The Magazine
👩💻 Welcome to OPIT’s blog! You will find relevant news on the education and computer science industry.
Search inside The Magazine
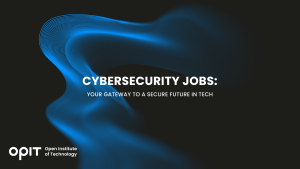
Cybersecurity has been a necessity ever since the advent of digital technology. However, decades ago, few would’ve envisaged just how much demand would eventually grow. Information technology is now such an integral part of modern life, everyone needs to safeguard their digital footprint.
As a result, demand for cybersecurity professionals has never been greater. Let’s take a closer look at both the governmental and private sector positions now available.
The Landscape of Cybersecurity Jobs
The roles within the scope of cybersecurity are broad but united by a common goal: protecting digital assets. Each cybersecurity job relies on another for a comprehensive cyber defense system. Here are some examples:
- Security analysts scrutinize and fortify network defenses.
- Ethical hackers preemptively probe systems for vulnerabilities.
- Incident responders who manage the aftermath of security breaches.
- Security architects who design robust security structures.
- Compliance officers who verify and promote adherence to data protection laws and regulations.
- Malware analysts analyze the nature of malicious software to develop defenses against it.
- Network security engineers take care of the security of an organization’s network from threats and vulnerabilities by installing firewalls and running tests, among other activities.
- Cybersecurity consultants share expert advice to organizations on how to protect their digital assets and follow regulations.
- Information security managers oversee the operations of an organization’s information security department.
- Penetration testers, much like ethical hackers, are authorized to attack systems to find vulnerabilities from an adversary’s perspective.
- Forensic computer analysts, the “detectives” of cybersecurity, investigate cybercrimes by analyzing digital evidence to track down perpetrators and find out the details of a breach.
- Chief information security officer (CISO) is a high-ranking executive responsible for an organization’s overall security strategy against cyber threats.
These professionals are collectively in high demand across various industries. Healthcare, government, finances, and technology, to name but a few. All these industries now depend on valuable documents and client information stored in local databases or the cloud. They also typically boast websites, for both client services and information, which may be targets for malicious actors and attackers. This diversity underscores the universal relevance of cybersecurity, transcending industry boundaries.
Qualifications and Skills for Cybersecurity Careers
Education, certifications, and hands-on experience collectively underpin the path to a cybersecurity job. It’s worth knowing that for you to succeed in the field, you don’t absolutely need a specialized degree, though it certainly helps. However, a bachelor’s degree in computer science, information technology, or a specialist cybersecurity qualification will give you a strong foundation.
Still, the field also places a premium on specialized certifications. Certifications such as CompTIA Security+, Certified Information Systems Security Professional (CISSP), Certified Ethical Hacker (CEH), along with OPIT’s master’s degree in cybersecurity are accolades that signify a professional’s know-how in the field. As such, they will likely put you ahead of the competition for a job position. The competition for cybersecurity jobs is strong, so try and obtain all the qualifications you can get.
OPIT’s MSc in Enterprise Cybersecurity program is one of the best examples of the integration of various fields that link together to create comprehensive cybersecurity measures. It combines theoretical knowledge with practical application, such as simulated cyberattacks, or problems modeled after real-world cyber emergency situations. The program covers a broad spectrum of topics, such as network security, threat intelligence, and legal aspects and ethical considerations.
One of the most valuable cybersecurity skills isn’t one that could be passed down through books or traditional lectures. That skill is strategic thinking. Your strategic mind must be ready to come up with actionable solutions in the heat of the moment, possibly as a response to a situation you’ve never seen before. For that reason, expect this ability to be scrutinized at a cybersecurity job interview.
Building a Career in Cybersecurity: Here’s How You Do It
As mentioned, building a career in cybersecurity takes continuous learning and adaptation, even after you land the job. After laying groundwork for understanding the field through formal education and certification, follow up with internships and entry-level positions. This way, you’ll get invaluable hands-on experience in scenarios that aren’t simulated, rather scenarios with real-life consequences. This is your chance to prove yourself and put what you’ve learned to the test while making a difference for genuine clients or employers.
OPIT offers you a curriculum that balances academic rigor with practical relevance. Students taking this course engage in project-based learning, simulations, and internships, gaining exposure to the challenges they’ll face in their professional careers. Furthermore, OPIT’s strong industry connections mean that students have a one-of-a-kind opportunity to network with seasoned cybersecurity professionals and organizations. This kind of access opens doors to possible quick employment opportunities once you’re out of the program.
Trends in Cybersecurity Employment
Technological advancements drive bad actors to find new vulnerabilities to exploit. For example, the recent rise of AI and machine learning has enabled more sophisticated threat detection and response mechanisms. However, these technologies have their weak spots, many of which are substantially different from what cybersecurity specialists are used to handling.
Recent reports warn of “worms” that could be injected into language models to fish for users’ personal data. Another interesting phenomenon is a tool that claims to “poison” an AI’s training data against training on copyrighted art. In the wrong hands, this could cause significant damage.
Furthermore, “traditional” malware isn’t going anywhere, particularly with the rise of ransomware and identity theft in the last few years. The same goes for malicious software installing crypto miners without a user’s consent. The demand for cybersecurity professionals will grow exponentially, with the Bureau of Labor Statistics projecting a 31% increase in employment of information security analysts from 2019 to 2029. A rate much higher than the average for other occupations.
Security for All
The field of cybersecurity jobs is a promising career path for those looking to make a significant impact in the tech industry. As digital threats proliferate, the need for skilled professionals to combat them has never been greater. OPIT’s cybersecurity programs are at the forefront of this challenge, giving students the knowledge, skills, and insights needed to secure a rewarding future career.
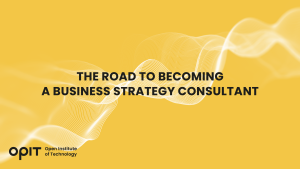
Business strategy consulting is a growing field. Digital transformation is one trend driving the need for consultants who can provide advice and support on areas existing business leaders aren’t familiar with. Emerging technologies like AI and machine learning also offer opportunities for strategy consultants. If you feel like you’ve got your finger on the pulse of modern business trends, it could be a very profitable career choice.
OPIT’s BSc in Digital Business provides an educational pathway to achieving success as a business strategy consultant. If you already have a bachelor’s degree, a master’s could be your next step to gaining thorough proficiency in this field.
Understanding Business Strategy Consulting
Successful companies tend to be very good at what they do, whether that’s providing a physical product or a service. The most successful firms know that for some things, they need external help. Business strategy consultants are usually third-party, outsourced professionals who spend time with the company on a temporary basis. They’re onboarded to deal with specific challenges, from employee churn to reduced profits.
Consultants use their business acumen and digital skills to assess problems and suggest solutions. They may also help implement those solutions, set goals and KPIs, and monitor results. The success of a consultant’s strategy could determine the future of their career. With that in mind, these professionals are highly driven to help the companies they work for succeed.
Specific responsibilities may involve:
- Assessing current budgets and financial processes
- Liaising with executives from CEOs to CFOs
- Driving efficiencies across various departments
- Identifying growth opportunities
What skills does a business strategy consultant need to perform these duties? Ideally, they’ll have a strong grasp of the company’s industry. They also need to demonstrate digital fluency, particularly in data analysis and visualization. Business consultants must also be objective, great listeners, and effective communicators.
Educational Pathways to Business Strategy Consulting
Business strategy consultants need a bachelor’s degree at the very minimum. OPIT’s BSc in Digital Business covers many of the skills needed for success in this role. You’ll learn business management skills alongside computer science. There are sections on managerial economics, project management, and digital analytics — all critical knowledge for a business consultancy role.
Graduates can opt for a master’s degree to hone their skill set. The MSc in Applied Digital Business delves deep into business strategy and its interplay with digitalization. There’s also the opportunity for an internship to gain real-life experience.
Building Your Career as a Business Strategy Consultant
Your degree is a great foundation for your career as a business strategy consultant. However, it’s important to build up from here any way you can. Here’s one step-by-step pathway you could follow to achieve success as a business consultant.
- Take an Internship
As mentioned before, some courses come with the opportunity for internships. Consider seeking time working with existing consulting firms. You might want to think about the industry you’re most interested in and opt for organizations within that sphere. Another option is to approach companies within that market and offer your services to get as much experience as possible.
- Create a Network of Contacts
While you’re finding ways to gain this experience, start building a network of contacts. A business leader who is impressed with your internship now might hire you in the future. Make sure you keep a record of their details. You can also attend conferences, webinars, and industry trade shows. Speak to people, tell them what you do and what your goals are. It’s a great way to meet people with similar interests and make contacts that can help your career.
- Embrace Life-Long Learning
Continuous education is also vital. The digital landscape is always shifting, so business strategy consultants have to keep up with trends in transformation and data management. Seeking out courses that you can complete alongside your existing career is one way to ensure you’re always ahead of the curve.
Key Skills for Success in Business Strategy Consulting
All business strategy consultants need the following skills:
- Knowing how to make tough calls.
- Handling conflict and objections.
- The ability to make snap decisions.
- Utilizing multiple streams of data to make those decisions.
- The determination to see projects through to completion.
- The confidence to take risks and pivot when something isn’t working.
Your OPIT courses support you in the development of these skills. Because you study foundational business skills alongside data science, you learn how to connect analysis to business decisions. You can quickly justify what others might see as brash choices by visualizing the data that led to that path.
OPIT’s Role in Shaping Future Business Strategy Consultants
At OPIT, our courses are helping create a highly skilled cohort of business strategy consultants. The career-aligned courses are designed to meet the demands of the consultancy industry and the digital needs of the companies you’ll work with.
The BSc in Digital Business is a fully remote, online course. The program runs over five terms, with a final additional term for completing your dissertation project. The minimum timeframe is 24 months, but 36 months is the standard length.
You’ll start with business fundamentals, moving on to computer architectures, ICT skills, and web development. More importantly, for prospective consultants, you cover digital business models, business strategy, and critical thinking.
The MSc in Applied Digital Business builds on what you already know. You’ll cover data science and AI and how it fits into the digital economy. From here, you’ll discover business problem-solving, digital project management, and entrepreneurship, among other topics.
After your third term, you’ll start your thesis project, where you’re encouraged to intern with a trusted industrial partner. You can complete this course in 12 months on the fast-track plan, and the regular duration is 18 months.
As well as the high-quality course content and opportunities to work with professionals, you gain access to unparalleled student support. It’s important that students don’t feel alone or isolated during remote learning. With the proper support, online or hybrid study can be more effective than traditional classroom or lecture-based learning. Analysis from the UK shows that 85% of studies on online learning confirm that supportive, digital courses are more beneficial to students than conventional courses.
Combine Digital Fluency With Business Expertise for a Lucrative Career
Your role as a business strategy consultant could change the direction of many companies. The satisfaction you’ll gain from driving success and transformation is second to none. Successful consultants never stop learning, always discovering new ways to uncover business opportunities.
If you’re excited to start your journey towards business strategy consulting, explore OPIT’s available courses or contact us for more details.
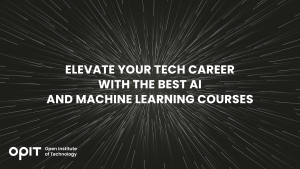
Artificial Intelligence (AI) and machine learning are two of the fastest-growing emerging technologies right now. In late 2022, generative AI burst onto the tech scene in the shape of ChatGPT and its antecedents. However, that’s not the first time AI has made a major impact. In fact, the first AI chatbot, Eliza, was around in the 1960s.
Both AI and machine learning do far more than chat and research. AI is embedded in analytics, predictive forecasting, and monitoring for multiple industries. As the use of AI and machine learning expands, the need for professionals with relevant skills is also growing exponentially.
OPIT (Open Institute of Technology) provides top-tier education in various tech fields, including highly respected machine learning and artificial intelligence courses. Let’s take a look at these fascinating technologies and how the right AI machine learning course can elevate your tech career.
Understanding AI and Machine Learning
When you’re searching for courses on artificial intelligence and machine learning, it helps to have a basic definition for both terms. If you already work in the tech industry, you likely work with one or both of these technologies every day. Yet they’re often so embedded within systems or apps that you might not even realize.
AI refers to the computer’s to exhibit behavior that replicates human thought patterns. However, the details of this definition are a little more complex than that. “Computers” can mean anything from a small subsystem to a supercomputer. It can also mean your smartphone or an app. And, by emulating human behavior, experts don’t necessarily mean AI does things exactly like us. Truly “thinking” AI with genuine cognitive abilities is a long way off.
What AI actually does is take things humans can already do – and do it faster and more often. Think about a software DevOps team requiring automated monitoring and testing of code prior to deployment. AI can do this while checking for vulnerabilities and producing relevant, actionable reports. In healthcare, AI uses pattern recognition to diagnose diseases quickly.
Machine learning is a subset of AI. It focuses on using algorithms to consistently and continuously improve pattern recognition for AI that appears to “learn.”
Courses in AI and machine learning are so popular because of the inherent usefulness of these technologies. Learning these skills now is a way to future-proof your tech career.
The Best AI and Machine Learning Courses
Numerous artificial intelligence and machine learning courses cover different topics and niches. You may choose to learn in a classroom setting or remotely. Some courses are short-term, generally covering foundational aspects of AI. Others carry on over several months for a deeper learning experience. Always consider how the course you invest in will impact your career advancement opportunities.
Absolute beginners may benefit from the Coursera IBM Applied Professional Certificate. This course runs entirely online over three months, presuming you can commit to 10 hours a week. Students learn the basics of AI, particularly how it powers IBM’s Watson AI services.
Oxford Online runs a 6-week online AI program course requiring 7-10 hours of commitment a week. This course looks at AI concepts and business cases for implementation and takes a glimpse at the future of AI.
For classroom-based courses on AI and machine learning, prospective students are best placed to contact local educational institutions. Offline courses vary in length, depth, and usefulness, so always check the syllabus and what certification you gain. It’s worth considering how far you’ll have to travel to gain a qualification.
One of the biggest challenges with AI is making it ethical. OPIT addresses that head-on with the MSc in Responsible AI. Learn advanced AI skills while keeping inclusivity and human interest at the heart of every aspect of the syllabus.
OPIT also offers other courses that consider the impact AI has on modern business practice. Undergraduates could consider the BSc in Digital Business, which includes a full Introduction to AI segment. There are also elective topics, including AI-Driven Software Development.
The Structure of AI and Machine Learning Courses
What should you expect from the best courses on AI and machine learning? Each course has a specific length, either in terms of study hours or a set deadline date. Most online courses have a specific intake date to make sure students get the right support at the right time.
Once you start your machine learning and AI course, you can expect a good balance between theory and practical application. For example, OPIT’s master’s degree course starts with foundational theory and critical thinking around ethics in AI. From here, students get to handle complex data sets. They program in Python and learn how to design effective AI-powered data pipelines.
The structure of your course will depend on the focus, but to give you the best foundation, courses may follow a similar pathway to this:
- Basics of AI, including the differences between AI and machine learning
- Discovering applications of AI — these may be general or industry-specific, depending on the nature of your course
- Data collation, analysis, and visualization
- Programming for AI
- Natural language processing (NLP) and natural language generation (NLG)
- Removing or preventing bias in AI training
Some courses will also offer advanced elective programs, such as understanding AI within the sphere of FinOps (financial operations) or business strategy. If you have a particular industry you’re hoping to excel in, look out for courses with topics that could help you further those ambitions.
Online AI and Machine Learning Courses: Flexibility and Accessibility
Choosing one of the best machine learning and AI courses to do online offers more benefits than new skills. Online learning allows you to study in your preferred environment and at your own pace. You just need to make sure you keep an eye on set deadlines.
You’re not distracted by a class full of people, but you still have access to tutors and support. Many open learning institutes have online communities of students. These are great for preventing isolation and gaining advice.
As a tech professional, the ability to set your own study schedule is essential. Online AI and machine learning courses provide flexibility, allowing you to learn as you work. With OPIT’s Master’s Degree in Responsible Artificial Intelligence, you could potentially have an MSc in 12-18 months without taking any time off work.
Key Skills Gained from AI and Machine Learning Courses
When choosing your online course on AI and machine learning, consider the skills you’ll learn. You should expect to cover:
- Data preprocessing
- Data cleansing
- Data visualization and storytelling
- Linear and nonlinear dimensionality reduction
- Manifold learning
- Human-centered AI design
- Language-agnostic AI programming skills
An MSc in AI and machine learning provides specialized skills and knowledge that you can use to address complex AI challenges in just about any industry.
Choosing the Right AI and Machine Learning Course for You
Picking the right AI and machine learning course is simpler when you consider your goals. Do you want a quick upskill and insight into emerging technologies? Or do you want an immersive course that empowers you to take on new career challenges? Most AI and machine learning courses will provide guidance on the type of career students could hope to pursue after completion.
Always look at the syllabus of a course and see if it meets your personal goals. If you’re unsure about any aspects, contact the education provider for more information.
OPIT’S MSc in Responsible Artificial Intelligence: An Overview
If you’ve decided an online AI and machine learning course is for you, as a graduate, an MSc is the natural choice. The next intake for the OPIT MSc in Responsible AI is September 2024, and details on how to apply are online.
What are the benefits of taking this course?
- A fast-track option to gain your master’s degree in just 12 months
- Fully inclusive fees — no hidden charges
- Various scholarship and funding options
- Availability of early-bird discounts
- Access to academic leaders from all over the world
- Education with an EU-accredited institution
Your MSc course covers every aspect of AI you might require for a career in AI and machine learning. Topics start with AI and ethics and quickly move into human-centered design, computer vision, and how AI impacts IoT and automation.
As you move into your final term, you start your MSc thesis, which focuses on AI projects with industrial relevance. There’s also the opportunity to pursue an internship to complement your thesis and gain vital experience.
AI and Machine Learning Courses for a Future-Proof Career
AI is now part of most growing industries, from property and real estate to healthcare and social care. Tech professionals have the opportunity to upskill themselves and move into fields that they have a real passion for. Organizations are looking for and willing to pay high salaries for knowledgeable, qualified AI experts.
Taking the time now to embark on machine learning and AI courses could speed your journey along your chosen career trajectory.
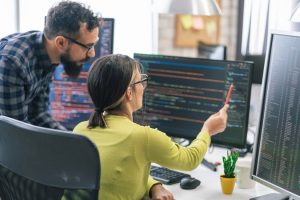
Written on March 21st 2024
Source here: Les Echos Solutions
According to the UN, a cyber attack takes place every 39 seconds! Faced with this omnipresent threat, training in cybersecurity is essential. Whether to master IT security strategies or to manage the digital transformation of your company, OPIT’s programs are adapted to the needs of companies. The Open Institute of Technology offers an innovative range of Bachelor’s and Master’s programs, specially designed to meet the challenges of digital business and cybersecurity. Focus on the different programs that are offered.
The current context of Cybersecurity
In recent years, the CESIN (Club des Experts de la Sécurité de l’Information et du Numérique) cybersecurity barometer has revealed alarming trends. Half of businesses were victims of at least one cyberattack in 2023, compared to a slightly lower rate (45%) the previous year.
The consequences of these cyber attacks are far from negligible. They lead to significant financial losses and an erosion of customer confidence. According to a study carried out by the consulting firm Asterès, the cost of successful cyberattacks on organizations in France reached 2 billion euros in 2022. An average of 58,600 euros per attack!
Investing in specialized training then becomes an essential step to secure the future of your business. A Master’s degree in cybersecurity allows you not only to understand risks, but also to develop effective strategies to anticipate, detect and react effectively to cyber threats.
The digital transformation of businesses
The digital transformation of businesses is an essential process in the contemporary economy. This phenomenon, accelerated by the Covid-19 pandemic, involves the integration of digital technologies in order to improve efficiency, productivity and customer value.
To stay in pole position, you must be able to anticipate trends, continually innovate and adopt a digital-first approach.
It is precisely in this context that a Master’s degree in digital business proves relevant. This program teaches you how to integrate digital technologies strategically and develop new business models to create added value for both your customers and your company.
Programs designed according to your needs
OPIT, for example, offers specialized programs in Cybersecurity and Digital Business, tailor-made to meet the contemporary challenges of the business world. The goal is to help you propel your business to the forefront of innovation and technology while protecting it:
- MSc in Enterprise Cybersecurity: This advanced program is specifically designed to train you in IT security. By integrating a multidisciplinary approach, this master’s degree in cybersecurity explores in depth risk management strategies, advanced cryptography techniques, as well as best practices in information systems security. This program allows you to acquire the skills necessary to effectively protect your company’s data and systems against cyber threats.
- MSc in Applied Digital Business: This program is a gateway to the digital transformation of your business. This master’s degree in digital business is mainly focused on the synergies between technology and business strategies. By exploring the twists and turns of digitalization, this program offers to you a precise vision of emerging trends, innovative business models, and the best strategies to guarantee the competitiveness of your company.
Additional bachelor’s and master’s programs to strengthen your expertise
Beyond courses in Cybersecurity and Digital Business, there are other programs on the market to allow you to develop multidimensional expertise:
● BSc in Digital Business: This bachelor’s program serves as a foundation to the Master’s level to teach you how to merge business acumen and digital fluency, focusing on digital strategies and innovation.
● BSc in Modern Computer Science: This is a program that provides foundational skills, both theoretical and practical, in all aspects of modern computing.
● MSc in Responsible Artificial Intelligence: This program teaches you the techniques for developing and using Artificial Intelligence in an ethical and sustainable way.
● MSc in Applied Data Science: This master’s degree covers business fundamentals, data science, machine learning, artificial intelligence and problem solving.
Online courses: combining professional responsibilities and learning
“The programs of OPIT (Open Institute of Technology), an institution based in Malta, are accessible 100% online. This approach allows you to enrich your skills without interrupting your career or the development of your business. You learn at your own pace, while honoring your professional responsibilities”, indicates one of the managers of the organization.
This learning method also allows the practical application of the knowledge acquired in the professional context.
“OPIT training courses provide a solid foundation for navigating the complex landscape of cybersecurity and digital business. Thanks to comprehensive, innovative study programs adapted to current challenges, you will strengthen your leadership while securing your business”, he specifies.
To find out more: https://www.opit.com/
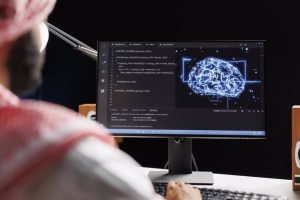
By Open Institute of Technology, March 12th 2024
Source here: EuropaPress
OPIT – Open Institute of Technology, an online higher education institution recognized for its innovation and quality, has recently announced the inclusion of new online Master’s degrees. These programs are focused on the practical and theoretical application of emerging technologies, particularly Artificial Intelligence (AI). This initiative provides students with a comprehensive vision of the latest trends, facilitating their insertion into various disciplines linked to the digital world.
Important to highlight is that OPIT is accredited under the prestigious European Qualifications Framework (EQF), ensuring that its study programs, such as the Master’s in Responsible AI and the Master’s in Applied Data Science and AI comply with European educational quality standards. These accreditations not only validate OPIT’s academic excellence, but also ensure that the degrees obtained are recognized and respected internationally, opening doors to job opportunities around the world.
New growth opportunities in the digital environment
The contemporary work landscape demands more and more specialists in Information and Communication Technologies (ICT), driven by the digitalization of all sectors. ICT experts are essential to innovate, manage complex infrastructures and face current challenges such as Cybersecurity and AI. Having specific specialization in these areas creates diverse career opportunities and competitive rewards, as the ICT specialists contribute to innovation and technological development.
OPIT – Open Institute of Technology offers a complete educational experience, immersing students in the world of ICT and Technology. The Institute has developed Bachelor’s and Master’s programs in key areas of the ICT sector, such as Modern Computer Science, Responsible Artificial Intelligence, Enterprise Cybersecurity and Applied Data Science and AI. These programs are designed to provide not only a solid theoretical foundation, but also practical skils that are highly demanded in the job market.
Intensive Master’s degrees in Cybersecurity and Artificial Intelligence
Cybersecurity is essential to protect our digital infrastructures from increasingly sophisticated threats. OPIT has launched a Master’s degree in Enterprise Cybersecurity that prepares students to implement effective security solutions and lead Cybersecurity initiatives in any environment. This program, like the rest of those offered by OPIT, is accredited according to international standards, which ensures that the knowledge and skills acquired are relevant and applicable globally.
The connection between Cybersecurity and AI is essential to develop a comprehensive understanding of today’s security challenges. OPIT offers a Master’s degree in Artificial Intelligence that integrates the technique with ethical considerations, preparing students to lead at the technological forefront. This knowledge allows us to anticipate and proactively respond to emerging threats, guaranteeing the protection of digital assets in an increasingly complex and dynamic environment. In this sense, OPIT – Open Institute of Technology has perfectly understood the need of the current labor market by offering a training program better known as a Master’s degree in Artificial Intelligence. The program combines a technical approach with the ethics of AI and its advanced aspects and covers fields such as Natural Language Processing, Computer Vision, Sensor Systems and Automation in Industry.
OPIT is a leader in the education of digital and technological disciplines, offering programs that combine theory and practice. Students find in these programs an opportunity to expand their horizons and prepare to contribute significantly to their fields of expertise.
For more details about the accreditation and internation recognition of our programs, visit our website: https://www.opit.com/.
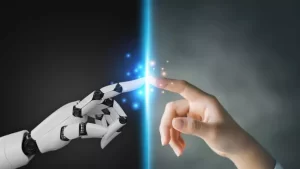
The Artificial Intelligence revolution is now a reality, and the recent appointment of Father Paolo Benanti, a member of the United Nations Committee on Artificial Intelligence, as Chairman of the AI Commission for Information, underlines the importance of addressing this transformation in a conscious way.
The mission today, and with OPIT – Open Institute of Technology we are going in this direction, is to guarantee for the new generations the opportunity to become aware and responsible in facing change. It is precisely for this reason that we have integrated AI into our educational offer, applying it in a transversal way and with different cuts, and our Master in Responsible AI is just one example. In this article from La Stampa, I write my thoughts on the crucial role that AI can play in the higher education sector, where ethics and philosophy will be central elements in the development and use of this technology.
By Francesco Profumo, January 31st 2024
Source here: LinkedIn
Full article available on La Stampa (in Italian)
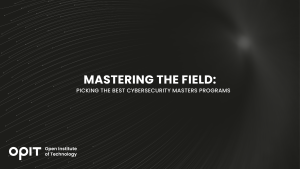
Malicious attacks on IT systems and devices are increasing in complexity and organizations need to be proactive and agile to deal with ever-evolving threats. These threats vary from ransomware attacks and the installation of malware to password theft and phishing.
Organizations want the best of the best when it comes to senior cybersecurity professionals, and demand is growing. In an increasingly competitive job market, those who have graduated from the best cybersecurity master’s programs (such as OPIT’s MSc in Enterprise Cybersecurity) will stand out from the crowd.
Exploring Cybersecurity Programs
The ever-evolving nature of threats makes a career in cybersecurity one of the most exciting in the field of IT. However, the applicant must have suitable qualifications to secure a place at a leading company.
The right program should incorporate advanced master’s level theoretical subjects and practical, experiential learning, and the courses on offer should be at the cutting edge of cybersecurity best practices. This will provide the students with the tools they need to deal with not only current cybersecurity challenges but also emerging threats.
Each qualification has core courses allowing students to choose which competencies would best fit their unique requirements. This makes deciding on the best qualification from the best cybersecurity master’s programs essential.
Criteria for the Best Cybersecurity Programs
When evaluating qualification options, it will soon become clear that the best cybersecurity programs have much to offer. But how do you assess whether your chosen program will deliver the goods regarding career opportunities?
When researching the best cybersecurity master’s programs, keep the following criteria in mind:
- Ensure that the master’s program you choose has course content applicable to your specialty and aligns with other industry certifications you may already have.
- Check if your selected qualification offers courses that balance theory with real-world application.
- Verify that the qualification provider is accredited by an internationally recognized regulatory or accreditation body.
- Make sure the program offers a flexible online study schedule.
Aside from the course offerings, services such as networking opportunities, career advisory, and post-graduate support will be invaluable for job placement opportunities – particularly institutions in partnership with leading cybersecurity firms.
Researching alumni success stories will also give you a unique insight into the program beyond the program’s overview and curriculum.
Best Cybersecurity Masters in the World
Cyber threats are global; they don’t respect borders. In light of this, the best cybersecurity masters in the world will offer a range of internationally accepted coursework, providing skills based on diverse perspectives. This is the program for those who want to build a robust network of business contacts.
Here are five top cybersecurity programs that tick all the boxes:
1. M.S. in Cybersecurity From the University of Tampa – Sykes College of Business
This program may be a good fit for those with an ever-changing schedule because they can study full-time or part-time. However, due to its flexibility, the on-campus degree will take between one and two years to complete. One of its key features is preparation for professional certifications such as the Certified Information Systems Security Professional (CISSP) exam. The program costs approximately $20,960 (about 19,380 euros) per annum.
2. Master of Science in Cybersecurity Management – Nova Southeastern University
Students will devote between one and two years to this qualification, with a mixture of distance learning and on-campus study. Fees are $950 (around 878 euros) per credit. The program focuses on vital skills such as, cybersecurity management communication, organizational policy development, compliance issue management, and risk management principles application to the real-world environment.
3. MSc in Cybersecurity and Public Policy – Tufts University School of Engineering
Coursework for this exceptional master’s qualification takes place entirely on campus, and student fees are $1,730 (approximately 1,600 euros) per credit. Students have the flexibility to complete the coursework in either one or two years. Core coursework includes privacy in the digital age, how systems work and fail, and the role of cyber in the civil sector. This course is ideal for students who want to get on the fast track to a senior management position.
4. UC Berkeley Master’s in Cybersecurity Online
This online qualification is ideal for busy professionals who may find full-time on-campus study problematic, with completion occurring in as little as 20 months. The program allows students to master foundational cybersecurity-related technical skills and speak with authority on the political, business, and legal complexities of the ever-evolving cybersecurity environment.
5. Information Technology Auditing and Cyber Security MS – Temple University Fox School of Business
As another fairly flexible program on the list, this on-campus two-year qualification can be completed full-time or part-time. The program is hands-on, with coursework delivered by industry-level experts. Students learn how to assess business needs and design the internal processes that are so important for the development of robust cybersecurity systems. The course (costing $1,165 or 1,077 euros per credit) is ideal for employment in accounting and consulting firms, computer software and hardware companies, and financial institutions.
Best Online Cybersecurity Master’s
Given the increasing cybersecurity threats that are now a business reality, the best online cybersecurity master’s degrees are in increasing demand. Accordingly, many businesses consider a qualification like this essential for senior management.
Online master’s degrees are an attractive option for the busy executive or someone searching for the ideal work/life balance. They provide accessible, interactive access to state-of-the-art digital platforms that can make studying at your own pace a pleasure. The best cybersecurity master’s programs also offer a combination of theoretical foundations and hands-on experience, all delivered remotely.
Learn more about your options for further online study by clicking on this link: best online cybersecurity programs.
OPIT’s Master’s in Enterprise Cybersecurity: A Cut Above the Rest
The Master’s Degree (MSc) in Enterprise Cybersecurity from OPIT is not only accredited but also conforms to the criteria that elevate a qualification above the standard offerings. Especially notable is the prioritization of real-time industry needs, making it the best cybersecurity master’s degree to obtain. The coursework is the ideal combination of theoretical approaches and real-world experience, all delivered via a platform that is not only easily accessible but also intuitive to use.
Enrolling in the best online cybersecurity master’s, like that from OPIT, ensures students can study at their own pace while learning from industry expert faculty members. The 12–18-month qualification is also recognized by key industry players, future-proofing cybersecurity leaders with the knowledge to implement cutting-edge security solutions and lead world-class cybersecurity initiatives.
Best Cybersecurity Master’s Programs in the USA
The United States is at the epicenter of cybersecurity innovation. This is no coincidence, as it’s home to Silicon Valley and numerous global giants in the tech industry. The demand for qualified degree holders is growing exponentially, but, once again, selecting from the top cybersecurity master’s programs in the USA can be a daunting task. Here are two well-respected options.
1. Online Master’s in Cybersecurity – Syracuse University
Live online classes and coursework that provide professionals with the knowledge required to identify, prevent, and counteract cybercrime make this master’s program one of the best cybersecurity masters programs. The program features cutting-edge research and multidisciplinary collaboration.
2. Master of Science in Cybersecurity – Marshall University
This program is ideal for students who want to pursue a Ph.D. or those who want to reach the pinnacle of their chosen profession. The curriculum covers advanced topics such as cryptography, cybersecurity policy, cyber risk and vulnerability assessment, cyber operation, and software security (among others).
Future-Proof Your Career With OPIT
The most advanced and best cybersecurity master’s programs will equip busy professionals with the specialized skills and knowledge required to thrive in an ever more competitive job market.
Online degrees such as the OPIT Master’s in Enterprise Cybersecurity should be on the bucket list of any aspirant cybersecurity expert. Dominate the field and make significant contributions to the evolution of cybersecurity best practices with OPIT‘s exemplary program.
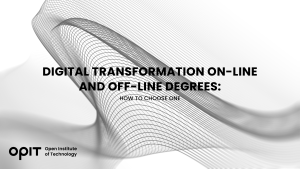
According to Statista, close to 85% of people in the EU use the Internet daily. That’s a huge population that demands a working digital infrastructure and expects online services to function seamlessly. This has resulted in extreme demands for digitizing online services across industries and sectors, with seven of the 10 most prominent companies in the world focusing on digital platforms. The process has been dubbed the Fourth Industrial Revolution.
With the advent of digital-first business accelerated by the COVID-19 pandemic, many companies are seeking experts in digital transformation to adapt to new practices. These positions are highly sought after and compensated accordingly, with the average yearly salary for digital transformation experts in Germany being €96K. In the U.S., similar positions can pay up to $200K per year.
That’s where the best online master’s degrees in digital transformation come in. Outfitted with future-proof knowledge and practices, you, too, can lead companies in making these pivotal changes. What’s important is to choose the best programs—like the one from OPIT—to give you the necessary edge and expertise in this forward-facing career.
Understanding the Value of Online Education in Digital Transformation
At its core, digital transformation is firmly entrenched in technology use and relies on many principles brought out by rapid globalization. All industries rely on technology and digital systems in one way or another, whether those are services, sales, marketing, or even production. And technology is changing more quickly than the educational systems can keep up.
With online education, the curriculum can adapt to the most recent advancements in the industries, from optimized data tracking to AI use. It allows direct access and a deeper dive into the same platforms you’ll be using during practical work. The basic practices you learn during an online curriculum can be immediately applied to real-life work and extrapolated onto custom projects in your career.
Furthermore, online teaching evens out educational opportunities across the globe. So long as you have an internet connection, you can access world-class education piloted by leaders regardless of the institution you wish to enroll in.
Online learning also allows you to set your own learning pace. It’s an excellent option if you’re already a career professional who wants to be more aligned with the needs of the modern world while still working full-time or providing for your family.
What to Look For in Top Online Digital Transformation Programs
With so many online and offline teaching programs that promise state-of-the-art education in digital transformation, it can be extremely challenging to find the best options. Here’s what you should look for in your digital transformation degree or course:
- Curriculum relevance: Digital transformation combines data science, management, and analysis and how technology affects businesses and entrepreneurs, which should be must-haves in the program. Also, look for classes that focus on engineering, finance, project or product management, and marketing.
- Innovative teaching: Due to the subject’s inherent reliance on available technology, opt for programs that have interactive tests, simulations of real-life events and platforms, and real-world practice projects.
- Industry partnerships: Look for programs supported by leading digital-first companies or those that provide internships and real-life projects as part of the dissertation.
- Accreditation: Find programs that are accepted as fully accredited Higher Education Institutions, such as the European Qualifications Framework (EQF), EAHEA, EQUIS, AMBA, and AACSB, or a member of an international body such as ENQA. It will ensure your degree is universally accepted.
- One-on-one teaching: Apart from traditional group teaching, look for courses that allow for dedicated one-on-one supplemental teaching and mentorship.
- Modern concepts: The course doesn’t need to teach just the theory of the currently dominant technologies and practices but also set up future concepts and teach a theoretical basis that accounts for future advancements.
Best Online Masters in Digital Transformation
Here are the top five options for your online digital transformation degree.
OPIT’s MSc in Applied Digital Business
Developed by industry leaders from modern educational institutions and leading companies such as Amazon, the EQF-accredited Open Institute of Technology has built the 18-month course in Applied Digital Business from the ground up. As a result, the course aims to train students for careers in digital transformation, data science, marketing, and business analysis. Due to its online-first nature, the course immerses you into the digital management sphere from the get-go, guiding you via a combination of theoretical analysis and practical projects.
In the first term, the program provides a detailed post-graduate education in data science, digital project management, problem-solving in the digital era, and customer value management analysis. In the second term, the course focuses on digital transformation, platform, and project management, as well as finance in the digital-driven economy. Finally, the course ends with a long-term research capstone project to consolidate your skills with full support from a faculty mentor.
It’s one of the most versatile programs available that will allow you to transfer your existing skills into modern practices and create opportunities for growth in both current and emerging industries. With support from global industry leaders, you get access to a wide network of peers to set you up for success.
University of Hull Online – MSc in Digital Transformation
This is a focused 2-year program in digital transformation, teaching the relevance of emerging technologies in modern digital systems, such as AI, blockchain, 3D printing, and quantum computing. However, the curriculum also contains subjects that touch on information and project management, how customers affect digital businesses, and digital innovation and strategy development. The program is fully online with a part-time commitment and optional group webinars, with the University of Hull accredited by AMBA.
University of Edinburgh Online – MSc in Digital Education
While not a focused master’s degree in digital transformation, this degree in digital education provides a well-rounded theoretical basis for working in the digital sphere. It focuses on how digitization will affect education, making it a lucrative choice for people employed in academia who want to future-proof their skills. It lasts from two to six years of part-time online study, and the university is currently ranked among the top 20 in the world.
ESSCA Online Campus – MSc in International Business 4.0 – Leading Green & Digital Transformation
ESSCA Online is a triple-accredited online school that teaches international business practices, digital transformation techniques, and sustainable operation management. It also provides an overview of blockchain, Web3, the metaverse, project management, fintech, and data analysis. It’s a 12- or 24-month program with case study programs and consulting projects with companies affiliated with ESSCA.
University of South Wales – MSc in Leading Digital Transformation
This degree course focuses on leadership, digital technologies, and innovation in the digital field, with a particular focus on the healthcare sector. However, it also teaches UX design, management within digital-only teams, and agile business practices. The program lasts for two years of part-time study (half a day each week) and is delivered through online real-time courses with guests from leading companies.
Why Opt for OPIT’s Digital Transformation Education Online
Traditional university programs commonly use the same in-person curriculum and just deliver the content online. However, OPIT’s education system is fully tuned towards online learning. You get to interact with lecturers worldwide and learn from industry leaders in real-time or on-demand. The program combines theoretical knowledge on modern topics with practical exams and assignments that put it to the test to ensure it sticks.
OPIT’s curriculum is also designed to be future-proof. The skills you learn in the master’s degree for digital transformation will be useful in solving future challenges with yet-to-be-conceived technology. It ensures that your career can last for years with minimal re-skilling and creates a path of lifetime improvement through practice.
Start Your Digital Transformation Journey
Earning a post-graduate degree in emerging technologies and concepts can push you forward as an expert for years to come. Choose OPIT as one of the best online digital transformation degree options and receive a stellar combination of theory and practice to provide you with real-world skills crucial for career development.
Have questions?
Visit our FAQ page or get in touch with us!
Write us at +39 335 576 0263
Get in touch at hello@opit.com
Talk to one of our Study Advisors
We are international
We can speak in: