Data Science & AI
Dive deep into data-driven technologies: Machine Learning, Reinforcement Learning, Data Mining, Big Data, NLP & more. Stay updated.
Search inside The Magazine
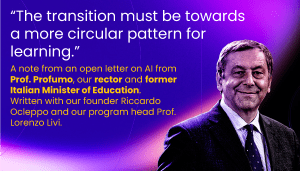
AI, and its integration with society, had an incredible acceleration in recent months. By now, it seems certain that AI will be the fourth GPT (General Purpose Technology) of human history: one of those few technologies or inventions that radically and indelibly change society. The last of these technologies was ICT (internet, semiconductor industry, telecommunications); before this, electricity and the steam engine were the first 2 GPTs.
All three GPTs had a huge impact on the overall productivity and advancement of our society with, of course, a profound impact on the world of work. Such an impact, though, was very different across these technologies. The advent of electricity and the steam motor allowed the displacement of large masses of workers from more archaic and manual jobs to their equivalent jobs in the new industrial era, where not many skills were required. The advent of ICT, on the other hand, has generated enormous job opportunities, but also the need to develop meaningful skills to pursue them.
As a result, an increasingly large share of the economic benefit deriving from the advent of ICT has gradually been polarized towards people who had (and have) these skills in society. Suffice it to say that, already in 2017, the richest 1% of America owned twice the wealth of the “poorest” 90%.
It is difficult to make predictions about how the advent of AI will impact this trend already underway. But there are some very clear elements: one of these is that quality education in technology (and not only) will increasingly play a primary role in being able to secure the best career opportunities for a successful future in this new era.
To play a “lead actor” role in this change, though, the world of education – and in particular that of undergraduate and postgraduate education – requires a huge change towards being much more flexible, aligned to today’s needs of students and companies, and affordable.
Let’s take a step back: we grew up thinking that “learning” meant following a set path. Enroll in elementary school, attend middle and high school, and, for the luckiest or most ambitious, conclude by taking a degree.
This model needs to be seriously challenged and adapted to the times: solid foundational learning remains an essential prerogative. But in a “fast” world in rapid change like today’s, knowledge acquired along this “linear” path will not be able to accompany people in their professions until the end of their careers. The “utility period” of the knowledge we acquire today reduces every day, and this emphasizes how essential continuous learning is throughout our lives.
The transition must therefore be towards a more circular pattern for learning. A model in which one returns “to the school desk” several times in life, in order to update oneself, and forget “obsolete” knowledge, making room for new production models, new ways of thinking, organizing, and new technologies.
In this context, Education providers must rethink the way they operate and how they intend to address this need for lifelong learning.
Higher Education Institutions, as accredited bodies and guarantors of the quality of education (OPIT – Open Institute of Technology among these), have the honor of playing a primary role in this transition.
But also the great burden of rethinking their model from scratch which, in a digital age, cannot be a pure and simple digital transposition of the old analog learning model.
The Institutions Universities are called upon to review and keep updated their own study programmes, think of new, more flexible and faster ways of offering them to a wider public, forge greater connections with companies, and ultimately provide them with students who are immediately ready to successfully enter the dynamics of production. And, of course, be more affordable and accessible: quality education in the AI era cannot cost tens of thousands of dollars, and needs to be accessed from wherever the students are.
With OPIT – Open Institute of Technology, this is the path we have taken, taking advantage of the great privilege of being able to start a new path, without preconceptions or “attachment” to the past. We envision a model of a new, digital-first, higher education institution capable of addressing all the points above, and accompany students and professionals throughout their lifetime learning journey.
We are at the beginning, and we hope that the modern and fresh approach we are following can be an interesting starting point for other universities as well.
Authors
Prof. Francesco Profumo, Rector of OPIT – Open Institute of Technology
Former Minister of Education, University and Research of Italy, Academician and author, former President of the National Research Council of Italy, and former Rector of Politecnico di Torino. He is an honorary member of various scientific associations.
Riccardo Ocleppo, Managing Director of OPIT
Founder of OPIT, Founder of Docsity.com, one of the biggest online communities for students with 19+ registered users. MSc in Management at London Business School, MSc in Electronics Engineering at Politecnico di Torino
Prof. Lorenzo Livi, Programme Head at OPIT
Former Associate Professor of Machine Learning at the University of Manitoba, Honorary Senior Lecturer at the University of Exeter, Ph.D. in Computer Science at Università La Sapienza.
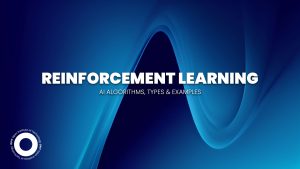
Reinforcement learning is a very useful (and currently popular) subtype of machine learning and artificial intelligence. It is based on the principle that agents, when placed in an interactive environment, can learn from their actions via rewards associated with the actions, and improve the time to achieve their goal.
In this article, we’ll explore the fundamental concepts of reinforcement learning and discuss its key components, types, and applications.
Definition of Reinforcement Learning
We can define reinforcement learning as a machine learning technique involving an agent who needs to decide which actions it needs to do to perform a task that has been assigned to it most effectively. For this, rewards are assigned to the different actions that the agent can take at different situations or states of the environment. Initially, the agent has no idea about the best or correct actions. Using reinforcement learning, it explores its action choices via trial and error and figures out the best set of actions for completing its assigned task.
The basic idea behind a reinforcement learning agent is to learn from experience. Just like humans learn lessons from their past successes and mistakes, reinforcement learning agents do the same – when they do something “good” they get a reward, but, if they do something “bad”, they get penalized. The reward reinforces the good actions while the penalty avoids the bad ones.
Reinforcement learning requires several key components:
- Agent – This is the “who” or the subject of the process, which performs different actions to perform a task that has been assigned to it.
- Environment – This is the “where” or a situation in which the agent is placed.
- Actions – This is the “what” or the steps an agent needs to take to reach the goal.
- Rewards – This is the feedback an agent receives after performing an action.
Before we dig deep into the technicalities, let’s warm up with a real-life example. Reinforcement isn’t new, and we’ve used it for different purposes for centuries. One of the most basic examples is dog training.
Let’s say you’re in a park, trying to teach your dog to fetch a ball. In this case, the dog is the agent, and the park is the environment. Once you throw the ball, the dog will run to catch it, and that’s the action part. When he brings the ball back to you and releases it, he’ll get a reward (a treat). Since he got a reward, the dog will understand that his actions were appropriate and will repeat them in the future. If the dog doesn’t bring the ball back, he may get some “punishment” – you may ignore him or say “No!” After a few attempts (or more than a few, depending on how stubborn your dog is), the dog will fetch the ball with ease.
We can say that the reinforcement learning process has three steps:
- Interaction
- Learning
- Decision-making
Types of Reinforcement Learning
There are two types of reinforcement learning: model-based and model-free.
Model-Based Reinforcement Learning
With model-based reinforcement learning (RL), there’s a model that an agent uses to create additional experiences. Think of this model as a mental image that the agent can analyze to assess whether particular strategies could work.
Some of the advantages of this RL type are:
- It doesn’t need a lot of samples.
- It can save time.
- It offers a safe environment for testing and exploration.
The potential drawbacks are:
- Its performance relies on the model. If the model isn’t good, the performance won’t be good either.
- It’s quite complex.
Model-Free Reinforcement Learning
In this case, an agent doesn’t rely on a model. Instead, the basis for its actions lies in direct interactions with the environment. An agent tries different scenarios and tests whether they’re successful. If yes, the agent will keep repeating them. If not, it will try another scenario until it finds the right one.
What are the advantages of model-free reinforcement learning?
- It doesn’t depend on a model’s accuracy.
- It’s not as computationally complex as model-based RL.
- It’s often better for real-life situations.
Some of the drawbacks are:
- It requires more exploration, so it can be more time-consuming.
- It can be dangerous because it relies on real-life interactions.
Model-Based vs. Model-Free Reinforcement Learning: Example
Understanding model-based and model-free RL can be challenging because they often seem too complex and abstract. We’ll try to make the concepts easier to understand through a real-life example.
Let’s say you have two soccer teams that have never played each other before. Therefore, neither of the teams knows what to expect. At the beginning of the match, Team A tries different strategies to see whether they can score a goal. When they find a strategy that works, they’ll keep using it to score more goals. This is model-free reinforcement learning.
On the other hand, Team B came prepared. They spent hours investigating strategies and examining the opponent. The players came up with tactics based on their interpretation of how Team A will play. This is model-based reinforcement learning.
Who will be more successful? There’s no way to tell. Team B may be more successful in the beginning because they have previous knowledge. But Team A can catch up quickly, especially if they use the right tactics from the start.
Reinforcement Learning Algorithms
A reinforcement learning algorithm specifies how an agent learns suitable actions from the rewards. RL algorithms are divided into two categories: value-based and policy gradient-based.
Value-Based Algorithms
Value-based algorithms learn the value at each state of the environment, where the value of a state is given by the expected rewards to complete the task while starting from that state.
Q-Learning
This model-free, off-policy RL algorithm focuses on providing guidelines to the agent on what actions to take and under what circumstances to win the reward. The algorithm uses Q-tables in which it calculates the potential rewards for different state-action pairs in the environment. The table contains Q-values that get updated after each action during the agent’s training. During execution, the agent goes back to this table to see which actions have the best value.
Deep Q-Networks (DQN)
Deep Q-networks, or deep q-learning, operate similarly to q-learning. The main difference is that the algorithm in this case is based on neural networks.
SARSA
The acronym stands for state-action-reward-state-action. SARSA is an on-policy RL algorithm that uses the current action from the current policy to learn the value.
Policy-Based Algorithms
These algorithms directly update the policy to maximize the reward. There are different policy gradient-based algorithms: REINFORCE, proximal policy optimization, trust region policy optimization, actor-critic algorithms, advantage actor-critic, deep deterministic policy gradient (DDPG), and twin-delayed DDPG.
Examples of Reinforcement Learning Applications
The advantages of reinforcement learning have been recognized in many spheres. Here are several concrete applications of RL.
Robotics and Automation
With RL, robotic arms can be trained to perform human-like tasks. Robotic arms can give you a hand in warehouse management, packaging, quality testing, defect inspection, and many other aspects.
Another notable role of RL lies in automation, and self-driving cars are an excellent example. They’re introduced to different situations through which they learn how to behave in specific circumstances and offer better performance.
Gaming and Entertainment
Gaming and entertainment industries certainly benefit from RL in many ways. From AlphaGo (the first program that has beaten a human in the board game Go) to video games AI, RL offers limitless possibilities.
Finance and Trading
RL can optimize and improve trading strategies, help with portfolio management, minimize risks that come with running a business, and maximize profit.
Healthcare and Medicine
RL can help healthcare workers customize the best treatment plan for their patients, focusing on personalization. It can also play a major role in drug discovery and testing, allowing the entire sector to get one step closer to curing patients quickly and efficiently.
Basics for Implementing Reinforcement Learning
The success of reinforcement learning in a specific area depends on many factors.
First, you need to analyze a specific situation and see which RL algorithm suits it. Your job doesn’t end there; now you need to define the environment and the agent and figure out the right reward system. Without them, RL doesn’t exist. Next, allow the agent to put its detective cap on and explore new features, but ensure it uses the existing knowledge adequately (strike the right balance between exploration and exploitation). Since RL changes rapidly, you want to keep your model updated. Examine it every now and then to see what you can tweak to keep your model in top shape.
Explore the World of Possibilities With Reinforcement Learning
Reinforcement learning goes hand-in-hand with the development and modernization of many industries. We’ve been witnesses to the incredible things RL can achieve when used correctly, and the future looks even better. Hop in on the RL train and immerse yourself in this fascinating world.
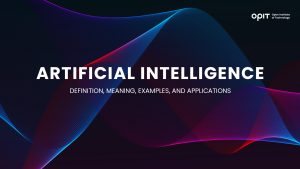
The artificial intelligence market was estimated to be worth $136 billion in 2022, with projections of up to $1,800 billion by the end of the decade. More than a third of companies today implement AI in their business processes, and over 40% will consider doing so in the future.
These whopping numbers testify to the importance, prevalence, and reality of AI in the modern world. If you’re considering an education in AI, you’re looking at a highly rewarding and prosperous future career. But what are the applications of artificial intelligence, and how did it all begin? Let’s start from scratch.
What Is Artificial Intelligence?
Artificial intelligence definition describes AI as a part of computer science that focuses on building programs and software with human intelligence. There are four types of artificial intelligence: the theory of mind, reactive, self-aware, and limited memory.
Reactive AI masters one field, like playing chess, performing a single manufacturing task, and similar. Limited memory machines can gather and remember information and use findings to offer recommendations (hotels, restaurants, etc.).
Theory of mind is a more developed type of AI capable of understanding human emotions. These machines can also take part in social interactions. Finally, self-aware AI is a conscious machine, but its development is reserved for the future.
History of Artificial Intelligence
The concept of artificial intelligence has roots in the 1950s. This was when AI became an academic discipline, and scientists started publishing papers about it. It all started with Alan Turing and his paper about computer machinery and intelligence that introduced basic AI concepts.
Here are some important milestones in the artificial intelligence field:
- 1952 – Arthur Samuel created a program that taught itself to play checkers.
- 1955 – John McCarthy’s workshop on AI, where the term was used for the first time.
- 1961 – First robot worker on a General Motors factory’s assembly line.
- 1980 – First conference on AI.
- 1986 – Demonstration of the first driverless car.
- 1997 – A program beat Gary Kasparov in a legendary chess match, thus becoming the first AI tool to win in a competition over a human.
- 2000 – Development of a robot that simulates a person’s body movement and human emotions.
AI in the 21st Century
The 21st century has witnessed some of the fastest advancements and applications of artificial intelligence across industries. Robots are becoming more sophisticated, they land on other planets, work in shops, clean, and much more. Global corporations like Facebook, Twitter, Netflix, and others regularly use AI tools in marketing to boost user experience, etc.
We’re also seeing the rise of AI chatbots like ChatGPT that can create content indistinguishable from human content.
Fields Used in Artificial Intelligence
Artificial intelligence relies on the use of numerous technologies:
- Machine Learning – Making apps and processes that can perform tasks like humans.
- Natural Language Processing – Training computers to understand words like humans.
- Computer Vision – Developing tools and programs that can read visual data and take information from it.
- Robotics – Programming agents to perform tasks in the physical world.
Applications of Artificial Intelligence
Below is an overview of applications of artificial intelligence across industries.
Automation
Any business and sector that relies on automation can use AI tools for faster data processing. By implementing advanced artificial intelligence tools into daily processes, you can save time and resources.
Healthcare
Fraud is common in healthcare. AI in this field is mostly oriented toward lowering the risk of fraud and administrative fees. For example, using AI makes it possible to check insurance claims and find inconsistencies.
Similarly, AI can help advance and finetune medical research, telemedicine, medical training, patient engagement, and support. There’s virtually no aspect of healthcare and medicine that couldn’t benefit from AI.
Business
Businesses across industries benefit from AI to finetune various aspects like the hiring process, threat detection, analytics, task automation, and more. Business owners and managers can make better-informed business decisions with less risk of error.
Education
Modern-day education offers personalized programs tailored to the individual learner’s abilities and goals. By automating tasks with AI tools, teachers can spend more time helping students progress faster in their studies.
Security
Security has never been more important following the rise of web applications, online shopping, and data sharing. With so much sensitive information shared daily, AI can help increase data protection and mitigate hacking attacks and threats. Systems with AI features can diagnose, scan, and detect threats.
Benefits and Challenges of Artificial Intelligence
There are enormous benefits of AI applications that can revolutionize any industry. Here are just some of them:
Automation and Increased Efficiency
AI helps streamline repetitive tasks, automate processes, and boost work efficiency. This characteristic of AI is already visible in all industries, and the use of programming languages like R and Python makes it all possible.
Improved Decision Making
Stakeholders can use AI to analyze immense amounts of data (with millions or billions of pieces of information) and make better-informed business decisions. Compare this to limited data analysis of the past, where researchers only had access to local documents or libraries, and you can understand how AI empowers present-day business owners.
Cost Savings
By automating tasks and streamlining processes, businesses also spend less money. Savings in terms of energy, extra work hour costs, materials, and even HR are significant. When you use AI right, you can turn almost any project into reality with minimal cost.
Challenges of AI
Despite the numerous benefits, AI also comes with a few challenges:
Data Privacy and Security
All AI developments take place online. The web still lacks proper laws on data protection and privacy, and it’s highly possible that user data is being used without consent in AI projects worldwide. Until strict laws are enacted, AI will continue to pose a threat to data privacy.
Algorithmic Bias
Algorithms today assist humans in decision-making. Stakeholders and regular users rely on data provided by AI tools to complete or approach tasks and even form new beliefs and behaviors. Poorly trained machines can encourage human biases, which can be especially harmful.
Job Less
AI is developing at the speed of light. Many tools are already replacing human labor in both the physical and digital worlds. A question remains to what degree machines will overtake the labor market in the future.
Artificial Intelligence Examples
Let’s look at real-world examples of artificial intelligence across applications and industries.
Virtual Assistants
Apple was the first company to introduce a virtual assistant based on AI. We know the tool today by the name of Siri. Numerous other companies like Amazon and Google have followed suit, so now we have Alexa, Google Assistant, and many other AI talking assistants.
Recommendation Systems
Users today find it ever more challenging to resist addictive content online. We’re often glued to our phones because our Instagram feed keeps suggesting must-watch Reels. The same goes for Netflix and its binge-worthy shows. These platforms use AI to enhance their recommendation system and offer ads, TV shows, or videos you love.
Shopping on Amazon works in a similar fashion. Even Spotify uses AI to offer audio recommendations to customers. It relies on your previous search history, liked content, and similar data to provide new suggestions.
Autonomous Vehicles
New-age vehicles powered by AI have sophisticated systems that make commuting easier than ever. Tesla’s latest AI software can collect information in real-time from the multiple cameras on the vehicles. The AI makes a 3D map with roads, obstacles, traffic lights, and other elements to make your ride safer.
Waymo has a similar system of lidar sensors around the vehicles that send pulsations around the car and offer an overview of the car’s surroundings.
Fraud Detection
Banks and credit card companies implement AI algorithms to prevent fraud. Advanced software helps these companies understand their customers and prevent non-authorized users from making payments or completing other unauthorized actions.
Image and Voice Recognition
If you have a newer smartphone, you’re already familiar with Face ID and voice assistant tools. These are built on basic AI principles and are being integrated into broader systems like vehicles, vending machines, home appliances, and more.
Deep Learning
Artificial intelligence encompasses both deep learning and machine learning. Machine learning encompasses deep learning and uses algorithms that learn from data, explore patterns, and predict outputs.
Deep learning relies on sophisticated neural networks similar to the networks in the human brain. Deep learning specialists use these neural networks to pinpoint patterns in large data sets.
Artificial Intelligence Continues to Grow and Develop
Although predicting the future is impossible, numerous AI specialists expect to see further development in this computer science discipline. More businesses will start implementing AI and we’ll see more autonomous vehicles and smarter robotics. That said, it’s increasingly important to take into account ethical considerations. As long as we use AI ethically, there’s no danger to our social interactions and privacy.
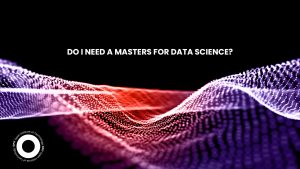
The future looks bright for the data science sector, with the U.S. Bureau of Labor Statistics stating that there were 113,300 jobs in the industry in 2021. Growth is also a major plus. The same resource estimates a 36% increase in data scientist roles between 2021 and 2031, which outpaces the national average considerably. Combine that with attractive salaries (Indeed says the average salary for a data scientist is $130,556) and you have an industry that’s ready and waiting for new talent.
That’s where you come in, as you’re exploring the possibilities in data science and need to find the appropriate educational tools to help you enter the field. A Master’s degree may be a good choice, leading to the obvious question – do you need a Master’s for data science?
The Value of a Masters in Data Science
There’s plenty of value to committing the time (and money) to earning your data science Master’s degree:
- In-depth knowledge and skills – A Master’s degree is a structured course that puts you in front of some of the leading minds in the field. You’ll develop very specific skills (most applying to the working world) and can access huge wellsprings of knowledge in the forms of your professors and their resources.
- Networking opportunities – Access to professors (and similar professionals) enables you to build connections with people who can give you a leg up when you enter the working world. You’ll also work with other students, with your peers offering as much potential for startup ideas and new roles as your professors.
- Increased job opportunities – With salaries in the $130,000 range, there’s clearly plenty of potential for a comfortable career pursuing a subject that you love. Having a Master’s degree in data science on your resume demonstrates that you’ve reached a certain skill threshold for employers, making them more likely to hire you.
Having said all of that, the answer to “do I need a Master’s for data science?” is “not necessarily.” There are actually some downsides to going down the formal studying route:
- The time commitment – Data science programs vary in length, though you can expect to commit at least 12 months of your life to your studies. Most courses require about two years of full-time study, which is a substantial time commitment given that you’ve already earned a degree and have job opportunities waiting.
- Your financial investment – A Master’s in data science can cost anywhere between about $10,000 for an online course to over $50,000 for courses from more prestigious institutions. For instance, Tufts University’s course requires a total investment of $54,304 if you wish to complete all of your credit hours.
- Opportunity cost – When opportunity beckons, committing two more years to your studies may lead to you missing out. Say a friend has a great idea for a startup, or you’re offered a role at a prestigious company after completing your undergraduate studies. Saying “no” to those opportunities may come back to bite you if they’re not waiting for you when you complete your Master’s degree.
Alternatives to a Masters in Data Science
If spending time and money on earning a Master’s degree isn’t to your liking, there are some alternative ways to develop data science skills.
Self-Learning and Online Resources
With the web offering a world of information at your fingertips, self-learning is a viable option (assuming you get something to show for it). Options include the following:
- Online courses and tutorials – The ability to learn at your own pace, rather than being tied into a multi-year degree, is the key benefit of online courses and tutorials. Some prestigious universities (including MIT and Harvard) even offer more bite-sized ways to get into data science. Reputation (both for the course and its providers) can be a problem, though, as some employers prefer candidates with more formal educations.
- Books and articles – The seemingly old-school method of book learning can take you far when it comes to learning about the ins and outs of data science. While published books help with theory, articles can keep you abreast of the latest developments in the field. Unfortunately, listing a bunch of books and articles that you’ve read on a resume isn’t the same as having a formal qualification.
- Data science competitions – Several organizations (such as Kaggle) offer data science competitions designed to test your skills. In addition to giving you the opportunity to wield your growing skillset, these competitions come with the dual benefits of prestige and prizes.
Bootcamps and Certificate Programs
Like the previously mentioned competitions, bootcamps offer intensive tests of your data science skills, with the added bonus of a job waiting for you at the end (in some cases). Think of them like cramming for an exam – you do a lot in a short time (often a few months) to get a reward at the end.
The prospect of landing a job after completing a bootcamp is great, but the study methods aren’t for everybody. If you thrive in a slower-paced environment, particularly one that allows you to expand your skillset gradually, an intensive bootcamp may be intimidating and counter to your educational needs.
Gaining Experience Through Internships and Entry-Level Positions
Any recent graduate who’s seen a job listing that asks for a degree and several years of experience can tell you how much employers value hands-on experience. That’s as true in data science as it is in any other field, which is where internships come in. An internship is an unpaid position (often with a prestigious company) that’s ideal for learning the workplace ropes and forming connections with people who can help you advance your career.
If an internship sounds right for you, consider these tips that may make them easier to find:
- Check the job posting platforms – The likes of Indeed and LinkedIn are great places to find companies (and the people within them) who may offer internships. There are also intern-dedicated websites, such as internships.com, which focus specifically on this type of employment.
- Meet the basic requirements – Most internships don’t require you to have formal qualifications, such as a Master’s degree, to apply. But by the same token, companies won’t accept you for a data science internship if you have no experience with computers. A solid understanding of major programming and scripting languages, such as Java, SQL, and C++, gives you a major head start. You’ve also got a better chance of landing a role if you enrolled in an undergraduate program (or have completed one) in computer science, math, or a similar field.
- Check individual business websites – Not all companies run to LinkedIn or job posting sites when they advertise vacant positions. Some put those roles on their own websites, meaning a little more in-depth searching can pay off. Create a list of companies that you believe you’d enjoy working for and check their business websites to see if they’re offering internships via their sites.
Factors to Consider When Deciding if a Masters Is Necessary
You know that the answer to “Do you need a Master’s for data science?” is “no,” but there are downsides to the alternatives. Being able to prove your skills on a resume is a must, which the self-learning route doesn’t always provide, and some alternatives may be too fast-paced for those who want to take their time getting to grips with the subject. When making your choice, the following four factors should play into your decision-making
Personal Goals and Career Aspirations
The opportunity cost factor often comes into play here, as you may find that some entry-level roles for computer science graduates can “teach you as you go” when it comes to data science. Still, you may not want to feel like you’re stuck in a lower role for several years when you could advance faster with a Master’s under your belt. So, consider charting your ideal career course, with the positions that best align with your goals, to figure out if you’ll need a Master’s to get you to where you want to go.
Current Level of Education and Experience
Some of the options for getting into data science aren’t available to those with limited experience. For example, anybody can make their start with books and articles, which have no barrier to entry. But many internships require demonstrable proof that you understand various programming and scripting languages, with some also asking to see evidence of formal education. As for a Master’s degree, you’ll need a BSc in computer science (or an equivalent degree) to walk down that path.
Financial Considerations
Money makes the educational wheel turn, at least when it comes to formal education. As mentioned, a Master’s in data science can set you back up to $50,000, which may sting (and even be unfeasible) if you already have student loans to pay off for an undergraduate degree. Online courses are more cost-effective (and offer certification), while bootcamps and competitions can either pay you for learning or set you up in a career if you succeed.
Time Commitment and Flexibility
The simple question here is how long do you want to wait to start your career in data science? The patient person can afford to spend a couple of years earning their Master’s degree, and will benefit from having formal and respectable proof of their skills when they’re done. But if you want to get started right now, internships combined with more flexible online courses may provide a faster route to your goal.
A Master’s Degree – Do You Need It to Master Data Science?
Everybody’s answer is different when they ask themselves “do I need a Master’s in data science?” Some prefer the formalized approach that a Master’s offers, along with the exposure to industry professionals that may set them up for strong careers in the future. Others are less patient, preferring to quickly develop skills in a bootcamp, while yet others want a more free-form educational experience that is malleable to their needs and time constraints.
In the end, your circumstances, career goals, and educational preferences are the main factors when deciding which route to take. A Master’s degree is never a bad thing to have on your resume, but it’s not essential for a career in data science. Explore your options and choose whatever works best for you.
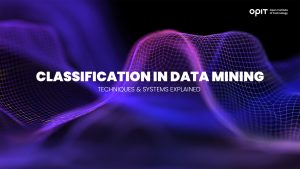
Data mining is an essential process for many businesses, including McDonald’s and Amazon. It involves analyzing huge chunks of unprocessed information to discover valuable insights. It’s no surprise large organizations rely on data mining, considering it helps them optimize customer service, reduce costs, and streamline their supply chain management.
Although it sounds simple, data mining is comprised of numerous procedures that help professionals extract useful information, one of which is classification. The role of this process is critical, as it allows data specialists to organize information for easier analysis.
This article will explore the importance of classification in greater detail. We’ll explain classification in data mining and the most common techniques.
Classification in Data Mining
Answering your question, “What is classification in data mining?” isn’t easy. To help you gain a better understanding of this term, we’ll cover the definition, purpose, and applications of classification in different industries.
Definition of Classification
Classification is the process of grouping related bits of information in a particular data set. Whether you’re dealing with a small or large set, you can utilize classification to organize the information more easily.
Purpose of Classification in Data Mining
Defining the classification of data mining systems is important, but why exactly do professionals use this method? The reason is simple – classification “declutters” a data set. It makes specific information easier to locate.
In this respect, think of classification as tidying up your bedroom. By organizing your clothes, shoes, electronics, and other items, you don’t have to waste time scouring the entire place to find them. They’re neatly organized and retrievable within seconds.
Applications of Classification in Various Industries
Here are some of the most common applications of data classification to help further demystify this process:
- Healthcare – Doctors can use data classification for numerous reasons. For example, they can group certain indicators of a disease for improved diagnostics. Likewise, classification comes in handy when grouping patients by age, condition, and other key factors.
- Finance – Data classification is essential for financial institutions. Banks can group information about consumers to find lenders more easily. Furthermore, data classification is crucial for elevating security.
- E-commerce – A key feature of online shopping platforms is recommending your next buy. They do so with the help of data classification. A system can analyze your previous decisions and group the related information to enhance recommendations.
- Weather forecast – Several considerations come into play during a weather forecast, including temperatures and humidity. Specialists can use a data mining platform to classify these considerations.
Techniques for Classification in Data Mining
Even though all data classification has a common goal (making information easily retrievable), there are different ways to accomplish it. In other words, you can incorporate an array of classification techniques in data mining.
Decision Trees
The decision tree method might be the most widely used classification technique. It’s a relatively simple yet effective method.
Overview of Decision Trees
Decision trees are like, well, trees, branching out in different directions. In the case of data mining, these trees have two branches: true and false. This method tells you whether a feature is true or false, allowing you to organize virtually any information.
Advantages and Disadvantages
Advantages:
- Preparing information in decision trees is simple.
- No normalization or scaling is involved.
- It’s easy to explain to non-technical staff.
Disadvantages:
- Even the tiniest of changes can transform the entire structure.
- Training decision tree-based models can be time-consuming.
- It can’t predict continuous values.
Support Vector Machines (SVM)
Another popular classification involves the use of support vector machines.
Overview of SVM
SVMs are algorithms that divide a dataset into two groups. It does so while ensuring there’s maximum distance from the margins of both groups. Once the algorithm categorizes information, it provides a clear boundary between the two groups.
Advantages and Disadvantages
Advantages:
- It requires minimal space.
- The process consumes little memory.
Disadvantages:
- It may not work well in large data sets.
- If the dataset has more features than training data samples, the algorithm might not be very accurate.
Naïve Bayes Classifier
The Naïve Bayes is also a viable option for classifying information.
Overview of Naïve Bayes Classifier
The Naïve Bayes method is a robust classification solution that makes predictions based on historical information. It tells you the likelihood of an event after analyzing how many times a similar (or the same) event has taken place. The most frequent application of this algorithm is distinguishing non-spam emails from billions of spam messages.
Advantages and Disadvantages
Advantages:
- It’s a fast, time-saving algorithm.
- Minimal training data is needed.
- It’s perfect for problems with multiple classes.
Disadvantages:
- Smoothing techniques are often required to fix noise.
- Estimates can be inaccurate.
K-Nearest Neighbors (KNN)
Although algorithms used for classification in data mining are complex, some have a simple premise. KNN is one of those algorithms.
Overview of KNN
Like many other algorithms, KNN starts with training data. From there, it determines the distance between particular objects. Items that are close to each other are considered related, which means that this system uses proximity to classify data.
Advantages and Disadvantages
Advantages:
- The implementation is simple.
- You can add new information whenever necessary without affecting the original data.
Disadvantages:
- The system can be computationally intensive, especially with large data sets.
- Calculating distances in large data sets is also expensive.
Artificial Neural Networks (ANN)
You might be wondering, “Is there a data classification technique that works like our brain?” Artificial neural networks may be the best example of such methods.
Overview of ANN
ANNs are like your brain. Just like the brain has connected neurons, ANNs have artificial neurons known as nodes that are linked to each other. Classification methods relying on this technique use the nodes to determine the category to which an object belongs.
Advantages and Disadvantages
Advantages:
- It can be perfect for generalization in natural language processing and image recognition since they can recognize patterns.
- The system works great for large data sets, as they render large chunks of information rapidly.
Disadvantages:
- It needs lots of training information and is expensive.
- The system can potentially identify non-existent patterns, which can make it inaccurate.
Comparison of Classification Techniques
It’s difficult to weigh up data classification techniques because there are significant differences. That’s not to say analyzing these models is like comparing apples to oranges. There are ways to determine which techniques outperform others when classifying particular information:
- ANNs generally work better than SVMs for making predictions.
- Decision trees are harder to design than some other, more complex solutions, such as ANNs.
- KNNs are typically more accurate than Naïve Bayes, which is rife with imprecise estimates.
Systems for Classification in Data Mining
Classifying information manually would be time-consuming. Thankfully, there are robust systems to help automate different classification techniques in data mining.
Overview of Data Mining Systems
Data mining systems are platforms that utilize various methods of classification in data mining to categorize data. These tools are highly convenient, as they speed up the classification process and have a multitude of applications across industries.
Popular Data Mining Systems for Classification
Like any other technology, classification of data mining systems becomes easier if you use top-rated tools:
WEKA
How often do you need to add algorithms from your Java environment to classify a data set? If you do it regularly, you should use a tool specifically designed for this task – WEKA. It’s a collection of algorithms that performs a host of data mining projects. You can apply the algorithms to your own code or directly into the platform.
RapidMiner
If speed is a priority, consider integrating RapidMiner into your environment. It produces highly accurate predictions in double-quick time using deep learning and other advanced techniques in its Java-based architecture.
Orange
Open-source platforms are popular, and it’s easy to see why when you consider Orange. It’s an open-source program with powerful classification and visualization tools.
KNIME
KNIME is another open-source tool you can consider. It can help you classify data by revealing hidden patterns in large amounts of information.
Apache Mahout
Apache Mahout allows you to create algorithms of your own. Each algorithm developed is scalable, enabling you to transfer your classification techniques to higher levels.
Factors to Consider When Choosing a Data Mining System
Choosing a data mining system is like buying a car. You need to ensure the product has particular features to make an informed decision:
- Data classification techniques
- Visualization tools
- Scalability
- Potential issues
- Data types
The Future of Classification in Data Mining
No data mining discussion would be complete without looking at future applications.
Emerging Trends in Classification Techniques
Here are the most important data classification facts to keep in mind for the foreseeable future:
- The amount of data should rise to 175 billion terabytes by 2025.
- Some governments may lift certain restrictions on data sharing.
- Data automation is expected to be further automated.
Integration of Classification With Other Data Mining Tasks
Classification is already an essential task. Future platforms may combine it with clustering, regression, sequential patterns, and other techniques to optimize the process. More specifically, experts may use classification to better organize data for subsequent data mining efforts.
The Role of Artificial Intelligence and Machine Learning in Classification
Nearly 20% of analysts predict machine learning and artificial intelligence will spearhead the development of classification strategies. Hence, mastering these two technologies may become essential.
Data Knowledge Declassified
Various methods for data classification in data mining, like decision trees and ANNs, are a must-have in today’s tech-driven world. They help healthcare professionals, banks, and other industry experts organize information more easily and make predictions.
To explore this data mining topic in greater detail, consider taking a course at an accredited institution. You’ll learn the ins and outs of data classification as well as expand your career options.
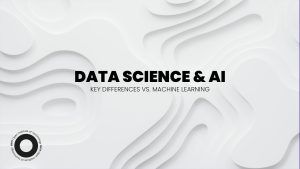
Machine learning, data science, and artificial intelligence are common terms in modern technology. These terms are often used interchangeably but incorrectly, which is understandable.
After all, hundreds of millions of people use the advantages of digital technologies. Yet only a small percentage of those users are experts in the field.
AI, data science, and machine learning represent valuable assets that can be used to great advantage in various industries. However, to use these tools properly, you need to understand what they are. Furthermore, knowing the difference between data science and machine learning, as well as how AI differs from both, can dispel the common misconceptions about these technologies.
Read on to gain a better understanding of the three crucial tech concepts.
Data Science
Data science can be viewed as the foundation of many modern technological solutions. It’s also the stage from which existing solutions can progress and evolve. Let’s define data science in more detail.
Definition and Explanation of Data Science
A scientific discipline with practical applications, data science represents a field of study dedicated to the development of data systems. If this definition sounds too broad, that’s because data science is a broad field by its nature.
Data structure is the primary concern of data science. To produce clean data and conduct analysis, scientists use a range of methods and tools, from manual to automated solutions.
Data science has another crucial task: defining problems that previously didn’t exist or slipped by unnoticed. Through this activity, data scientists can help predict unforeseen issues, improve existing digital tools, and promote the development of new ones.
Key Components of Data Science
Breaking down data science into key components, we get to three essential factors:
- Data collection
- Data analysis
- Predictive modeling
Data collection is pretty much what it sounds like – gathering of data. This aspect of data science also includes preprocessing, which is essentially preparation of raw data for further processing.
During data analysis, data scientists draw conclusions based on the gathered data. They search the data for patterns and potential flaws. The scientists do this to determine weak points and system deficiencies. In data visualization, scientists aim to communicate the conclusions of their investigation through graphics, charts, bullet points, and maps.
Finally, predictive modeling represents one of the ultimate uses of the analyzed data. Here, create models that can help them predict future trends. This component also illustrates the differentiation between data science vs. machine learning. Machine learning is often used in predictive modeling as a tool within the broader field of data science.
Applications and Use Cases of Data Science
Data science finds uses in marketing, banking, finance, logistics, HR, and trading, to name a few. Financial institutions and businesses take advantage of data science to assess and manage risks. The powerful assistance of data science often helps these organizations gain the upper hand in the market.
In marketing, data science can provide valuable information about customers, help marketing departments organize, and launch effective targeted campaigns. When it comes to human resources, extensive data gathering, and analysis allow HR departments to single out the best available talent and create accurate employee performance projections.
Artificial Intelligence (AI)
The term “artificial intelligence” has been somewhat warped by popular culture. Despite the varying interpretations, AI is a concrete technology with a clear definition and purpose, as well as numerous applications.
Definition and Explanation of AI
Artificial intelligence is sometimes called machine intelligence. In its essence, AI represents a machine simulation of human learning and decision-making processes.
AI gives machines the function of empirical learning, i.e., using experiences and observations to gain new knowledge. However, machines can’t acquire new experiences independently. They need to be fed relevant data for the AI process to work.
Furthermore, AI must be able to self-correct so that it can act as an active participant in improving its abilities.
Obviously, AI represents a rather complex technology. We’ll explain its key components in the following section.
Key Components of AI
A branch of computer science, AI includes several components that are either subsets of one another or work in tandem. These are machine learning, deep learning, natural language processing (NLP), computer vision, and robotics.
It’s no coincidence that machine learning popped up at the top spot here. It’s a crucial aspect of AI that does precisely what the name says: enables machines to learn.
We’ll discuss machine learning in a separate section.
Deep learning relates to machine learning. Its aim is essentially to simulate the human brain. To that end, the technology utilizes neural networks alongside complex algorithm structures that allow the machine to make independent decisions.
Natural language processing (NLP) allows machines to comprehend language similarly to humans. Language processing and understanding are the primary tasks of this AI branch.
Somewhat similar to NLP, computer vision allows machines to process visual input and extract useful data from it. And just as NLP enables a computer to understand language, computer vision facilitates a meaningful interpretation of visual information.
Finally, robotics are AI-controlled machines that can replace humans in dangerous or extremely complex tasks. As a branch of AI, robotics differs from robotic engineering, which focuses on the mechanical aspects of building machines.
Applications and Use Cases of AI
The variety of AI components makes the technology suitable for a wide range of applications. Machine and deep learning are extremely useful in data gathering. NLP has seen a massive uptick in popularity lately, especially with tools like ChatGPT and similar chatbots. And robotics has been around for decades, finding use in various industries and services, in addition to military and space applications.
Machine Learning
Machine learning is an AI branch that’s frequently used in data science. Defining what this aspect of AI does will largely clarify its relationship to data science and artificial intelligence.
Definition and Explanation of Machine Learning
Machine learning utilizes advanced algorithms to detect data patterns and interpret their meaning. The most important facets of machine learning include handling various data types, scalability, and high-level automation.
Like AI in general, machine learning also has a level of complexity to it, consisting of several key components.
Key Components of Machine Learning
The main aspects of machine learning are supervised, unsupervised, and reinforcement learning.
Supervised learning trains algorithms for data classification using labeled datasets. Simply put, the data is first labeled and then fed into the machine.
Unsupervised learning relies on algorithms that can make sense of unlabeled datasets. In other words, external intervention isn’t necessary here – the machine can analyze data patterns on its own.
Finally, reinforcement learning is the level of machine learning where the AI can learn to respond to input in an optimal way. The machine learns correct behavior through observation and environmental interactions without human assistance.
Applications and Use Cases of Machine Learning
As mentioned, machine learning is particularly useful in data science. The technology makes processing large volumes of data much easier while producing more accurate results. Supervised and particularly unsupervised learning are especially helpful here.
Reinforcement learning is most efficient in uncertain or unpredictable environments. It finds use in robotics, autonomous driving, and all situations where it’s impossible to pre-program machines with sufficient accuracy.
Perhaps most famously, reinforcement learning is behind AlphaGo, an AI program developed for the Go board game. The game is notorious for its complexity, having about 250 possible moves on each of 150 turns, which is how long a typical game lasts.
Alpha Go managed to defeat the human Go champion by getting better at the game through numerous previous matches.
Key Differences Between Data Science, AI, and Machine Learning
The differences between machine learning, data science, and artificial intelligence are evident in the scope, objectives, techniques, required skill sets, and application.
As a subset of AI and a frequent tool in data science, machine learning has a more closely defined scope. It’s structured differently to data science and artificial intelligence, both massive fields of study with far-reaching objectives.
The objectives of data science are pto gather and analyze data. Machine learning and AI can take that data and utilize it for problem-solving, decision-making, and to simulate the most complex traits of the human brain.
Machine learning has the ultimate goal of achieving high accuracy in pattern comprehension. On the other hand, the main task of AI in general is to ensure success, particularly in emulating specific facets of human behavior.
All three require specific skill sets. In the case of data science vs. machine learning, the sets don’t match. The former requires knowledge of SQL, ETL, and domains, while the latter calls for Python, math, and data-wrangling expertise.
Naturally, machine learning will have overlapping skill sets with AI, since it’s its subset.
Finally, in the application field, data science produces valuable data-driven insights, AI is largely used in virtual assistants, while machine learning powers search engine algorithms.
How Data Science, AI, and Machine Learning Complement Each Other
Data science helps AI and machine learning by providing accurate, valuable data. Machine learning is critical in processing data and functions as a primary component of AI. And artificial intelligence provides novel solutions on all fronts, allowing for more efficient automation and optimal processes.
Through the interaction of data science, AI, and machine learning, all three branches can develop further, bringing improvement to all related industries.
Understanding the Technology of the Future
Understanding the differences and common uses of data science, AI, and machine learning is essential for professionals in the field. However, it can also be valuable for businesses looking to leverage modern and future technologies.
As all three facets of modern tech develop, it will be important to keep an eye on emerging trends and watch for future developments.
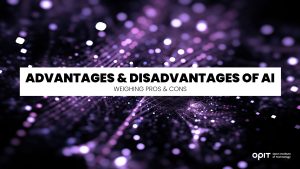
Artificial intelligence has impacted on businesses since its development in the 1940s. By automating various tasks, it increases security, streamlines inventory management, and provides many other tremendous benefits. Additionally, it’s expected to grow at a rate of nearly 40% until the end of the decade.
However, the influence of artificial intelligence goes both ways. There are certain disadvantages to consider to get a complete picture of this technology.
This article will cover the most important advantages and disadvantages of artificial intelligence.
Advantages of AI
Approximately 37% of all organizations embrace some form of AI to polish their operations. The numerous advantages help business owners take their enterprises to a whole new level.
Increased Efficiency and Productivity
One of the most significant advantages of artificial intelligence is elevated productivity and efficiency.
Automation of Repetitive Tasks
How many times have you thought to yourself: “I really wish there was a better way to take care of this mundane task.” There is – incorporate artificial intelligence into your toolbox.
You can program this technology to perform basically anything. Whether you need to go through piles of documents or adjust print settings, a machine can do the work for you. Just set the parameters, and you can sit back while AI does the rest.
Faster Data Processing and Analysis
You probably deal with huge amounts of information. Manual processing and analysis can be time-consuming, but not if you outsource the project to AI. Artificial intelligence can breeze through vast chunks of data much faster than people.
Improved Decision-Making
AI makes all the difference with decision-making through data-driven insights and the reduction of human error.
Data-Driven Insights
AI software gathers and analyzes data from relevant sources. Decision-makers can use this highly accurate information to make an informed decision and predict future trends.
Reduction of Human Error
Burnout can get the better of anyone and increase the chances of making a mistake. That’s not what happens with AI. If correctly programmed, it can carry out virtually any task, and the chances of error are slim to none.
Enhanced Customer Experience
Artificial intelligence can also boost customer experience.
Personalized Recommendations
AI machines can use data to recommend products and services. The technology reduces the need for manual input to further automate repetitive tasks. One of the most famous platforms with AI-based recommendations is Netflix.
Chatbots and Virtual Assistants
Many enterprises set up AI-powered chatbots and virtual assistants to communicate with customers and help them troubleshoot various issues. Likewise, these platforms can help clients find a certain page or blog on a website.
Innovation and Creativity
Contrary to popular belief, one of the biggest advantages of artificial intelligence is that it can promote innovation and creativity.
AI-Generated Content and Designs
AI can create some of the most mesmerizing designs imaginable. Capable of producing stunning content, whether in the written, video, or audio format, it also works at unprecedented speeds.
Problem-Solving Capabilities
Sophisticated AI tools can solve a myriad of problems, including math, coding, and architecture. Simply describe your problem and wait for the platform to apply its next-level skills.
Cost Savings
According to McKinsey & Company, you can decrease costs by 15%-20% in less than two years by implementing AI in your workplace. Two main factors underpin this reduction.
Reduced Labor Costs
Before AI became widespread, many tasks could only be performed by humans, such as contact management and inventory tracking. Nowadays, artificial intelligence can take on those responsibilities and cut labor costs.
Lower Operational Expenses
As your enterprise becomes more efficient through AI implementation, you reduce errors and lower operational expenses.
Disadvantages of AI
AI does have a few drawbacks. Understanding the disadvantages of artificial intelligence is key to making the right decision on the adoption of this technology.
Job Displacement and Unemployment
The most obvious disadvantage is redundancies. Many people lose their jobs because their position becomes obsolete. Organizations prioritize cost cutting, which is why they often lay off employees in favor of AI.
Automation Replacing Human Labor
This point is directly related to the previous one. Even though AI-based automation is beneficial from a time and money-saving perspective, it’s a major problem for employees. Those who perform repetitive tasks are at risk of losing their position.
Need for Workforce Reskilling
Like any other workplace technology, artificial intelligence requires people to learn additional skills. Since some abilities may become irrelevant due to AI-powered automation, job seekers need to pick up more practical skills that can’t be replaced by AI.
Ethical Concerns
In addition to increasing unemployment, artificial intelligence can also raise several ethical concerns.
Bias and Discrimination in AI Algorithms
AI algorithms are sophisticated, but they’re not perfect. The main reason being that developers inject their personal biases into the AI-based tool. Consequently, content and designs created through AI may contain subjective themes that might not resonate with some audiences.
Privacy and Surveillance Issues
One of the most serious disadvantages of artificial intelligence is that it can infringe on people’s privacy. Some platforms gather information about individuals without their consent. Even though it may achieve a greater purpose, many people aren’t willing to sacrifice their right to privacy.
High Initial Investment and Maintenance Costs
As cutting-edge technology, Artificial Intelligence is also pricey.
Expensive AI Systems and Infrastructure
The cost of developing a custom AI solution can be upwards of $200,000. Hence, it can be a financial burden.
Ongoing Updates and Improvements
Besides the initial investment, you also need to release regular updates and improvements to streamline the AI platform. All of which quickly adds up.
Dependence on Technology
While reliance on technology has its benefits, there are a few disadvantages.
Loss of Human Touch and Empathy
Although advanced, most AI tools fail to capture the magic of the human touch. They can’t empathize with the target audience, either, making the content less impactful.
Overreliance on AI Systems
If you become overly reliant on an AI solution, your problem-solving skills suffer and you might not know how to complete a project if the system fails.
Security Risks
AI tools aren’t impervious to security risks. Far from it – many risks arise when utilizing this technology.
Vulnerability to Cyberattacks
Hackers can tap into the AI network by adding training files the tool considers safe. Before you know it, the malware spreads and wreaks havoc on the infrastructure.
Misuse of AI Technology
Malicious users often have dishonorable intentions with AI software. They can use it to create deep fakes or execute phishing attacks to steal information.
AI in Various Industries: Pros and Cons
Let’s go through the pros and cons of using AI in different industries.
Healthcare
Advantages:
- Improved Diagnostics – AI can drastically speed up the diagnostics process.
- Personalized Treatment – Artificial intelligence can provide personalized treatment recommendations.
- Drug Development – AI algorithms can scan troves of information to help develop drugs.
Disadvantages:
- Privacy Concerns – Systems can collect patient and doctor data without their permission.
- High Costs – Implementing an AI system might be too expensive for many hospitals.
- Potential Misdiagnosis – An AI machine may overlook certain aspects during diagnosis.
Finance
Advantages:
- Fraud Detection – AI-powered data collection and analysis is perfect for preventing financial fraud.
- Risk Assessment – Automated reports and monitoring expedite and optimize risk assessment.
- Algorithmic Trading – A computer can capitalize on specific market conditions automatically to increase profits.
Disadvantages:
- Job Displacement – Risk assessment professionals and other specialists could become obsolete due to AI.
- Ethical Concerns – Artificial intelligence may use questionable data collection practices.
- Security Risks – A cybercriminal can compromise an AI system of a bank, allowing them to steal customer data.
Manufacturing
Advantages:
- Increased Efficiency – You can set product dimensions, weight, and other parameters automatically with AI.
- Reduced Waste – Artificial intelligence is more accurate than humans, reducing waste in manufacturing facilities.
- Improved Safety – Lower manual input leads to fewer workplace accidents.
Disadvantages:
- Job Displacement – AI implementation results in job loss in most fields. Manufacturing is no exception.
- High Initial Investment – Production companies typically need $200K+ to develop a tailor-made AI system.
- Dependence on Technology – AI manufacturing programs may require tweaks after some time, which is hard to do if you become overly reliant on the software.
Education
Advantages:
- Personalized Learning – An AI program can recommend appropriate textbooks, courses, and other resources.
- Adaptive Assessments – AI-operated systems adapt to the learner’s needs for greater retention.
- Virtual Tutors – Schools can reduce labor costs with virtual tutors.
Disadvantages:
- Privacy Concerns – Data may be at risk in an AI classroom.
- Digital Divide – Some nations don’t have the same access to technology as others, leading to so-called digital divide.
- Loss of Human Interaction – Teachers empathize and interact with their learners on a profound level, which can’t be said for AI.
AI Is Mighty But Warrants Caution
People rely on AI for higher efficiency, productivity, innovation, and automation. At the same time, it’s expensive, raises unemployment, and causes many privacy concerns.
That’s why you should be aware of the advantages and disadvantages of artificial intelligence. Striking a balance between the good and bad sides is vital for effective yet ethical implementation.
If you wish to learn more about AI and its uses across industries, consider taking a course by renowned tech experts.
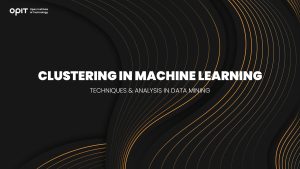
How do machine learning professionals make data readable and accessible? What techniques do they use to dissect raw information?
One of these techniques is clustering. Data clustering is the process of grouping items in a data set together. These items are related, allowing key stakeholders to make critical strategic decisions using the insights.
After preparing data, which is what specialists do 50%-80% of the time, clustering takes center stage. It forms structures other members of the company can understand more easily, even if they lack advanced technical knowledge.
Clustering in machine learning involves many techniques to help accomplish this goal. Here is a detailed overview of those techniques.
Clustering Techniques
Data science is an ever-changing field with lots of variables and fluctuations. However, one thing’s for sure – whether you want to practice clustering in data mining or clustering in machine learning, you can use a wide array of tools to automate your efforts.
Partitioning Methods
The first groups of techniques are the so-called partitioning methods. There are three main sub-types of this model.
K-Means Clustering
K-means clustering is an effective yet straightforward clustering system. To execute this technique, you need to assign clusters in your data sets. From there, define your number K, which tells the program how many centroids (“coordinates” representing the center of your clusters) you need. The machine then recognizes your K and categorizes data points to nearby clusters.
You can look at K-means clustering like finding the center of a triangle. Zeroing in on the center lets you divide the triangle into several areas, allowing you to make additional calculations.
And the name K-means clustering is pretty self-explanatory. It refers to finding the median value of your clusters – centroids.
K-Medoids Clustering
K-means clustering is useful but is prone to so-called “outlier data.” This information is different from other data points and can merge with others. Data miners need a reliable way to deal with this issue.
Enter K-medoids clustering.
It’s similar to K-means clustering, but just like planes overcome gravity, so does K-medoids clustering overcome outliers. It utilizes “medoids” as the reference points – which contain maximum similarities with other data points in your cluster. As a result, no outliers interfere with relevant data points, making this one of the most dependable clustering techniques in data mining.
Fuzzy C-Means Clustering
Fuzzy C-means clustering is all about calculating the distance from the median point to individual data points. If a data point is near the cluster centroid, it’s relevant to the goal you want to accomplish with your data mining. The farther you go from this point, the farther you move the goalpost and decrease relevance.
Hierarchical Methods
Some forms of clustering in machine learning are like textbooks – similar topics are grouped in a chapter and are different from topics in other chapters. That’s precisely what hierarchical clustering aims to accomplish. You can the following methods to create data hierarchies.
Agglomerative Clustering
Agglomerative clustering is one of the simplest forms of hierarchical clustering. It divides your data set into several clusters, making sure data points are similar to other points in the same cluster. By grouping them, you can see the differences between individual clusters.
Before the execution, each data point is a full-fledged cluster. The technique helps you form more clusters, making this a bottom-up strategy.
Divisive Clustering
Divisive clustering lies on the other end of the hierarchical spectrum. Here, you start with just one cluster and create more as you move through your data set. This top-down approach produces as many clusters as necessary until you achieve the requested number of partitions.
Density-Based Methods
Birds of a feather flock together. That’s the basic premise of density-based methods. Data points that are close to each other form high-density clusters, indicating their cohesiveness. The two primary density-based methods of clustering in data mining are DBSCAN and OPTICS.
DBSCAN (Density-Based Spatial Clustering of Applications With Noise)
Related data groups are close to each other, forming high-density areas in your data sets. The DBSCAN method picks up on these areas and groups information accordingly.
OPTICS (Ordering Points to Identify the Clustering Structure)
The OPTICS technique is like DBSCAN, grouping data points according to their density. The only major difference is that OPTICS can identify varying densities in larger groups.
Grid-Based Methods
You can see grids on practically every corner. They can easily be found in your house or your car. They’re also prevalent in clustering.
STING (Statistical Information Grid)
The STING grid method divides a data point into rectangular grills. Afterward, you determine certain parameters for your cells to categorize information.
CLIQUE (Clustering in QUEst)
Agglomerative clustering isn’t the only bottom-up clustering method on our list. There’s also the CLIQUE technique. It detects clusters in your environment and combines them according to your parameters.
Model-Based Methods
Different clustering techniques have different assumptions. The assumption of model-based methods is that a model generates specific data points. Several such models are used here.
Gaussian Mixture Models (GMM)
The aim of Gaussian mixture models is to identify so-called Gaussian distributions. Each distribution is a cluster, and any information within a distribution is related.
Hidden Markov Models (HMM)
Most people use HMM to determine the probability of certain outcomes. Once they calculate the probability, they can figure out the distance between individual data points for clustering purposes.
Spectral Clustering
If you often deal with information organized in graphs, spectral clustering can be your best friend. It finds related groups of notes according to linked edges.
Comparison of Clustering Techniques
It’s hard to say that one algorithm is superior to another because each has a specific purpose. Nevertheless, some clustering techniques might be especially useful in particular contexts:
- OPTICS beats DBSCAN when clustering data points with different densities.
- K-means outperforms divisive clustering when you wish to reduce the distance between a data point and a cluster.
- Spectral clustering is easier to implement than the STING and CLIQUE methods.
Cluster Analysis
You can’t put your feet up after clustering information. The next step is to analyze the groups to extract meaningful information.
Importance of Cluster Analysis in Data Mining
The importance of clustering in data mining can be compared to the importance of sunlight in tree growth. You can’t get valuable insights without analyzing your clusters. In turn, stakeholders wouldn’t be able to make critical decisions about improving their marketing efforts, target audience, and other key aspects.
Steps in Cluster Analysis
Just like the production of cars consists of many steps (e.g., assembling the engine, making the chassis, painting, etc.), cluster analysis is a multi-stage process:
Data Preprocessing
Noise and other issues plague raw information. Data preprocessing solves this issue by making data more understandable.
Feature Selection
You zero in on specific features of a cluster to identify those clusters more easily. Plus, feature selection allows you to store information in a smaller space.
Clustering Algorithm Selection
Choosing the right clustering algorithm is critical. You need to ensure your algorithm is compatible with the end result you wish to achieve. The best way to do so is to determine how you want to establish the relatedness of the information (e.g., determining median distances or densities).
Cluster Validation
In addition to making your data points easily digestible, you also need to verify whether your clustering process is legit. That’s where cluster validation comes in.
Cluster Validation Techniques
There are three main cluster validation techniques when performing clustering in machine learning:
Internal Validation
Internal validation evaluates your clustering based on internal information.
External Validation
External validation assesses a clustering process by referencing external data.
Relative Validation
You can vary your number of clusters or other parameters to evaluate your clustering. This procedure is known as relative validation.
Applications of Clustering in Data Mining
Clustering may sound a bit abstract, but it has numerous applications in data mining.
- Customer Segmentation – This is the most obvious application of clustering. You can group customers according to different factors, like age and interests, for better targeting.
- Anomaly Detection – Detecting anomalies or outliers is essential for many industries, such as healthcare.
- Image Segmentation – You use data clustering if you want to recognize a certain object in an image.
- Document Clustering – Organizing documents is effortless with document clustering.
- Bioinformatics and Gene Expression Analysis – Grouping related genes together is relatively simple with data clustering.
Challenges and Future Directions
- Scalability – One of the biggest challenges of data clustering is expected to be applying the process to larger datasets. Addressing this problem is essential in a world with ever-increasing amounts of information.
- Handling High-Dimensional Data – Future systems may be able to cluster data with thousands of dimensions.
- Dealing with Noise and Outliers – Specialists hope to enhance the ability of their clustering systems to reduce noise and lessen the influence of outliers.
- Dynamic Data and Evolving Clusters – Updates can change entire clusters. Professionals will need to adapt to this environment to retain efficiency.
Elevate Your Data Mining Knowledge
There are a vast number of techniques for clustering in machine learning. From centroid-based solutions to density-focused approaches, you can take many directions when grouping data.
Mastering them is essential for any data miner, as they provide insights into crucial information. On top of that, the data science industry is expected to hit nearly $26 billion by 2026, which is why clustering will become even more prevalent.
Have questions?
Visit our FAQ page or get in touch with us!
Write us at +39 335 576 0263
Get in touch at hello@opit.com
Talk to one of our Study Advisors
We are international
We can speak in: