The Magazine
👩💻 Welcome to OPIT’s blog! You will find relevant news on the education and computer science industry.
Search inside The Magazine
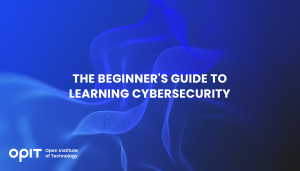
The world is shifting increasingly into an online world with every technological advancement. In this world, only one thing stands between your digital information and malicious actors – the presence of a cybersecurity team.
Cybersecurity professionals are in sky-high demand, and this trend isn’t slowing down. If you’re curious about joining their ranks and want to know how to learn cybersecurity, you’ve landed in the right spot. This roadmap will not only explain what cybersecurity is but how to get started in this exciting field.
Understanding the Cybersecurity Landscape
Cybersecurity might sound like a single, giant puzzle, but it’s more like a collection of smaller puzzles. It keeps the online space safe from threats and hackers, and the field spans various domains.
For example, network security keeps connections safe from attacks that lurk before you even access any website. On the other hand, application security fortifies the apps, while information security guards the data you share and store.
Another cybersecurity domain, ethical hacking, involves breaking into systems (legally, of course!) to find vulnerabilities before the bad actors do.
Learning about cybersecurity starts with getting familiar with the basics, such as concepts and key terms. Then, you have to keep up with the tech and threats, which means dipping into the latest trends in the cyber world.
Getting Started: Cybersecurity for Beginners
If you’re ready to address the question, “How to learn cybersecurity for beginners,” you’ll be glad to know that getting started isn’t complicated. The following steps will get you started in the right direction:
- Step 1: Basic Knowledge. Many resources are easily discoverable online for free. Look for tutorials, blogs, and free courses that introduce the core concepts of cybersecurity.
- Step 2: Formal Education. Once you’re comfortable with your basic know-how, it’s time to dive into structured learning. Paid, full-curriculum online courses and certifications for beginners are more comprehensive. Organizations like CompTIA and (ISC)² offer foundational certifications like Security+ and SSCP.
- Step 3: Practical Experience. Learning theory is a great way to build a solid foundation, but cybersecurity is a hands-on field. You’ll need practical experience, so take part in labs, simulations, and project-based learning like Hack The Box or CyberSecLabs to apply what you’ve learned in the real world.
OPIT’s Role in Cybersecurity Education
OPIT’s cybersecurity program give you a strong base in cybersecurity principles blended with the real-world side of the field with practical, hands-on applications. You will team up with experts who know the ins and outs of cyber threats, the latest tech defenses, and strategies that work.
There’s even more.
OPIT’s lectures and exams are nothing like the typical classroom-style courses you might’ve found at other educational institutions. In the program, you’ll have access to virtual labs and have you work on live projects. You’re being given the keys to a safe cyber playground where you can test skills, make mistakes, learn, and grow without the risk of letting the real intruder in.
Building Essential Cybersecurity Skills
Here are some of the core skills every beginner needs to develop to enter the complex and ever-evolving sphere of digital security.
- Encryption is how information stays safe from prying eyes online. As a beginner, learning encryption means learning how to use these secret codes to protect data and keep it readable only by the intended recipient.
- Network protocols are the web’s traffic rules. Getting to grips with these protocols will help you understand how data travels across the web and how to keep it secure as it does. You learn the pathways and the signposts – HTTP, HTTPS, FTP.
- Cybersecurity picks apart your mindset as much as it does the tools and technical skills. Sharpening analytical thinking is akin to becoming a digital detective. You’ll learn to look beyond the obvious and piece together clues to uncover potential threats before they strike.
- Every day in cybersecurity brings a new problem to solve, like finding a vulnerability in a network or responding to a cyber-attack. Your problem-solving ability to think on your feet and devise solutions will be your greatest asset.
However, while all these skills are invaluable and necessary, there’s one aspect that, if you’re lacking, might set you back from becoming top of the field. The cybersecurity field is as much about connections as it is about computers. By participating in webinars, attending conferences, and joining forums, you keep your knowledge up to date and build a network of peers and mentors. These interactions can inspire new ideas, offer support in tackling challenges, and open doors to opportunities in cybersecurity.
Why Choose a Career in Cybersecurity
Beyond asking how to learn about cybersecurity, you might also wonder why you should. It’s a career path full of excitement, challenges, and the immense satisfaction of making an impact on the world. Here’s why this field is worth considering:
In High Demand
Everything is going digital at an unprecedented rate. And with it, the need for skilled cybersecurity warriors. There’s a constant call for talent capable of safeguarding data and infrastructure against never-ending threats. Stepping into cybersecurity means you’re stepping into a realm where your skills are a shield for everyone’s very existence and functioning online.
Diverse Roles
Cybersecurity isn’t a one-size-fits-all career. It’s a mosaic of roles that cater to different interests and skills. For example, you might be intrigued by ethical hacking, fascinated by digital forensics, or drawn to creating secure networks. There’s always a niche for you. This diversity means you can find a path that plays to your strengths, keeps you engaged, and pushes you to learn more.
Making a Difference
Cybersecurity specialists are protectors. They shield not just bytes and data but people and their way of life. You have the power to prevent fraud, thwart cyberattacks, recover people’s precious data, and protect the privacy of individuals and the secrets of corporations. The impact is real, tangible, and incredibly rewarding.
Be the Cyber Warrior You’re Meant to Be
Cybersecurity starts with getting familiar with the basics and exploring accessible online treasures. You have to layer up knowledge with more structured learning as you dive into courses that challenge you more each time. Then, you get your hands dirty with actual work, where you learn the ropes by doing. The softer, more analytical skills will also be helpful, whether you’re taking time to figure out a complex problem or have to pivot for an immediate threat. And don’t forget to mingle in the cyber crowd—webinars, forums, the works.
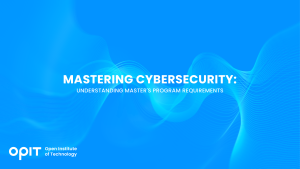
Businesses are under increasing threat from cybercriminals and malicious cyber attacks, a threat that is growing year on year. In 2023, malicious attacks cost U.S. businesses $8 trillion, and those losses are expected to climb to $9.5 trillion in 2024, a steady increase that shows no sign of slowing.
Given this state of affairs, it is no surprise to learn that professionals with a master’s in cybersecurity are in increasing demand. However, choosing the best cybersecurity master’s degree can be a daunting task. There are an increasing number of educational institutions that provide this qualification (or others like it).
However, those wishing to take their qualifications to a new level should be aware of the cybersecurity master’s requirements.
Most institutions will need the prospective student to have previous qualifications, such as a bachelor’s degree or relevant work experience. These requirements differ for each educational institution, and understanding them is key to choosing the right master’s degree in cybersecurity.
General Requirements for Cybersecurity Master’s Programs
Although the requirements to gain admission to a master’s in cybersecurity program vary by educational institution, there are some common prerequisites. These can include:
Prior Education
As mentioned, a recognized bachelor’s degree in cybersecurity is considered an essential stepping stone towards a master’s qualification. However, this is not an absolute. Many educational institutions will evaluate prospective students on a case-by-case basis, and degrees in other fields can count in the applicant’s favor. As a general rule, the student should be able to demonstrate knowledge in areas such as computer science, information technology, or a related field.
GPA Requirements
As a rule of thumb, entry into most master’s programs will require a GPA between 2.5 and 3.0. However, there are exceptions, with some schools requiring much higher grade point averages.
Program Prerequisites
Many educational institutions have stringent requirements on undergraduate courses that they require for the student for admittance to the master’s program. Knowledge of data structures, programming languages, calculus, programming, networks, and systems security concepts will definitely be advantageous.
Letters of Recommendation
Admission can also be influenced by work experience demonstrating a knowledge of softer business skills. These include communication, teamwork, mentoring, and even ethical standards. Many schools will accept letters of recommendation from business leaders, as well as a variety of other testimonials. These will certainly increase the chances of acceptance into the master’s program of your choice, irrespective of other cybersecurity master’s requirements.
Specific Skills and Experience
The importance of prior experience in the fields of IT and cybersecurity when applying for entry to a master’s degree in cybersecurity cannot be overstated. A good track record in real-world implementation is valuable, as is participation in research projects.
Paid internships can be extremely valuable when it comes to admission to the degree of your choice. These internships are also important in demonstrating a commitment to lifelong learning and can contribute to credits toward a master’s qualification.
OPIT’s Cybersecurity Master’s Program Requirements
The OPIT Master’s Degree (MSc) in Enterprise Cybersecurity has several core requirements for admission. These include prior technical experience or proven expertise. However, this requirement does not bar those who lack experience from admission. Applicants who do not have a technical background in the cybersecurity field will undergo an assessment to gauge their foundational IT and cybersecurity skills.
A passion for cybersecurity innovation in an ever-evolving threat environment is as important as prior experience when it comes to gaining entry to the OPIT master’s course. Candidates who demonstrate a commitment to continuous learning will not be hamstrung by a lack of previous working experience when it comes to gaining acceptance into the OPIT postgraduate program.
Preparing for OPIT’s Cybersecurity Master’s
Those wishing to enroll in the OPIT cybersecurity master’s program can ensure that they are prepared for any potential assessment (and the demands of the coursework) in a variety of ways.
Online courses offer a flexible, affordable, and accessible way to gain insights into the cybersecurity environment, and chat groups can provide real-world interactions that can fill any knowledge gaps. Taking part in group chats may also provide mentoring for the aspirant cybersecurity expert.
As part of a commitment to lifelong learning, staying up to date with the latest trends and developments in the cybersecurity field is essential. Subscribe to relevant newsletters and set your news alerts to flag stories about cyber threats and cybersecurity.
Why Choose OPIT for Your Cybersecurity Education?
OPIT provides a fully accredited Master’s Degree (MSc) in Enterprise Cybersecurity that emphasizes integrating theory and practical application in real-world solutions.
The affordable OPIT master’s program boasts a curriculum developed in close consultation with industry leaders and is presented by leaders in the field of cybersecurity. The program is designed to meet and exceed the requirements of some of the industry’s most innovative organizations.
The study experience is streamlined through an advanced online learning environment that is perfect for those who want to take their careers to the next level while enjoying the flexibility to set their own pace when it comes to coursework.
For professionals who want flexibility and demand only the best qualifications, this master’s degree is ideal. An OPIT master’s in cybersecurity is the key to preparing students for leadership roles in the cybersecurity sector.
A Master’s in Cybersecurity – Final Considerations
Research is the key to both successful enrolment and eventual graduation from a master’s degree in cybersecurity.
Students should be aware of cybersecurity master’s requirements before they make a final decision on a degree provider. These requirements will often include a bachelor’s degree or work experience. But soft skills also count when applications are evaluated.
By choosing an OPIT Master’s in Enterprise Cybersecurity any prospective student will enjoy peace of mind. That sense of confidence comes from knowing that the degree they have selected is respected by leading organizations in the cybersecurity field.
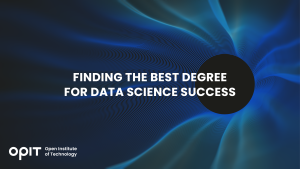
By 2025 the global data volume will approach 175 zettabytes. Store that information on DVDs and the stack would reach the moon 23 times over. That sheer volume of data means that professionals are required to make sense of the information.
Sectors like finance, healthcare, manufacturing, and telecoms use vast amounts of data and present attractive career opportunities. Choosing the best degree for data science can open up new doors for those interested in playing a leading role in the lucrative field of data science.
Understanding Data Science and Its Educational Pathways
Data science has always been important. Businesses have been leveraging the power of data ever since the term was invented, but the data landscape is changing.
Today, data science combines math and statistics, advanced analytics, specialized programming, Artificial Intelligence (AI), and machine learning to provide actionable strategic insights to organizations.
Aspirant professionals interested in playing a data-centric role in the success of an organization need appropriate, respected, and relevant qualifications, and finding the best degree for data science is the first step on the road to success.
That road offers many routes toward success in the individual’s chosen field.
Some data science career trajectories include:
- Data scientist
- Data analyst
- Business analyst
- Business intelligence analyst
Mid-Level
- Data architect
- Data engineer
- Senior business analyst
Senior-Level
- Lead data scientist
- Director of data science
- Vice president of data science
- Chief information officer
- Chief operations officer
What to Look For in a Data Science Degree
A firm foundation is essential for a rewarding career in data science, and that foundation must include a recognized undergraduate degree. The best degree for data science will be obtained from an accredited and well-respected education institution. It will provide foundational skills in areas such as data analysis, machine learning, big data, and statistical analysis (among others).
However, the structure of the coursework is important. An undergraduate degree in data science should:
- Provide a solid understanding of principles and theory
- Offer practical experience based on real-world immersion
- Give opportunities for specialization
In addition, a world-class program will emphasize teamwork, innovation and effective communication and offer the chance to make industry connections.
Best Degree for Data Science: Which One Should You Choose?
Navigating the sometimes murky waters of higher education can be a daunting task, especially when it comes to choosing the best degree for data science, but here are some well-respected choices.
1. M.S. In Data Analytics – Franklin University
This online qualification will equip the professional with the statistical skills required to conduct descriptive and predictive analytics. It also provides the programming skillset necessary to create and apply computer algorithms and the tools and platforms to visualize and mine big data. Students can expect to complete the coursework in around 19 months.
2. Bachelor of Science in Industrial Systems Data Analytics – Lakeland University
The strength of this qualification from Lakeland is its focus on both programming and data management. The flexibility of the on-campus/online program makes it a very attractive option for those who already hold a 9-5 job. This program provides students with essential skills in programming, statistics, data analysis, and visualization.
3. Bachelor of Science in Data Analytics – Southern New Hampshire University
Although this is an online course, the experience of using advanced analytical tools to solve real-world challenges will provide potential employers with peace of mind. Also on offer is a focus on project management, which is essential given the complexities of data-driven projects. Focus areas include data analytics, computer science, and computer programming. The course should take four years to complete, although online delivery allows students to graduate more quickly
4. Bachelor of Science in Computer Science – Full Sail University
This program focuses on data structure and system design. The online and on-campus study option means that students can finish the coursework in less than 80 weeks. Focusing on core competencies such as computer science, computer programming, and data science, it is the perfect qualification for those entering the potentially rewarding world of data science.
5. Bachelor of Science in Data Analytics – Lynn University
The 100% online undergraduate qualification in data analytics can be completed in four years or less. Coursework includes business analytics, advanced business techniques, data programming, and data mining. With a focus on real-world solutions, this is a program that will pay dividends in increased employability in a highly competitive environment.
OPIT’s Bachelor’s and Master’s Programs in Data Science
OPIT’s Bachelor’s (BSc) in Modern Computer Science and Master’s Degrees (MSc) in Applied Digital Business and Applied Data Science & Artificial Intelligence have been designed with input from industry leaders and feature real-world application of the skills gained through study. This approach results in qualifications that are extremely attractive to potential employers.
The BSC in Modern Computer Science
The coursework of the six-term Bachelor’s in Modern Computer Science is delivered entirely using state-of-the-art platforms designed for ease of use and flexibility.
Both potential employers, academics, and industry professionals have had a hand in developing this degree. It aims to provide graduates with theoretical and practical 360-degree foundational skills, including such coursework as programming, software development, database development and functionality. Students will also dive into more complex topics like cloud computing, cybersecurity, data science, and the ever-more important subject of Artificial Intelligence.
The MSC in Applied Digital Business and Applied Data Science & Artificial Intelligence
The 12–18 month Master’s Degree (MSc) in Applied Digital Business from OPIT supplies students with the knowledge and skills to tackle real-world challenges in technology, digitalization, and business. Coursework includes strategically orientated subjects such as digital transformation, digital finance, entrepreneurship, and digital product management. Students will also explore real-world applications with a capstone project and dissertation based on a real-world case study.
The 12-18 month Master’s in Applied Data Science & Artificial Intelligence is at the cutting edge of data science specialization. Online delivery of coursework means that students have incredible flexibility and can complete coursework at their own pace, a boon for busy professionals. Like other OPIT Master’s courses, this program emphasizes foundational principles and courses with content applicable to real-world challenges that can be analyzed using data science and AI. Coursework includes business principles, data science, machine learning, and Artificial Intelligence.
Why Consider OPIT for Your Data Science Education?
OPIT’s affordable, fully accredited, and internationally recognized degrees leverage knowledge from leading academics and industry leaders. This ensures the most relevant course content and resources, all delivered via cutting-edge online platforms. The institute’s flexible scheduling, the blend of theoretical and practical knowledge, and hands-on experience deliver an educational experience unlike any other available today.
The Future of Data Science and the Role of Education
The amount of data that has to be gathered, stored and analyzed by businesses is growing exponentially. This has fueled increasing demand for skilled and qualified data scientists. Employers are looking for the best of the best, and one of the time-proven ways to stand out from the crowd is by obtaining a recognized and respected qualification – the best degree for data science.
Of course, the learning doesn’t stop at one degree. Data science pioneers know the importance of lifelong learning and staying abreast of the latest methodologies, trends, and advancements.
A Data Science Degree – Making the Right Choice
Choosing the best degree for data science can be a challenge, but that challenge becomes manageable when one whittles down the choices. Make sure that the education provider you choose has impeccable credentials and a good reputation. Both of these are based on the delivery of exceptional course content that focuses on both theory and real-world experience.
Employers want graduates who can hit the ground running. Choosing a degree from OPIT means that the employee can start adding real value to organizational strategy from Day 1, and that is what employers want.
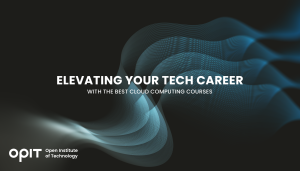
There was a time when cloud computing was nothing more than an interesting concept, but today the cloud computing industry is worth in the region of $371 billion. Businesses are leveraging the power and scalability of cloud computing due to its flexibility, enhanced security and cost savings realized through the reduction of expensive IT infrastructure maintenance.
Those professionals who are intent on becoming leaders in the ever-evolving world of cloud computing need appropriate skills and a world-class qualification, and that means finding the best cloud computing courses.
Understanding Cloud Computing and Its Courses
Cloud computing focuses on the delivery of computing services over the internet. These services can include database management, server hosting, storage, networking, online software solutions, and analytics. A recognized qualification in cloud computing will incorporate course content covering these areas and is essential for cloud innovators and future leaders.
Discovering the Best Cloud Computing Courses
A career in cloud computing provides a wealth of choice. There are qualifications that will prepare the student for a career as a Cloud Architect, Cloud Engineer, developer, or consultant. There are also more specialized careers involving cloud security or operations management. The key to a successful career in any of these fields is selecting the best cloud computing courses and qualifications.
Qualifications that will put the aspirant cloud expert on the fast track to success will be offered by recognized and certified educational institutions. Coursework should ideally cover both theoretical foundations and the practical application of skills.
Some criteria to consider when choosing the best cloud computing courses:
- Comprehensive Curriculum. Ensure that the coursework of their chosen qualification covers the skills required for career advancement. Ideally, it should include foundational cloud concepts, infrastructure management, security, practical implementation, and vendor-specific training, depending on the desired career path.
- Appropriate Teaching Methodology. Identify education providers that offer platforms including a mix of learning formats. Prioritize coursework that includes virtual instructor-led lectures, hands-on experience, real-world projects, and cloud sandboxes to allow for knowledge and application testing.
- Flexible Offerings. Many potential students will already have careers. Even those who do not hold full-time positions may be time-poor. The best cloud computing courses will make allowances for this by offering accessible and flexible options for study.
- Reputation and Accreditation. Prioritize offerings from reputable educational institutions that are acknowledged by industry leaders. The providers should be accredited by recognized regulatory bodies, offering industry leaders and organizations peace of mind when evaluating a potential employee.
Here are the top five cloud computing courses available on- and off-line to help get you started:
1. OPIT BSc in Modern Computer Science
The fully accredited OPIT BSc in Modern Computer Science provides students with the flexibility of online and fully remote coursework and study. The degree was developed to provide students with comprehensive foundation skills, both theoretical and practical. Courses include programming, software development, database management, cloud computing, cybersecurity, data science and artificial intelligence.
2. Introduction to Amazon Web Services Cloud Computing
Amazon Web Services (AWS) is the world’s leading cloud computing platform. This beginner-friendly “Introduction to Cloud Computing on AWS” qualification will provide hands-on, practical skills related to both fundamental cloud computing concepts and advanced AWS competencies.
The coursework consists of seven hours of easily accessible video and two articles. Content can be accessed online, and the successful student will receive a certificate of completion. The skills obtained during the study are perfect for those who want to progress to either a qualification as an AWS Certified Cloud Practitioner or an AWS Certified Solutions Architect.
3. Udemy – Getting Started With Cloud Computing – Level 1
This Udemy program provides a grounding in some fundamental cloud computing concepts. It includes course content such as cloud fundamentals and the five basic concepts that underpin cloud computing. The coursework also covers an introduction to service models such as SaaS, PaaS, and IaaS, and deployment models (private, public, hybrid, and community).
4. Coursera Cloud Computing Concepts (Part 1)
This flexible 23-hour course will provide foundational skills in distributed algorithms, distributed and cloud computing, and C++. Students will have access to industry experts to get a practical understanding of cloud computing and related subjects. Coursework includes hands-on projects that are relevant to individual career paths. A shareable ‘career certificate’ ideal for LinkedIn use is available to those obtaining the qualification.
5. Udemy Ultimate AWS Certified Solutions Architect Associate SAA-C03
This course is among the best cloud computing courses available. It is ideal for aspirant professionals who want to take their AWS qualifications to the next level. The coursework is flexible and allows students access to 800+ explanatory slides and a practice exam (with answer explanations). Students will have the opportunity to learn solution architecture analysis and database management, AWS fundamentals, and the implementation of real-world architecture using AWS.
The Skills You Gain From Top Cloud Computing Courses
Qualifications offered by accredited education institutions will provide both foundational and advanced competencies in cloud-related subjects. Students will obtain theoretical and practical knowledge that will advance their careers in the ever-evolving world of cloud computing.
A diverse skillset, including subjects such as database management, coding, and understanding the complexities of the various cloud solutions available is essential for those who are committed to a career in the cloud. However, these skills are not only applicable to the domain of cloud computing. Many are highly sought-after skills that are perfect for various IT and digital business roles, giving degree-holders options about their career trajectories.
Choosing the Right Course for Your Career Goals
The explosion of cloud applications and functionality provides enormous scope for a variety of career paths. These can include careers in cloud architecture, cloud application development, systems administration, front-end development, business consulting, cloud security, and data science, among many others.
The key to a successful cloud-based career is to understand the various career paths available and to ensure that the chosen path helps meet career objectives. An essential part of this journey is focusing on the best cloud computing courses.
How OPIT’s Cloud Computing Course in the BSc in Modern Computer Science Stands Out
The OPIT BSc in Modern Computer Science has become the preferred choice of those who want to fast-track their career in cloud computing. The coursework for the program provides the student with foundational, advanced, and real-world skills and leverages state-of-the-art tools and input from industry leaders and academic experts to provide actionable learning.
Flexibility and remote learning make this qualification the ideal choice for those who simply cannot commit to full-time study.
OPIT (Open Institute of Technology) is a fully licensed and accredited online higher education, a factor that can influence hiring decisions. It is recognized by the MFHEA under the European Qualification Framework (EQF) and the Malta Qualification Framework (MQF).
A Cloud Computing Qualification – Your Key to Success
The cloud is today part and parcel of the modern business environment, and it continues to evolve. This continued evolution provides incredible opportunities for the business leaders of tomorrow.
Carefully researching the best cloud computing courses is the key to success in the growing industry. However, the prospective student needs to take extra care when selecting their desired qualification and area of study. The coursework should complement the student’s chosen career path and offer the flexibility to study when and where they want.
OPIT is recognized as a premium education provider. The recognition of OPIT accreditation from leading standards authorities lends further weight to any qualification received from this respected institution. A qualification from OPIT opens the door to a stimulating and rewarding career at the cutting edge of cloud development.
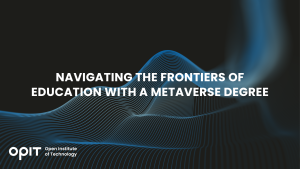
Interest in the potential of the metaverse is skyrocketing. This virtual landscape shows promising innovations in the way an average user interacts with tech. Using a blend of augmented reality (AR), virtual reality (VR), and blockchain technology, it promises to elevate user experience to new heights.
But it’s not just techies that are rejoicing. The metaverse will bring new marketing, brand-building, and sales opportunities for businesses of all sizes. And even more exciting – it promises to supercharge one of the globe’s fastest-growing entertainment sectors, gaming.
Those wanting to get in on the ground floor of the rapidly growing business and employment opportunities of the metaverse should carefully evaluate the advantages of a metaverse degree.
The metaverse is attracting some large investments from technology leaders, including Apple, Google, and Meta (previously known as Facebook). Even Microsoft has staked a claim to the immersive digital universes promised by Meta’s Metaverse.
With this much investment, it seems certain that the metaverse is here to stay.
The metaverse provides a new generation of business leaders and innovators a unique opportunity in this fast-evolving space. For these aspirant leaders, leveraging the opportunities of the metaverse will require a multidisciplinary degree. Those equipped with this specialized education will be in a prime position to enter the rapidly evolving metaverse-related employment market and adapt to the digital landscape’s changes.
Best On- and Off-Line Metaverse Degrees
Citibank estimates that the pool of metaverse users could top 5 billion by 2030 and that by then, metaverse business may be worth around $13 trillion.
The potentially explosive growth of metaverse career opportunities means that choosing the right provider of on or offline degree courses is essential. Given increasing demand, it is no surprise that some of the most respected technology-focused higher education institutions are providing some cutting-edge options for advanced study. These institutions embrace a multidisciplinary approach to the intersection of technology, business, and creativity.
Here are the five best metaverse degrees currently available:
Carnegie Mellon University – The Master of Human-Computer Interaction (MHCI) Program
This three-semester program consists of core courses such as the Capstone course – a seven-month R&D team project with an external industry client. The offering from Carnegie-Mellon claims to be the first program dedicated to preparing innovators and professionals for a career in human-computer interaction, user experience design, and user-focused research.
The University of Washington – MSc in Human-Centered Design and Engineering (HCDE)
The University of Washington MSC is a flexible course that offers a part-time or full-time degree. The program’s core courses include Theoretical Foundations of Human-Centered Design & Engineering, Navigating Design in Organizational Contexts, Visual Communication, and User-Centered Design.
University of Queensland – Master of Interaction Design
The University of Queensland’s course takes two years to complete, one of the lengthiest on this list. It incorporates core courses such as Design Thinking, Digital Prototyping, Human-Computer Interaction, and Social and Mobile Computing. Rather than focusing on the technology that will power the metaverse, this master’s degree focuses on how users interact with virtual environments. Those who qualify will become Interaction Designers, creating user-friendly and accessible digital products.
UCL (London, England) – The Human-Computer Interaction MSc
The UCL 12-month degree program is focused on sharpening their students’ real-world skills, with courses such as Interaction Design, Interaction Science, and the MSc HCI Project. This interdisciplinary MSc is centered on practical and professional skills related to the design and use of computer and mobile technology, with a concentration on interface usability.
University of Southern California (USC) – Master of Fine Arts in Interactive Media
As the lengthiest program on this list at three years, the USC degree features core courses such as Experiments in Interactivity, Design for Interactive Media Units, Survey of Interactive Media, Experiments in Interactivity, and Interactive Design and Production. The master’s program at USC prepares students for careers in the fast-growing field of interactive entertainment. It is suitable for those who do not have advanced computer capabilities and are unfamiliar with computer-based scripting. However, knowledge of computer-based authoring and production techniques will be useful.
OPIT’s Revolutionary Approach to Metaverse Education
Future-proof your qualification with an online Bachelor of Science (BSc) in Modern Computer Science from the Open Institute of Technology (OPIT). OPIT’s metaverse degree is the key to understanding and leveraging the latest developments in the field of interactive technology.
The elective choices offered by OPIT also allow students to master various metaverse-related competencies and tailor their degrees to suit their anticipated career path. Here are two examples of popular classes students are taking right now:
Specialization in Leadership and Business Development for the Metaverse
For those professionals who want to play a pivotal role in leading the metaverse revolution, this elective is a must. It examines how the metaverse is poised to disrupt existing business models through innovative digital asset management in a virtual environment. The course also examines the commercial applications of metaverse technology and how the metaverse leaders of tomorrow will use practical skills with real-world applicability to usher in a new wave of immersive opportunities.
Diving Into Project Methodology and Visual Communication
Electives such as “Project Methodology and Visual Communication” will equip the aspirant metaverse professional with the project management skills to master the virtual worlds of gaming and Meta’s Metaverse itself. Students will master topics such as agile project management, effective visual storytelling in virtual realities, and UI/UX design principles for immersive environments.
Why Choose OPIT for Your Metaverse Education?
Only those educational institutions that grasp the revolutionary nature of the metaverse can equip professionals with the skills and qualifications they require to become Masters of the Metaverse.
OPIT is fully accredited under the European Qualification Framework and the MFHEA (Malta Further and Higher Education Authority). It’s committed to providing the metaverse leadership of tomorrow with the skills they need to dominate the metaverse market.
Many institutions of higher learning offer foundational courses that will equip professionals with the skills required for a metaverse-focused career. OPIT is different because it provides students with real-world skills that can be leveraged from the day they obtain their degree. The theoretical underpinnings of these skills are delivered by recognized industry experts and innovators, providing students with insights that make a real difference in their chosen careers.
Dive Into the Metaverse With the Right Degree
The metaverse may be made of pixels, but it is real – and it’s here. The rise of the metaverse, a fast-evolving platform at the intersection of the digital and physical world, is set to entwine every aspect of our offline identity with a digital existence. But to enter this exciting field, you need the right degree.
A metaverse degree providing practical, real-world skills is required to dominate this ever-evolving digital space. An affordable, fast-track degree from OPIT will provide you with a comprehensive foundation of skills, theoretical and applicative, and marketable, to place you exactly where you need to be – at the forefront of this exhilarating frontier. Get ready to harness the potential of virtual realms with a metaverse degree from OPIT.
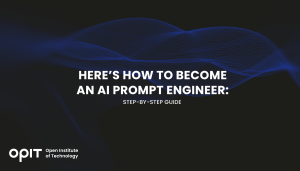
Artificial Intelligence (AI) is the talk of the town (or the globe). It is currently leading the charge in tech advancements in almost every sector, from healthcare to customer service. The advancement of AI has also brought new roles along with it, chief among them that of an AI prompt engineer—a career at the confluence of AI innovation and human creativity. This guide will show you how to join this cutting-edge field, where technical prowess meets linguistic flair and psychological insight.
Here’s how to become an AI prompt engineer.
What Is an AI Prompt Engineer?
AI prompt engineers translate and bridge the gap between human curiosity and AI’s massive knowledge base. They construct the behind-the-scenes questions or “prompts” that ask AI systems in a way that the machine’s response gives just the right result.
Imagine asking an AI about the best way to make a pizza, and instead of getting a recipe, you end up with a history lesson on tomatoes. You don’t have to be precise with your imagination because AI prompt engineers to step up, tweak, and fine-tune the prompts to lead the AI toward understanding the question.
These engineers also help interpret the AI’s responses and refine those prompts based on accuracy and relevance. They teach it how to understand not just words but the intent behind them. It’s what makes AI conversations feel more natural and less like you’re talking to a textbook.
AI prompt engineers are at the forefront of bridging the gap between human intentions and AI’s capabilities. They observe and train the AI models to grasp and respond to human languages more effectively.
Essential Skills and Qualifications
For this role, one must cultivate a blend of technical, creative, and analytical skills. The following are essential for any aspiring AI prompt engineer:
- Python. This lingua franca of AI development is necessary for any AI prompt engineer. You should have a solid grasp of this language for coding and for leveraging AI frameworks and libraries for developing and refining AI models.
- Natural Language Processing (NLP). As a merge between linguistics and computer science, it’s the heart of what makes AI systems understand and generate human language. Knowledge of NLP principles and technologies enables AI prompt engineers to make prompts that make sense to the AI.
- Creative touch. While you can’t necessarily learn the skill, it’s still fairly essential and leads to prompts that are clear to the AI and engaging or meaningful to humans. You must find novel ways to communicate with AI to achieve sought-after outcomes.
- Machine learning. You will also need a fundamental understanding of this field of study. Engineers use it to fine-tune AI models and improve their responsiveness and accuracy using feedback loops from real-world interactions.
AI prompt engineer is a very new job title, so it isn’t quite yet a distinct traditional academic path. Still, many paths can lead to this career.
- Computer science gives you a broad foundation in programming, algorithms, and data structures, technical skills necessary for AI development.
- Linguistics may not seem like a major to lead into a tech job. Still, it gives insights into the structure and function of language for understanding and improving AI’s language processing.
- Cognitive science bridges the gap between human psychology and computer science. It can show you how a machine can mimic (or fail at mimicking) human thinking.
- AI and machine learning programs, as a whole, focus directly on the technologies behind AI, which are the foundation for an AI prompt engineer.
Path to Becoming an AI Prompt Engineer
So, how to become an AI prompt engineer, then? Now that you understand what skills you need and what degrees might be the best, let’s see how to get there.
- Pursue a bachelor’s or master’s degree in fields that lay the groundwork for a career in AI, like computer science, linguistics, cognitive science, or AI and machine learning. You’ll get the theoretical basics and technical skills to get the human and computational parts of AI prompt engineering.
- Look for internships where you can work on actual AI projects. Try personal projects or contribute to open-source AI. Such projects can be related to anything you enjoy. Doing so is fun and lets you experiment and innovate with AI technologies. Moreover, others, including possible employers, will get an idea about your skills.
- Never stop learning. Take part in workshops, enroll in online courses, and get as many certificates in AI, NLP, and machine learning as you can.
- Take part in the AI community through forums, social media groups, and conferences. When you’re a part of a group effort, you get to learn and grow along with the community and get your name out.
- Take time to reflect on your learning and projects. Be open to exploring new areas of AI that interest you, and don’t be afraid to change your focus as you discover what excites you the most about AI prompt engineering and what might miss the mark for you.
OPIT’s Programs in AI and Machine Learning
OPIT’s educational program lineup offers several pathways to becoming an AI prompt engineer—the MSc in Responsible Artificial Intelligence, the BSc in Modern Computer Science, and the MSc in Data Science & AI. These degrees give you all the skills you need to tackle AI prompt battles and victories.
The heavy-duty content covers everything from the basics to the brain-bending advanced topics. Once you know the theory, you will also get the practice of project-based learning that takes you out of the classroom (figuratively, since you might still physically be in one). Hands-on learning segments plunge you into real-world AI development.
By the time you’re done, you will be theoretically proficient and have experience in applying AI in various scenarios, including the nuanced art of prompt engineering. For example, you might have to refine an AI’s ability to understand and generate human-like. Or, you might develop prompts that take an AI through complex ethical dilemmas.
Why Choose a Career as an AI Prompt Engineer
Being an AI prompt engineer takes you straight to the front lines of AI development, where every day brings a new challenge and a chance to shape the future of how humans and machines interact. It’s a career path with immense potential for growth, innovation, and creativity. This career is ideal for tech-inclined people who want to be pioneers, a part of the bleeding-edge technology before it becomes a necessary part of everyone’s workflow.
Be at the AI Frontlines
Now you know how to become an AI prompt engineer, so it’s time to get started on this exciting career path. Focus on relevant degree programs like computer science, linguistics, or AI, and keep an eye out for opportunities for more hands-on learning – whether it’s an internship or an open source project.
While you’re mapping out your career path, let OPIT be part of the journey with programs that will set you up for success in this field. Whether it’s a bachelor’s or master’s degree, you’ll receive a comprehensive education with relevant hands-on experience from experts in the field, poised to position any aspiring AI prompt engineer for success.
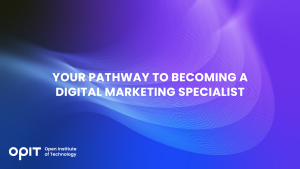
If you’re eyeing the digital marketing scene, know that every click tells a story, and every strategy can turn the tables for companies fighting for the online spotlight. Creativity meets metrics, and social media is a place to make connections that matter and spread the word about your brand or make it so that the word spreads on its own. Here is how to become a digital marketing specialist.
Understanding the Role of a Digital Marketing Specialist
Imagine being the person for everything online in a business. That’s more or less what a digital marketing specialist does.
They handle everything from making a website appear at the top of search results (thanks to SEO) to crafting the kind of content that makes people click, share, and buy. Specialists know what makes audiences tick on social media and keep up with the latest hashtag trends to figure out the best time to post. They don’t throw content out into the void and hope for the best—they analyze everything.
Which tweet has the most engagement? Did changing the call-to-action on that email campaign increase conversions? It’s all in a day’s work.
But what’s the big deal about all this, you might ask.
Being visible online is pretty much everything for a business these days. It matters little if it’s a local bakery or a multinational tech company. They all need to be online so potential customers can find them. Their digital marketing specialist uses a mix of creativity, analytics, and tech-savvy to boost brand awareness, rake in leads, and ultimately, drive sales. These specialists are the ones making sure a business is actively connecting with people and turning them into customers.
Essential Skills and Qualifications
To become a digital marketing specialist, you must have strong technical skills, creativity, and a fair bit of analytical prowess. Here’s a rundown:
- SEO/SEM reveals the alchemy of search engines that can make or break a brand’s online visibility. This skill helps you know the right keywords, the magic of backlinks, and the techniques of ranking higher.
- Content creation (blogs that tell a story, videos that captivate, or tweets that go viral) must resonate with your audience.
- Data analysis is great if you love numbers because dissecting website traffic, engagement rates, and conversion stats to understand what’s working (and what’s not) is a big part of the game.
- Social media expertise means knowing your TikToks from your Tweets. Each platform operates on a distinct system, and mastering these can help you connect with audiences effectively.
- PPC advertising is just as significant. Crafting the ads at the top of the search results requires a keen understanding of PPC strategies, especially if you’re aiming for a monetary balance.
As for the educational backdrop, while you don’t necessarily need a degree in digital marketing, having one in related fields like marketing, communications, or business can give you a solid foundation. That said, digital marketing values skills and real-world experience just as much, if not more.
Path to Becoming a Digital Marketing Specialist
Here’s a guide on how to be a digital marketing specialist:
1. Obtain a Relevant Degree
While you don’t need a degree in marketing to break into digital marketing, it undoubtedly helps. A bachelor’s in marketing, communications, or a related field lays a solid foundation. It introduces you to marketing principles, consumer behavior, and, increasingly, digital marketing basics. If college isn’t your path, there are still many success stories from self-taught specialists.
2. Get Hands-on Experience
There’s no substitute for getting directly involved in digital marketing. Internships are a great step, as they give you a peek into the industry’s workings and let you apply what you’ve learned. Or you can start your own project. Create a blog, manage social media for a family business, or run your own PPC campaigns to build a portfolio that showcases your skills.
3. Prioritize Continuous Learning
Digital marketing evolves fast, so you must keep up with the trends. Online courses and certifications can keep you up to date while also boosting your resume. Certifications like Google Ads, Facebook Blueprint, or SEMrush certifications also prove your skills and dedication to the craft. Each one is a step toward establishing your expertise.
4. Start Networking
Join digital marketing forums, attend webinars, and don’t be shy about reaching out to professionals you admire. The digital marketing community is surprisingly welcoming and a treasure trove of insights and opportunities.
5. Apply for Entry-Level Positions
With some education, a portfolio, and a few certifications under your belt, start applying for entry-level positions. Adjust your resume to highlight your digital marketing skills and projects, and don’t get discouraged by setbacks. Every interview, whether successful or not, is a point of learning.
OPIT’s Digital Marketing Education Programs
OPIT has a couple of solid programs up its sleeve for digital marketing—the BSc and MSc in Digital Business. They aren’t typical, dry lecture-based courses. These programs give you both the theory and practice necessary in digital marketing.
OPIT’s approach is unique in the way it blends theory with practice. You learn the latest trends, tools, and strategies currently being used in the industry. Plus, you’re not learning in a vacuum. OPIT connects you with industry experts, giving you a chance to pick the brains of people who have invaluable experience and skills. The programs offer first-hand insights, resources, and a network of professionals you’d be hard-pressed to find anywhere else.
Why Choose a Career as a Digital Marketing Specialist
For starters, every business out there, from the hole-in-the-wall coffee shop to giant corporations, is trying to make its mark online. That means there’s an enormous demand for people who can handle the digital world creatively and effectively.
This job also keeps you on your toes. One day, you might be cracking the code on a Google Ads campaign, and the next, you’re storytelling on Instagram or analyzing website traffic. It’s this mix of creativity, strategy, and analytics that makes the work diverse and, believe it or not, pretty exciting.
Become the Social Media Strategist You Were Meant to Be
The path to becoming a specialist is fairly varied, but two factors hold true: you need to keep on top of current trends, and you need hands-on experience. Fortunately, OPIT positions you on the right career path by providing just that. Check out OPIT’s bachelor’s and master’s programs in digital business and learn how to be a good digital marketing specialist first-hand.
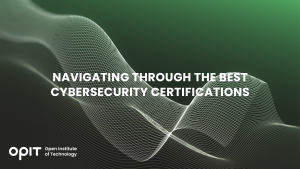
The world is becoming a more dangerous place, especially where company data and system integrity are concerned. Globally, data breaches cost organizations an average of $4.45 million in 2023, and business owners should not expect good news when the 2024 figures are made available.
The increasing threats of ransomware and phishing, two of the most prevalent threats, have motivated many businesses to increase their focus on cybersecurity. The result is increased demand for cybersecurity professionals. For those thinking about a career in the ever-evolving field of cybersecurity, learning more about the best cybersecurity certifications is essential.
Understanding the Cybersecurity Certification Landscape
As the sophistication of cyberattacks grows, so does the demand for IT professionals with certifications or degrees. Prospective employers need to know that the staff they onboard will add value to the organization. One of the ways these employers can enjoy peace of mind is by knowing that their employees are up to date with the latest developments in the cybersecurity field.
Put simply, a certificate validates the skills and knowledge of the holder. That can make all the difference when it comes to professional development. However, that certification must be provided by a recognized and accredited education provider. Finding the best cybersecurity certification can be a daunting task due to the wealth of options. Making the right decision is essential.
Identifying the Best Certifications for Cybersecurity
CyberSeek research has revealed that only 72 cybersecurity professionals are available for every 100 cybersecurity jobs (from September 2022 to August 2023). That means that demand for certified professionals is at an all-time high.
Given the opportunities in the cybersecurity field, it is no surprise that the field of certification is extremely competitive. However, as with most educational offerings, there are good certifications, and there are great certifications.
What factors should the prospective student take into account when making their choice?
- Industry recognition. A great supplier of cybersecurity certifications will usually showcase the companies that recognize their certifications as world-class. It’s a sure sign that they can deliver exceptional career opportunities.
- Real-world experience. The best cybersecurity certifications will include coursework that combines the theoretical with the practical. Look for certifications that have components offering students experience with real-world challenges.
- Accreditation. Ensure that the cybersecurity certification provider is accredited by a leading qualifications body. An example is accreditation as an Online Higher Education institution by regulatory bodies like the MFHEA (under the European Qualification Framework).
- Flexibility. It is more and more common for certification courses to be offered over the internet. These courses allow the student to study when and where they want.
- Scope of offerings. The cybersecurity field is complex, so make sure that the cybersecurity certification you choose has coursework that applies to your vision for professional growth.
Whether you opt for a consulting role, a specialization, or see yourself as a future board member choosing the best cybersecurity certification is vital.
Exploring the Best Cybersecurity Certificate Programs
Choose your program carefully. Although most cybersecurity jobs require at least a bachelor’s degree, many hiring managers will focus on candidates who add value through top cybersecurity certifications that complement their existing qualifications.
1. Google Cybersecurity Professional Certificate
For those starting on their cybersecurity journey, the cybersecurity certification from Google is an attractive option. The certification focuses on on-demand skills, and you can complete your studies in around six months. During the flexible course, students will learn about the importance of cybersecurity practices and how those practices impact organizations.
This foundational certification is ideal for those seeking a career as a cybersecurity analyst, security operations center (SOC) analyst, or security analyst.
2. CompTIA Security+
This certification is ideal for students looking to build foundational skills for almost any cybersecurity role. It provides companies with a validation that the certificate holder is able to monitor and secure mobile and cloud applications and the Internet of Things (IoT) environments. The best cybersecurity certificates also ensure students understand the laws and regulations related to risk and compliance.
This certification can be the foundation of a career as a systems administrator, security engineer, IT auditor, or cloud engineer (among others).
3. Certified Information Systems Security Professional (CISSP)
For professionals who want to take their cybersecurity skills and careers to new heights, the CISSP certification ticks all the right boxes. It underlines the applicant’s experience in IT security and their skills in developing, implementing, and monitoring a state-of-the-art cybersecurity program.
Students will require five years of cumulative work experience and a four-year degree in computer science – part-time work or paid internships count towards work experience.
This certification will open up opportunities as a chief information security officer, a security administrator, or a top-flight security consultant.
There are numerous programs for motivated professionals interested in either launching or furthering a career in cybersecurity, but a recognized certification from an accredited and trusted education provider is the key to success.
OPIT’s MSc in Enterprise Cybersecurity: A Unique Opportunity
OPIT’s postgraduate MSc in Enterprise Cybersecurity will take between a year and 18 months to complete. Courses are delivered online, allowing students to learn when and where they prefer.
This certification is designed to align with the four most important foundational industry certifications: CISSP, CISM, CRISC, and CEH.
The program provides employers with a certified professional skilled in both technical and managerial roles, fulfilling an urgent need for multidisciplinary cybersecurity resources. The coursework combines core theoretical concepts, real-world application, and soft skills, dovetailing neatly with the strategic needs of the modern organization.
The Master’s from OPIT is the best cybersecurity certification for IT professionals interested in a career as:
- Chief Information Security Officer (CISO)
- Security Solutions Architect
- Cybersecurity Manager/Director
- Cybersecurity Risk Analyst
- Cybersecurity Compliance Officer
- Incident Response Manager
- Cloud Security Manager
The Advantage of a Comprehensive Education With OPIT
The business world, and especially the world of cybersecurity, is complex and fast-evolving. Businesses require employees with an education aligned with the needs of the industry to cope with the ever-accelerating rate of change.
The best cybersecurity certifications, such as the MSc in Enterprise Cybersecurity from OPIT, provide a mix of technical and management skills aligned with industry demands and standards for a holistic educational approach. This allows graduates to deepen their understanding of the challenges of cybersecurity issues and how they impact business strategy and day-to-day operations.
Elevate Your Career With OPIT
The cybersecurity environment is complex. Employers want to know that their IT professionals can deal with complexity. This makes getting the best cybersecurity certification essential.
The reputation and accreditation of the certificate provider are essential, but keep your chosen career path in mind. Make sure that the certificate coursework combines theory and practical cybersecurity experience. The OPIT MSc in Enterprise Cybersecurity provides professionals with a unique career-focused advantage by integrating certification preparation with accessible, specialized education.
The result is a well-rounded, expert employee who is intimately familiar with the evolving challenges of cybersecurity, and that is the secret of success.
Have questions?
Visit our FAQ page or get in touch with us!
Write us at +39 335 576 0263
Get in touch at hello@opit.com
Talk to one of our Study Advisors
We are international
We can speak in: