree.
On the other hand, a bachelor’s degree in IT or computer science provides an excellent foundational background. While you might not use all the theoretical knowledge you learn, finishing a bachelor’s degree gives you a broad range of expertise you can leverage to zero in on a desired career path. Specifically, for a bachelor in cloud computing, the focus is on learning different programming languages and coding practices to allow you to adapt to any platform you may need to use during your future job.
Furthermore, completing a bachelor’s shows that you have persistence and can apply theory to practice in exams and project work as part of your degree.
Additionally, many institutions that offer a bachelor’s degree in cloud computing also have close connections with nearby companies that require these positions to grow. They can provide internships to promising students even before they finish their studies and keep them on as permanent team members afterward.
Understanding the Curriculum of a Cloud Computing Bachelor Degree
Cloud computing is an extensive term that encompasses pretty much every application that accesses remote servers over the internet. As a result, there have been many implementations of the concept, and several programming languages were developed to leverage it.
A Bachelor of Science cloud computing degree (or computer science in general) will often have multiple courses dedicated to learning programming languages at the start. Later, the curriculum moves to dedicated courses that translate those basics into tangible skills and projects.
In general, here’s what you will need to learn:
- Algebra and advanced mathematics
- Technical English
- Computer architecture (hardware)
- Programming principles
- Programming languages (C, C++, C#, Java, Node.js and Javascript, Python, Ruby, Golang, etc.)
- Algorithms and data management
- Database concepts and management
- Networking concepts
- Application development
- Web development
Additionally, you will likely have courses on machine learning and AI, given how the industry has bloomed around them in the past few years.
Generally, the curriculum for any given bachelor in cloud computing will include theoretical classes first. Later sections or courses will focus more on implementing these concepts in practice.
Alternatively, you can also have courses that more heavily focus on application, such as a bachelor’s degree from OPIT. It covers the theoretical parts as necessary to apply them while students follow practice work and develop projects.
The Best Offline and Online Bachelors in Cloud Computing
Here are some of the best courses and universities you can attend to get a cloud computing bachelor degree.
1. OPIT – Bachelor’s Degree (BSc) in Modern Computer Science
OPIT is one of the leading European higher education institutions that solely focuses on online learning. Due to a more modern design compared to a traditional university, OPIT fully utilizes the benefits of online learning to support students through an array of both theoretical and practical courses.
The bachelor’s degree lasts for six terms and teaches all aspects of computer science, but students can pick elective courses that zero in on cloud computing in later terms. These include cloud architecture, data stacks, cybersecurity in the cloud, and digitalization protocols for converting traditional applications to the cloud. The bachelor’s courses also include an introduction to business management, allowing students to delve into entrepreneurship and become future leaders.
2. Purdue University Global – Bachelor of Science in Cloud Computing and Solutions
Purdue is a U.S.-based university that provides an online four-year bachelor’s degree course. As a degree fully focused on cloud computing, it foregoes most of the basics of computer science. Students learn by following online lessons and applying the theory to practical projects and lab work. Additionally, the program includes project management practices that help students migrate into senior roles.
3. WGU – Cloud Computing Bachelor’s Program
WGU makes its offer extremely lucrative by focusing on some of the most popular cloud computing platforms: Amazon, Azure, and AWS. The program is designed to be completed within three years, with online learning allowing students to accelerate their progress as much as they want. Furthermore, the degree contains over 16 different certificates as part of its curriculum, allowing students to fill in their resumes even before they finish the degree.
4. University of Liverpool – Computer Science With Software Development With a Year in Industry – Offline Degree
The University of Liverpool is one of the top British universities, ranked around 150th in the world. Its computer science degree focuses on teaching theoretical knowledge in the first year, applying that to lab work in the second, and developing projects in the fourth. The third year is dedicated to working in a software development company that works closely with the university. This presents a unique opportunity for students to apply what they learn and develop their skills in real-life scenarios.
5. Morgan State University – Bachelor of Science in Cloud Computing
MSU is a U.S.-based university in Maryland, but it offers a bachelor’s degree in cloud computing as a fully online course. The program is designed for people who are already in the workforce and need a degree to upskill and progress through their careers. It focuses on modern aspects of cloud-based engineering and architecture. The degree lasts three years but contains slightly more general-purpose classes than dedicated courses.
The Online Advantage: Earning Your Bachelor Degree in Cloud Computing Remotely
The advent of online learning has broken traditional barriers to achieving higher education. Since you no longer have to relocate, the price of studying for a bachelor’s goes down dramatically. Furthermore, online classes typically have portions of the coursework as pre-recorded asynchronous lessons. This can be a great option for people with full-time jobs who can’t attend live lessons frequently.
OPIT provides a thorough support system for online students, with regular assessment sessions and thorough career and study advisory.
Career Outcomes With a Bachelor of Science in Cloud Computing
Cloud computing is one of the fastest-growing industries in the world. Most experts in cloud computing have developer or management positions that design and implement applications. Some of the most common positions in the industry include:
- Cloud architect
- Cloud network engineer
- DevOps engineer
- Cloud database administrator
Regardless of the role, cloud computing is a lucrative career and attracts a high salary.
Industry Certifications and Your Bachelor in Cloud Computing
Certifications are perhaps even more important than just having a bachelor’s degree. They are structured tests that showcase that you have the knowledge and practical aptitude for a platform or programming language. Many bachelor’s degrees in cloud computing, including OPIT, will directly provide students with the knowledge necessary to obtain these certifications, and some have the certifications built into the program.
Financial Investment and ROI of a Bachelor Degree in Cloud Computing
Apart from being an exciting career opportunity in terms of growth, the salaries of cloud engineers are also lucrative. A cloud engineer in Germany typically earns around €65k per year. However, salaries in the U.S. can frequently reach six figures.
So, don’t be put off by the high admission fees for cloud computing bachelor’s degrees. Consider it an investment into a comfortable future. OPIT’s bachelor’s degrees ensure access to higher education by keeping admissions low and providing scholarships.
Start Your Career in Cloud Computing With a Bachelor’s From OPIT
By getting a modern degree in cloud computing, you can get skills that will be relevant in the coming decades as the world increasingly turns to web-based applications. OPIT’s bachelor’s degree in modern computer science will provide you with the breadth of knowledge necessary to progress to leadership positions and ensure an excellent career. Go to OPIT’s course page to find out more and enroll today.
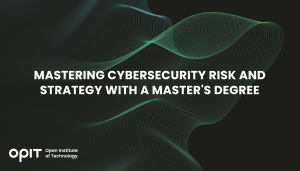
The world has become interconnected by technology and information. The vast amount of data available to companies has also made it increasingly evident that it needs to be stored and protected. It’s no surprise that there are around 2,300 cyberattacks daily, and that number is only expected to rise, seeing that 2023 saw over 70% more attacks than 2021.
These statistics open the door for valuable employment opportunities for specialists in cybersecurity and risk strategy. A cybersecurity expert in Germany can earn between €58k and €85k per year, and the wages in the U.S. are even higher.
Cybersecurity is a relatively vast field that requires both broad IT and management knowledge, but also dedicated experience that correlates to particular job postings. That makes cybersecurity experts difficult to find, as evidenced by the fact that Indeed currently has around 13,000 positions related to the industry.
If you’re already working in IT or planning an IT career, obtaining a master of science in cybersecurity and risk strategy can help you secure a lucrative position.
Understanding Cybersecurity Risk and Strategy
Cybersecurity risk and strategy analyzes the potential for attacks and creates proactive and reactive defenses against them. A complete risk and strategy outline must include all the potential consequences of an attack, such as financial, reputation, and operational losses. In general, a cybersecurity risk and strategy expert will do the following:
- Identify vulnerabilities in the system, whether they come from technical aspects (improper password storage) or human factors (susceptibility to phishing).
- Outline risk factors and the possible rates of attack.
- Create proactive measures, such as implementing more robust security protocols or training personnel on safe online practices.
- Detect attacks once they do come through via intrusion detection systems or other benchmarks.
- Coordinate efforts to contain and remove threats and recover lost data or funds.
A cybersecurity specialist needs to have expert knowledge in various technologies but also solid interpersonal and psychological skills. That’s why a dedicated master’s degree in cybersecurity can help create a complete skillset for the role.
The Curriculum of a Master’s in Cybersecurity Risk and Strategy
A master’s degree in cybersecurity builds upon the IT essentials from a dedicated bachelor’s program. As such, it will likely cover the following:
- Cryptography
- Secure coding practices
- Operating system security
- Network security
- Penetration testing
- Vulnerability assessment
- Government and national cybersecurity
- Ethics, governance, and law implications of cybersecurity
- Systems and security management
- Incident response tactics
However, even more importantly, a good master’s degree program must provide real-life practice assessments. It will allow students to apply the theoretical knowledge and gain valuable experience throughout the curriculum.
Thankfully, online learning has made this type of approach more accessible. Since cybersecurity is web-related by nature, online courses can give students the full breadth of experience and provide more opportunities for a holistic understanding of the subjects and how cybersecurity advances globally.
Take the OPIT Master’s Degree in Enterprise Cybersecurity as a perfect example of this concept. It’s an online-first master’s program that delves deep into cybersecurity concepts such as network security and intrusion detection, cryptography, and even AI in cybersecurity and systems management. Furthermore, students can sign up for practical internships with some of the industry leaders in data management and cybersecurity systems.
Career Outcomes With a Master’s in Cybersecurity
Since IT is a versatile industry, cybersecurity is no different. Students who obtain a master’s degree in this field can have a slew of openings available to them. Entry-to-mid-level roles include:
- Security engineer: In charge of designing, implementing, and maintaining security protocols.
- Penetration tester: Designs programs that ethically hack into existing systems to uncover exploits and vulnerabilities so they can be patched before malicious hackers can reveal them.
- Security analyst: Analyzes information provided by security systems to uncover possible threats and assist other cybersecurity roles.
Mid-to-senior level roles include:
- Security system architect: Designs and implements secure IT infrastructures. Architects can specialize in one specific sub-field, such as cloud, network, or local systems engineering.
- Security manager: In charge of an entire organization’s security systems and implementation.
- Threat response manager: Directly responsible for minimizing the consequences of an active threat or incident.
- Cybersecurity compliance officer: Ensures that the company follows the most recent ethical and legal standards in implementing proactive measures.
- Chief information security officer (CSO or CISO): A leadership position for broad cybersecurity management in larger corporations.
The Online Advantage: Pursuing Your Master’s at OPIT
If you’re interested in a career in cybersecurity, you might have been discouraged to find expensive or prohibitive local colleges. While in-person lessons have their merits, not all colleges are created equal and provide modern knowledge and practice to sharpen students’ skills and prepare them for work.
That’s why OPIT has designed an all-online master’s degree in enterprise cybersecurity. It’s a fully accredited three-term course providing broad and relevant knowledge in modern cybersecurity mechanics.
However, what sets OPIT apart from traditional online degrees is its close relationship with industry leaders. This is emphasized by the complete support from the institution staff and a close-knit community from its digital campus. The courses are a mixture of pre-recorded content that students gain full access to as well as live classes with guests from companies that can share their experience with cybersecurity measures.
As such, OPIT focuses on teaching students relevant skills and how to apply them in real-world situations. Additionally, the course doesn’t have a final exam but provides periodic assessments through projects and assignments to ensure what you learn sticks.
The master’s degree can last between 12 and 18 months, depending on whether you want to take the classes at an accelerated rate. The admission cost is €6,750, with discounts if you apply and pay the entire fee early. The application process is fully online. You need a background in STEM or a bachelor’s degree in a relevant field and be proficient in English.
Becoming a Leader in Cybersecurity
Ultimately, the aim of a master’s degree is to provide students with relevant skills and experience to advance in their careers (or make a significant change).
Apart from teaching technical subjects, the degree focuses on creating situations where students have to apply critical thinking. As mentioned, modern cybersecurity has a significant human factor, so students will also need to develop their interpersonal and management skills if they want to advance to senior-level roles.
OPIT’s master program allows students to partake in interactive projects that will test their newfound knowledge and allow them to flourish in controlled environments with full support from the faculty. This will help reinforce their knowledge and allow them to be more adaptable in the future.
Learn From the Best With OPIT
Since cybersecurity is a rapidly-advancing industry with extreme potential for growth, prospective IT specialists need to be proactive with their learning. Online courses such as OPIT’s master’s degree in enterprise cybersecurity provide all the relevant skills and experience to create a foothold for a successful career in the industry.
Take the next step in your career and upskill yourself with OPIT. Click here to learn more and apply.
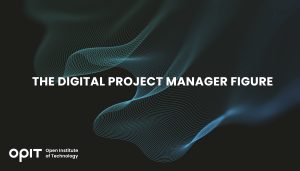
Project managers face the unique challenge of bringing multiple aspects of a job together, from personnel to assets. Digital project management requires the same skills but over many more streams of data and work. As a digital project manager, you might have to handle DevOps teams, data integration, and a wealth of online marketing information. Digital transformation means the opportunities for digital project managers are increasing — if you have the right skills and qualifications.
OPIT’s BSc and MSc courses provide the right foundation for building your digital project management career. Let’s take a look at what you can expect from this career choice and the best pathways to success.
The Role of a Digital Project Manager
What does a digital project manager do? Their roles and responsibilities vary depending on the industry they’re in. For example, a project manager in a game development company will have different workflows to manage than their counterpart in manufacturing. However, many duties remain common.
Digital project managers ensure every member of the team is delegated the right task and knows exactly what to do. Typically, they’ll use project management software, including collaboration tools and time management solutions. These software platforms may also empower project managers to divide larger projects into smaller tasks and assign assets to each task appropriately.
Another key responsibility of project managers is to monitor the timeline of projects. They’ll have to consider how long tasks should take and how that impacts overall completion. This involves setting and tracking deadlines, plus dealing with obstacles such as absenteeism, technical glitches, or client requests. Since this requires a great deal of inter-team and intrateam collaboration, digital project managers must have excellent leadership skills.
Digitally focused professionals are most likely to find work in:
- Digital marketing or advertising
- Enterprise software implementation
- Digital transformation projects
- Web or mobile app development
- SaaS (software as a service) development
As these factors are now embedded across multiple industries, digital project managers could find work in multiple fields. For example, many healthcare facilities are upgrading their patient data management systems to digital alternatives. Statistics show that globally, healthcare organizations are spending $1.3 trillion on digital transformation — a figure that’s continuing to grow. Digital project managers could find work helping implement electronic health records (EHR) and ensuring the smooth rollout of associated processes.
Digital Project Manager: A Typical Day
A digital project manager or PM will have their own daily routine:
- Start the day with a quick update from all team members — you might do this face-to-face or give a time slot for people to drop their updates in virtually. You’ll ensure all remote team members and contractors/freelancers are included.
- Check what tasks need to be completed today/this week and that you have all the required assets and personnel available. You might have to liaise with other teams or gain sign-off from change management professionals.
- Ensure someone has updated the client on the current progress of the project. You may have digital project management tools that provide automatic updates.
- Deal with obstacles and challenges as required. Listen to team members and ensure you remove as much friction from processes as possible. You may have to facilitate meetings between different areas of the business, or clients and project team leaders.
- Prioritize deliverables. As the digital PM, you get to decide what gets done first. Just remember to document the reasons. The board, client, or other stakeholders may need this data at some point.
- Utilize data from multiple streams to aid with prioritization and delegation. Digital project management requires knowledge of the skills and experience of team members. You must be able to share work fairly to avoid overburdening employees while considering their strengths.
- Resource management: you may have to liaise with HR, finance, or the client regarding allocating budgets and gaining relevant personnel and assets.
- Each day will also involve careful documentation of progress. Project managers are responsible for providing clear digital communication channels for all project stakeholders.
There may also be industry-specific daily responsibilities. To go back to the healthcare example, ensuring the security of data as per HIPAA requirements might fall under the scope of project management.
Skills Required for Digital Project Management
Whatever industry they work in, all digital project managers require the following skills:
- Technical knowledge of various systems, apps, and software
- Data analysis and pattern recognition
- Data visualization
- Excellent communication
- Problem-solving
- Leadership
- Mediation
- Time management
Project managers must be able to make tough calls. They’ll need to pivot when strategies aren’t working or reallocate team members at a moment’s notice.
OPIT’s courses help prepare prospective project managers for their role by nurturing these skills. The BSc and MSc courses in Digital Business both include segments on organizational behavior, project management, and quality analysis. By gaining both theory and hands-on practical work on these topics, students get the preparation they need for these demanding roles.
Career Path for Digital Project Managers
There are many paths to becoming a digital project manager, but they all require education and experience. As the PM has so much responsibility, most enterprises require applicants to have a BSc degree at the minimum. However, employers know that education only paints part of the picture. You might follow these steps to gain success in digital project management:
- Gain your qualification through an accredited education provider like OPIT.
- Seek internships to gain experience.
- Network and create contacts via conferences, webinars, and industry events.
- Research where opportunities exist and apply for roles that match your skills and career ambitions.
- Keep a document of everything you do and update your CV regularly.
Digital project managers are highly sought after in various sectors. Software developers with DevOps and DevSecOps teams often seek digital PMs to manage increasingly distributed systems and teams. Businesses looking to update their cybersecurity policy may hire project managers to ensure this gets done in a timely and efficient manner. Also, look for opportunities in industries undergoing dramatic digital transformation efforts. You might consider healthcare, finance, real estate, or agriculture. Sustainable digitalization is a hot topic in farming right now across Europe. Digital project managers passionate about environmental concerns might find job satisfaction in this industry.
Salaries for digital project managers range from €76K to €137K ($83K to $150K).
Your OPIT course can help open many career doors. We encourage internships and provide elective additional units that may align with your career goals. We also have a dedicated career services team to support students in realizing their dreams.
Challenges in Digital Project Management
A key aspect of gaining success in digital project management is recognizing and handling challenges:
- Managing increasingly remote and disparate teams.
- Providing team members with continuous education on rapidly evolving emerging technologies.
- Scope creep — where client change requests cause project parameters to veer outside the originally agreed scope.
Digital project managers need excellent communication skills and the confidence to approach these challenges head-on. To prevent scope creep, for example, digital PMs must be able to say “No,” to clients. They have to set reasonable expectations while giving unwanted answers a positive spin. Alternatively, they may build a sliding cost into a project allowing clients to request more on a pay-as-you-go basis.
OPIT’s curriculum is highly focused on producing similar practical solutions. Every course involves getting hands-on so students are always trying out new things and learning what works in each situation.
OPIT’s Bachelor’s and Master’s in Digital Business
What can you expect as a learner on an OPIT course? The Bachelor’s Degree in Digital Business covers business fundamentals, project management, business strategy, and much more. It’s aimed at undergraduates looking to combine digital fluency with business acumen. This course costs €2,250 per term and is fully remote.
For graduates looking to learn advanced skills and further their careers, the Master’s Degree in Applied Digital Business is the next natural step. This course dives further into digital project management and digital transformation. Students learn about the interplay between digitalization and business, and are highly encouraged to pursue an internship with a trusted industry partner. The cost for this course is €6,750 and dedicated students can complete a fast-track option in just 12 months.
On all OPIT courses, you gain access to high-level academics and excellent student support. Our courses combine strong technical skills with the digital business know-how you need to hasten your journey along your chosen career trajectory.
Digital Project Managers are Vital for Business Success
As a digital project manager, you could be the difference between an organization surviving or thriving in its market. You’ll need excellent communication and leadership skills, bolstered by the technical knowledge and experience that comes from the right educational pathway.
If you’re excited to pursue a career in digital project management, take a look at OPIT’s course offerings. Our goal is to help you succeed, so get in touch if you need more information.
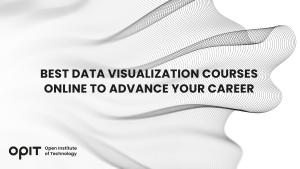
Data visualization is an essential skill in all areas of business and industry. The ability to take complex data and present it in a simple way speeds up decision-making and increases business agility. No wonder so many tech professionals are considering upskilling themselves with an online data visualization course.
If you’re a graduate looking for the best data visualization courses online, you might consider the Open Institute of Technology (OPIT). OPIT offers a Master’s Degree (MSc) in Responsible AI that includes a full segment on data analytics and visualization within the context of AI and other data sciences.
Let’s take a look at more reasons to start an online data visualization course and the benefits it can bring.
The Power of Data Visualization
Businesses thrive on high-quality data. The world generates 463 exabytes of data daily, much of which flows through busy organizations. While experienced data scientists may be able to gain snapshot analyses from complex datasets, most people can’t. That’s where data visualization comes into its own.
A data science and visualization course teaches students how to collect, cleanse, and analyze data before visualization transformations. This includes turning data into graphs, maps, or other graphic displays.
Data visualization turns raw data into usable insights. These insights allow business leaders to take action, change marketing campaigns, budget allocations, or even hiring policies. The ability to quickly see what needs to change allows businesses to edge ahead of competitors.
Choosing the Right Data Visualization Course
The best courses for data visualization teach these skills without taking busy IT professionals or data managers away from their current careers. Online courses provide flexibility and accessibility, allowing learners to study when it’s convenient for them.
Consider the following factors when choosing data visualization online courses:
- Duration
- Topics covered
- Support and student community
- Accreditation
- Career-aligned skills
- Certification
OPIT’s online courses cater to professionals with busy schedules by providing a high-level curriculum entirely online. OPIT is also fully EU-accredited and provides students with internationally recognized qualifications.
The Best Data Visualization Online Courses
Choosing data analysis and visualization courses online is tricky with so many options available. Here are five of the best currently available. Always take the above points on choosing the best online data visualization course into account before committing to a program of study.
Data Visualization with Python
This course from IBM via the Coursera platform looks exclusively at how to use the Python programming language for data analysis and visualization. Classed as intermediate level, some basic data management and programming knowledge is assumed. Tech professionals may find this course useful for upskilling themselves and learning some foundational data visualization skills.
Provider: IBM
Duration: 19 hours
Fees: $39 per month which includes access to other related courses
Qualification Gained: Digital course-specific certificate
Data Visualization Nanodegree Program
Udacity presents this “nanodegree” as a collection of four courses. The program introduces and then expands on data visualization and storytelling. Students will learn design principles, how to use Tableau, dashboard planning and design, and how to build a data story. There are also topics on data limitations and biases.
Provider: Udacity
Duration: Five months
Fees: Either month-to-month at $249 or four months for $846 (minimum $1,095)
Qualification Gained: Udacity Certificate of Achievement
Data Storytelling for Business
One of the few hybrid courses on our list, this storytelling and data visualization course does offer the option for an in-person class. However, you can also complete the program over two virtual seminars, each lasting three hours. This short course focuses on the three “Ds” of data storytelling: Define, Draft, Display, De-clutter, and Direct. It focuses primarily on helping business professionals deliver more effective, impactful presentations.
Provider: StoryIQ
Duration: One day in-person or six hours online with a follow-up session after four weeks
Fees: $230
Qualification Gained: Digital certificate of completion
Hands-On Tableau Training for Data Science
Tech professionals who want to get more out of Tableau could sign up for this software-specific data visualization online course. Tableau is a popular platform for creating data dashboards and is often used for business intelligence (BI) purposes. Students will learn about different types of visuals including charts, maps, graphs, and tables, with table calculations. There’s also a deeper dive into data aggregation and granularity.
Provider: Udemy
Duration: There are nine hours of lectures to complete at your own pace
Fees: $99 for this course but the platform has various subscription options available
Qualification Gained: Udemy Certificate of Completion
OPIT MSc in Responsible AI
For those searching for the best courses on data visualization for graduates, a master’s degree is usually the next step. OPIT’s Master’s Degree in Responsible Artificial Intelligence covers multiple AI-related topics, including data analysis and visualization. Students learn about the challenges associated with handling large, complex datasets. They cover data preprocessing, cleaning, and using that data to tell effective stories.
Provider: OPIT (EU-accredited higher education provider)
Duration: The fast-track option takes 12 months and the standard pathway takes 18 months
Fees: €6,500 — scholarships and discounts are available
Qualification Gained: Globally recognized MSc, equivalent to a Level 7 qualification worth 90-120 ECTS
Key Components of a Comprehensive Data Visualization Course
How do you choose which course is right for you? Your search should start by deciding why you want to take a data science and visualization course. If it’s simply for the joy of studying and learning new skills, one of the shorter courses might suit you. However, if you’re a graduate working in tech already, upskilling yourself will probably require investing in an MSc or similar-level course.
Here are some advanced topics tech professionals to look out for:
- Exploratory data analysis
- Crafting data pipelines in multiple programming languages
- Handling intricate datasets
- Data cleansing, processing, and integration
- Creating visualizations from multiple streams of data
- Linear and nonlinear dimensionality reduction
These skills can help you get ahead in your career by giving you the tools to work with data in any organization. Advanced data science skills are transferable and system agnostic, allowing you to apply for more roles at higher salaries.
OPIT’s Approach to Data Visualization Education
Why study with OPIT? Our unique teaching methods and course structure are deliberately career-aligned. We want to support busy professionals moving forward on their chosen trajectory. The best data visualization courses should allow you to work at your own pace, around your existing commitments.
Our teaching faculty is packed with top-notch academic leaders. We believe that choosing the right team makes the differences between good and great education. On the OPIT MSc in Responsible AI course, for example, you get to learn from Panagiota Katsikouli, a computer science researcher at the University of Copenhagen. Other top-flight faculty members include Pierluigi Casale, a principal data officer for TomTom, and Raj Dasgupta, an AI/ML research scientist at US Naval Research Laboratory.
Your course structure will include a balance of theory and practical hands-on activities. Students start with foundational theory, and then quickly learn how to apply this in real-life situations. For data visualization, expect to start with collating and cleansing data and move on to advanced analysis and presentation techniques. All courses are competency-based, with no final exams to stress about. You acquire new skills as you progress, making these courses ideal for career-minded tech professionals.
Integrating Data Visualization With Other Data Science Skills
Data visualization isn’t a standalone skill. That’s why integrating it with other data science topics such as AI and machine learning is essential. You want a skillset you can apply within your career, which means learning how it relates to various other aspects of data management. Data analysis is normally a primary step in effective visualization. However, analysis isn’t possible without first collating and processing data. The best data analysis and visualization online courses should naturally teach students how data visualization works with other data skills.
OPIT’s courses achieve this by empowering students to create industry-relevant data dashboards, pipelines, and stories. The MSc course culminates with a thesis, which is a research endeavor related to the student’s career ambitions. Learners are also encouraged to pursue internships to practice their skills and gain experience to help them achieve their career goals.
Make Sure You Choose the Right Online Data Visualization Course for Your Career
Choosing the best online data visualization course is essential to optimize your time and learn relevant skills. Make sure you understand the time commitment, cost, and qualifications you’ll gain at the end.
It’s also important to make sure you choose a trusted, accredited educational provider. OPIT’s accredited online programs could take you one step closer to your professional goals.
Explore the OPIT course offerings for more information on how we can further your tech career.
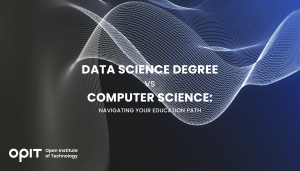
What you study typically dictates your future career. Picking an academic subject is a decision that pairs your passion with practicality, particularly in the computer science and data science fields.
If you’re at a crossroads between choosing one or the other, think about which path aligns with your interests and gives you the best chance of building a bright digital future.
Understanding the Core of Computer Science
Computer science is the backbone of technology. This field prepares you for understanding how software and systems work. It teaches the basics of coding and the complexities of algorithms and network security, all within the same field.
It’s a broad discipline with a knack for problem-solving and innovative thinking. When you master it, you might be crafting the next big app or securing cyber spaces for major companies.
Diving Into Data Science
In contrast, data science zooms in more closely on the digital age’s most precious resource: data. A degree in data science equips you with the knowledge to sift through mountains of information and extract insights that can be used in various industries. For example, it could help healthcare professionals uncover patterns in patient care, sports agents devise new strategies based on big data, or businesses to plan out a targeted marketing campaign.
Data science is about pattern recognition, predictive modeling, and telling stories through data visualization. It’s where statistics meet strategy and empower those in decision-making positions with actionable intelligence.
Comparing Curriculums: Computer Science vs Data Science Degree
Both degrees share a foundation in math and analytical thinking in terms of curricula. Regardless, they have distinct differences:
- Computer Science students are immersed in programming languages, software engineering principles, and computing theory. Their tasks consist primarily of building, designing, and optimizing systems.
- Data Science coursework, on the other hand, mixes together statistics, machine learning, data visualization, and ethical considerations in data handling. It focuses on the lifecycle of data analysis, from collection to communication.
- Each curriculum imparts the basic and advanced technical skills and fosters critical thinking. Once they graduate from either course, graduates will have the means to handle complex problems with creative solutions.
Career Trajectories: Data Science Degree vs Computer Science
Graduates from both fields are in high demand, but the roads they travel can look quite different.
- Computer Science aficionados might be developing software, protecting users against cyber threats, patching and upgrading existing systems, or designing new computing hardware.
- Data Science experts are likely to take on roles like data analysis, predictive modeling, or AI and machine learning engineering.
Fortunately, neither choice will leave you wanting in terms of salary. The sectors are thirsty for the talent and prepared to pay well for the best talent. The salary shouldn’t affect your choice, but whether your passion lies in creation versus analysis.
For instance, in Germany, you’re looking at an average salary of about €50,000 ($54,635) as of 2024. When you compare these numbers to the tech field in the U.S., salaries in countries like the U.K., Poland, France, Germany, and Spain range from 34% to 63% of what their counterparts make in the U.S. If you’re in the tech industry in Europe, what you take home can vary quite a bit depending on where you are.
In the U.K., the average salary for data scientists as of 2024 is around $67,254 per year, with potential additional compensation bringing it up to $79,978. Meanwhile, in Germany, the median salary for a data scientist is just slightly less, around €66,000 ($72,111) per year.
Educational Prerequisites and Learning Outcomes
Before enrolling into either of these fields, you must have a solid base in mathematics and a talent for problem-solving. More specifically, computer science aspirants should get ready for high-level programming, so basic familiarity with programming logic, languages (any would help), and algorithms will do wonders. Just as importantly, you should also have a strong grip on logical reasoning.
Furthermore, data science enthusiasts will need to have a solid understanding of statistics and a knack for critical thinking. Graduates from both fields emerge as tech-savvy professionals who can tackle tomorrow’s challenges with a deep understanding of tech nuances.
OPIT’s Approach to Technology Education
OPIT is at the heart of technology education. The service offers MSc in Applied Data Science and AI and BSc in Modern Computer Science. Both programs have the future in mind, yours and of that of the tech industry as a whole. The programs mix theoretical knowledge with hands-on experience to meet the demands of the job market.
They diverge in focus but converge in aim: to forge skilled professionals ready to make an impact. Best of all, the programs set themselves apart from the traditional classroom education with personalized study that you can do at your own time, without constrictive exams. Instead, the programs focus on continuous learning.
Making Your Decision: Factors to Consider
Now, while you might have a better understanding of what each field represents, there’s a lot more to it. The choice between data science and computer science hinges on a few factors:
- Decide if you are more interested by the prospect of developing software or deciphering data patterns.
- Think about where you see yourself in the tech industry and the type of projects that excite you.
- Keep an eye on the future, understand which skills are likely to remain in high demand, and whether they suit you.
- These considerations can put you on track for a degree that fuels your passion and boosts your career prospects.
Two Options, One Choice
Data science and computer science degrees are both lucrative, in demand, creative, and engaging careers. More than simply academic choices, they will determine what professions you can enter and your future opportunities. Ultimately, your interests, skills, and strengths should decide which path you take. Both pay well and both reward hard work, so choose wisely. Either way, the possibilities are vast and continue to grow by the day.
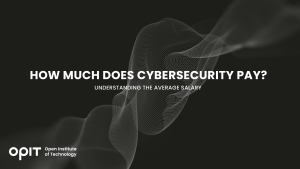
Cybersecurity jobs are better paid than you might expect. Given the skyrocketing significance of the data protection industry, that shouldn’t come as much of a surprise.
If you’ve been wondering what cybersecurity experts take home at the end of the day, this article will reveal the numbers involved. After all, knowing what you could earn gives a clearer picture of whether this field would be worthwhile endeavor for you or not.
Factors Influencing Cybersecurity Jobs Salary
First, let’s look at what makes one cybersecurity job pay more than another. The distinction goes beyond how good an individual is with computers or their knowledge around firewalls, proxies, and the like. Location is also a big factor. Living in or around tech hubs usually means higher pay, but also much higher living costs.
There is also the factor of experience to consider. The more years in the field, the more your salary will grow.
Also, your education level and qualifications matter, too. However, whatever your skill or education level, demand for cybersecurity talent is high. Businesses everywhere are looking for cyber defenders. Therefore, skilled professionals are in a position to negotiate for high pay for their services.
Cybersecurity Salary Overview: Entry-Level vs. Experienced Workers
So, how much does cybersecurity pay? It’s worth noting that you might not make a huge sum right away. But the growth potential within the field is impressive. Entry-level salaries are decent, but as you climb the ranks, your salary will increase accordingly.
It will also depend on the type of job you do. Penetration testers, security analysts, or CISO chairs all have different salaries at different levels. The main thing to remember is that pay will often reflect your skills, so honing them is the main way to help your future earnings soar.
In Europe, starting figures for cybersecurity salary are around €37,000, and can grow to significant sums as you climb the ranks, especially in roles like CISOs where salaries can reach upwards of €180,000.
How Much Do Cybersecurity Professionals Make Globally?
If you’re thinking of taking your cybersecurity talents on a global tour, know that the cybersecurity average salary varies widely around the world. The figures are influenced by local market demand, economic conditions, and living expenses. However, here is a thought worth considering: remote work is changing the way payments for these fields are distributed globally. Now, you can live in one country and work for a company in another, potentially earning more than the local rate. For that reason, it’s an exciting time to explore international opportunities and the global demand to make the most of your skills.
But how much do cybersecurity professionals make in Europe? The salaries are excellent, but can vary depending on where you live. For instance, if you’re eyeing a spot as an entry-level cybersecurity analyst in Germany, you might be looking at an average of €52,539 ($57,420) in 2024. The numbers naturally vary based on role, experience, and location.
Entry-level cybersecurity analysts in the U.S. have starting salaries of around $68,202, rising up to $112,000 as a median figure. Meanwhile, a cyber security engineer in Japan can expect an average cybersecurity salary of around ÂĄ6,963,427 (approximately USD $55,300) in 2024
Maximizing Your Cybersecurity Salary Potential
While the earnings sound appealing, you need the skills and knowledge to harness your earning potential. To climb the ladder, you must make strategic choices to earn the perks, more than anything, through continuous education. Since technology moves at breakneck speeds, and cyber threats emerge even faster, keeping up and staying ahead of the game means being a lifelong learner. Put simply, your education doesn’t end once you leave college or complete a course. As such, you should get extra certificates to show prospective employers that you aren’t being left behind.
However, don’t neglect the power of networking, either. It goes beyond an online presence or having a LinkedIn account. Engage with the cyber security community, attend industry meetups, present your own findings and projects, and lend a hand with open-source projects. Get to know people and you’ll gain opportunities you wouldn’t find in a job ad.
If you’re looking for a place to boost the skills and connections, OPIT is here to help. OPIT’s career-aligned online programs can catapult you into higher-earning roles.
OPIT’s Master’s in Cybersecurity
OPIT’s master’s program in Cybersecurity is one of the most efficient ways to gain the skills and knowledge that can propel you into the upper echelons of cybersecurity. The program is more than a traditional academic education in computer science and cybersecurity. It’s a challenging undertaking but rewards you with knowledge that you can apply in real-life circumstances right away, through practical sessions and workshops.
Over the course of this program, you’ll tackle digital forensics, encryption, firewalls, security systems, and also the strategic thinking behind secure network design. After all, cybersecurity thrives on critical thinking in stress-intensive circumstances and being flexible and creative enough to come up with solutions “on the spot.” However, you’ll be well-equipped for these trials by learning from the best in the industry, people who’ve been at the forefront of cybersecurity debate for years.
High Risk, High Reward
The salaries people earn within cybersecurity sphere reflect the major demand in the field and the skills necessary to complete the job effectively. If you play your cards right, you might be protecting the systems and IT infrastructures of major businesses, nonprofits, or governmental organizations. However, to get to that point, you must learn, and never stop learning. Just as importantly, never underestimate the power of networking and maintaining good relationships.
Programs like OPIT’s master’s degree in cybersecurity are some of the best ways to hone the skills from anywhere in the world, learning from the best in the industry, all at your own pace. Give it a try and see how much of a difference it can make.
Have questions?
Visit our FAQ page or get in touch with us!
Write us at +39 335 576 0263
Get in touch at hello@opit.com
Talk to one of our Study Advisors
We are international
We can speak in: