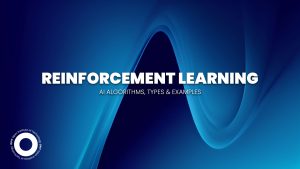
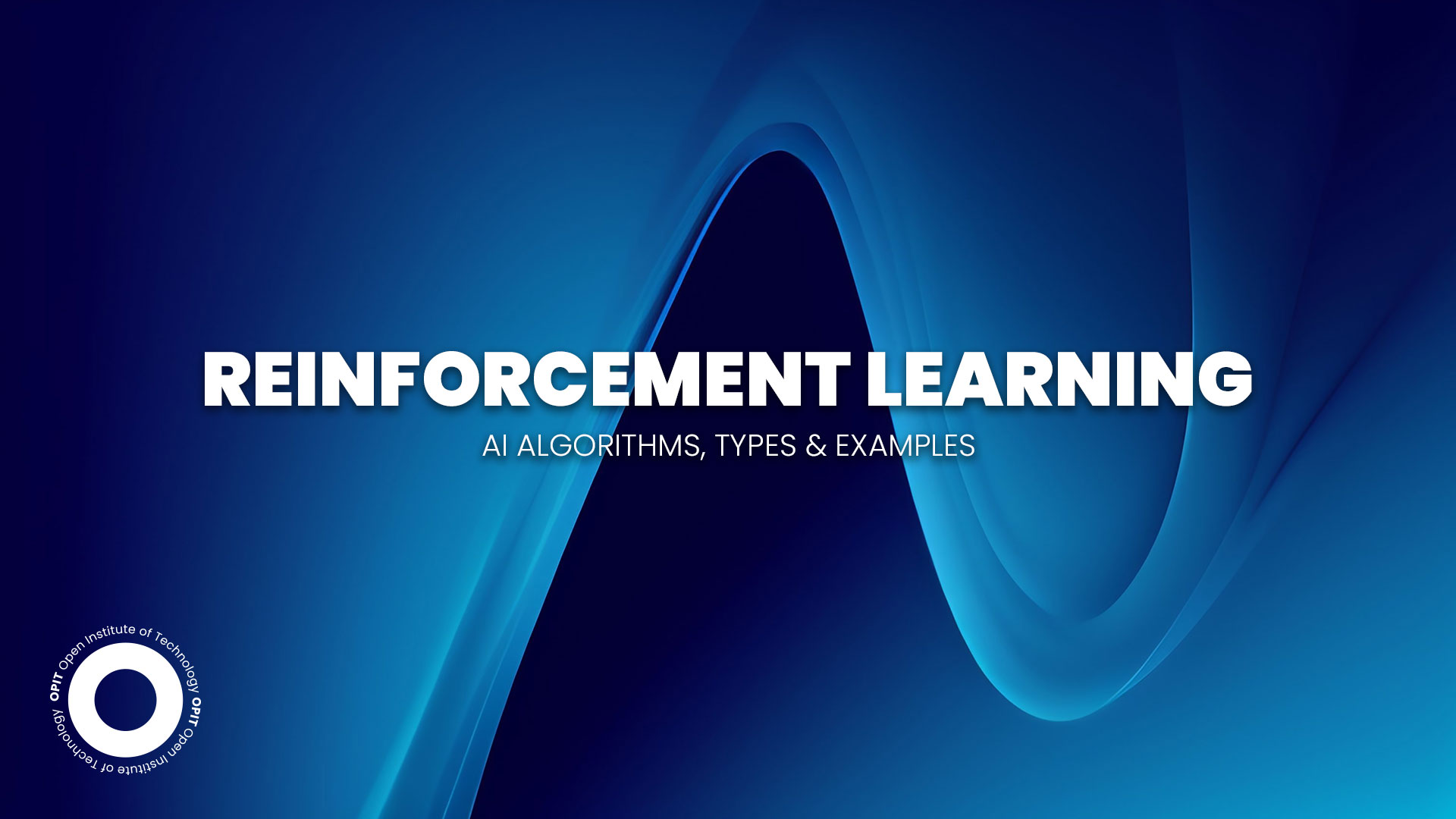
Reinforcement learning is a very useful (and currently popular) subtype of machine learning and artificial intelligence. It is based on the principle that agents, when placed in an interactive environment, can learn from their actions via rewards associated with the actions, and improve the time to achieve their goal.
In this article, we’ll explore the fundamental concepts of reinforcement learning and discuss its key components, types, and applications.
Definition of Reinforcement Learning
We can define reinforcement learning as a machine learning technique involving an agent who needs to decide which actions it needs to do to perform a task that has been assigned to it most effectively. For this, rewards are assigned to the different actions that the agent can take at different situations or states of the environment. Initially, the agent has no idea about the best or correct actions. Using reinforcement learning, it explores its action choices via trial and error and figures out the best set of actions for completing its assigned task.
The basic idea behind a reinforcement learning agent is to learn from experience. Just like humans learn lessons from their past successes and mistakes, reinforcement learning agents do the same – when they do something “good” they get a reward, but, if they do something “bad”, they get penalized. The reward reinforces the good actions while the penalty avoids the bad ones.
Reinforcement learning requires several key components:
- Agent – This is the “who” or the subject of the process, which performs different actions to perform a task that has been assigned to it.
- Environment – This is the “where” or a situation in which the agent is placed.
- Actions – This is the “what” or the steps an agent needs to take to reach the goal.
- Rewards – This is the feedback an agent receives after performing an action.
Before we dig deep into the technicalities, let’s warm up with a real-life example. Reinforcement isn’t new, and we’ve used it for different purposes for centuries. One of the most basic examples is dog training.
Let’s say you’re in a park, trying to teach your dog to fetch a ball. In this case, the dog is the agent, and the park is the environment. Once you throw the ball, the dog will run to catch it, and that’s the action part. When he brings the ball back to you and releases it, he’ll get a reward (a treat). Since he got a reward, the dog will understand that his actions were appropriate and will repeat them in the future. If the dog doesn’t bring the ball back, he may get some “punishment” – you may ignore him or say “No!” After a few attempts (or more than a few, depending on how stubborn your dog is), the dog will fetch the ball with ease.
We can say that the reinforcement learning process has three steps:
- Interaction
- Learning
- Decision-making
Types of Reinforcement Learning
There are two types of reinforcement learning: model-based and model-free.
Model-Based Reinforcement Learning
With model-based reinforcement learning (RL), there’s a model that an agent uses to create additional experiences. Think of this model as a mental image that the agent can analyze to assess whether particular strategies could work.
Some of the advantages of this RL type are:
- It doesn’t need a lot of samples.
- It can save time.
- It offers a safe environment for testing and exploration.
The potential drawbacks are:
- Its performance relies on the model. If the model isn’t good, the performance won’t be good either.
- It’s quite complex.
Model-Free Reinforcement Learning
In this case, an agent doesn’t rely on a model. Instead, the basis for its actions lies in direct interactions with the environment. An agent tries different scenarios and tests whether they’re successful. If yes, the agent will keep repeating them. If not, it will try another scenario until it finds the right one.
What are the advantages of model-free reinforcement learning?
- It doesn’t depend on a model’s accuracy.
- It’s not as computationally complex as model-based RL.
- It’s often better for real-life situations.
Some of the drawbacks are:
- It requires more exploration, so it can be more time-consuming.
- It can be dangerous because it relies on real-life interactions.
Model-Based vs. Model-Free Reinforcement Learning: Example
Understanding model-based and model-free RL can be challenging because they often seem too complex and abstract. We’ll try to make the concepts easier to understand through a real-life example.
Let’s say you have two soccer teams that have never played each other before. Therefore, neither of the teams knows what to expect. At the beginning of the match, Team A tries different strategies to see whether they can score a goal. When they find a strategy that works, they’ll keep using it to score more goals. This is model-free reinforcement learning.
On the other hand, Team B came prepared. They spent hours investigating strategies and examining the opponent. The players came up with tactics based on their interpretation of how Team A will play. This is model-based reinforcement learning.
Who will be more successful? There’s no way to tell. Team B may be more successful in the beginning because they have previous knowledge. But Team A can catch up quickly, especially if they use the right tactics from the start.
Reinforcement Learning Algorithms
A reinforcement learning algorithm specifies how an agent learns suitable actions from the rewards. RL algorithms are divided into two categories: value-based and policy gradient-based.
Value-Based Algorithms
Value-based algorithms learn the value at each state of the environment, where the value of a state is given by the expected rewards to complete the task while starting from that state.
Q-Learning
This model-free, off-policy RL algorithm focuses on providing guidelines to the agent on what actions to take and under what circumstances to win the reward. The algorithm uses Q-tables in which it calculates the potential rewards for different state-action pairs in the environment. The table contains Q-values that get updated after each action during the agent’s training. During execution, the agent goes back to this table to see which actions have the best value.
Deep Q-Networks (DQN)
Deep Q-networks, or deep q-learning, operate similarly to q-learning. The main difference is that the algorithm in this case is based on neural networks.
SARSA
The acronym stands for state-action-reward-state-action. SARSA is an on-policy RL algorithm that uses the current action from the current policy to learn the value.
Policy-Based Algorithms
These algorithms directly update the policy to maximize the reward. There are different policy gradient-based algorithms: REINFORCE, proximal policy optimization, trust region policy optimization, actor-critic algorithms, advantage actor-critic, deep deterministic policy gradient (DDPG), and twin-delayed DDPG.
Examples of Reinforcement Learning Applications
The advantages of reinforcement learning have been recognized in many spheres. Here are several concrete applications of RL.
Robotics and Automation
With RL, robotic arms can be trained to perform human-like tasks. Robotic arms can give you a hand in warehouse management, packaging, quality testing, defect inspection, and many other aspects.
Another notable role of RL lies in automation, and self-driving cars are an excellent example. They’re introduced to different situations through which they learn how to behave in specific circumstances and offer better performance.
Gaming and Entertainment
Gaming and entertainment industries certainly benefit from RL in many ways. From AlphaGo (the first program that has beaten a human in the board game Go) to video games AI, RL offers limitless possibilities.
Finance and Trading
RL can optimize and improve trading strategies, help with portfolio management, minimize risks that come with running a business, and maximize profit.
Healthcare and Medicine
RL can help healthcare workers customize the best treatment plan for their patients, focusing on personalization. It can also play a major role in drug discovery and testing, allowing the entire sector to get one step closer to curing patients quickly and efficiently.
Basics for Implementing Reinforcement Learning
The success of reinforcement learning in a specific area depends on many factors.
First, you need to analyze a specific situation and see which RL algorithm suits it. Your job doesn’t end there; now you need to define the environment and the agent and figure out the right reward system. Without them, RL doesn’t exist. Next, allow the agent to put its detective cap on and explore new features, but ensure it uses the existing knowledge adequately (strike the right balance between exploration and exploitation). Since RL changes rapidly, you want to keep your model updated. Examine it every now and then to see what you can tweak to keep your model in top shape.
Explore the World of Possibilities With Reinforcement Learning
Reinforcement learning goes hand-in-hand with the development and modernization of many industries. We’ve been witnesses to the incredible things RL can achieve when used correctly, and the future looks even better. Hop in on the RL train and immerse yourself in this fascinating world.
Related posts
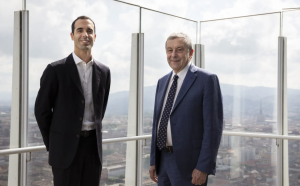
Source:
- EFMD Global, Published on July 12th, 2024.
By Stephanie Mullins
Many people love to read the stories of successful business school graduates to see what they’ve achieved using the lessons, insights and connections from the programmes they’ve studied. We speak to one alumnus, Riccardo Ocleppo, who studied at top business schools including London Business School (LBS) and INSEAD, about the education institution called OPIT which he created after business school.
Please introduce yourself and your career to date.
I am the founder of OPIT — Open Institute of Technology, a fully accredited Higher Education Institution (HEI) under the European Qualification Framework (EQF) by the MFHEA Authority. OPIT also partners with WES (World Education Services), a trusted non-profit providing verified education credential assessments (ECA) in the US and Canada for foreign degrees and certificates.
Prior to founding OPIT, I established Docsity, a global community boasting 15 million registered university students worldwide and partnerships with over 250 Universities and Business Schools. My academic background includes an MSc in Electronics from Politecnico di Torino and an MSc in Management from London Business School.
Why did you decide to create OPIT Open Institute of Technology?
Higher education has a profound impact on people’s futures. Through quality higher education, people can aspire to a better and more fulfilling future.
The mission behind OPIT is to democratise access to high-quality higher education in the fields that will be in high demand in the coming decades: Computer Science, Artificial Intelligence, Data Science, Cybersecurity, and Digital Innovation.
Since launching my first company in the education field, I’ve engaged with countless students, partnered with hundreds of universities, and collaborated with professors and companies. Through these interactions, I’ve observed a gap between traditional university curricula and the skills demanded by today’s job market, particularly in Computer Science and Technology.
I founded OPIT to bridge this gap by modernising education, making it affordable, and enhancing the digital learning experience. By collaborating with international professors and forging solid relationships with global companies, we are creating a dynamic online community and developing high-quality digital learning content. This approach ensures our students benefit from a flexible, cutting-edge, and stress-free learning environment.
Why do you think an education in tech is relevant in today’s business landscape?
As depicted by the World Economic Forum’s “Future of Jobs 2023” report, the demand for skilled tech professionals remains (and will remain) robust across industries, driven by the critical role of advanced technologies in business success.
Today’s companies require individuals who can innovate and execute complex solutions. A degree in fields like computer science, cybersecurity, data science, digital business or AI equips graduates with essential skills to thrive in this dynamic industry.
According to the International Monetary Fund (IMF), the global tech talent shortage will exceed 85 million workers by 2030. The Korn Ferry Institute warns that this gap could result in hundreds of billions in lost revenue across the US, Europe, and Asia.
To address this challenge, OPIT aims to democratise access to technology education. Our competency-based and applied approach, coupled with a flexible online learning experience, empowers students to progress at their own pace, demonstrating their skills as they advance.
Read the full article below:
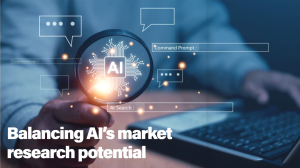
Source:
- The European, Summer 2024 Edition, Page 24
With careful planning, ethical considerations, and ensuring human oversight is maintained, AI can have huge market research benefits, says Lorenzo Livi of the Open Institute of Technology.
By Lorenzo Livi
To market well, you need to get something interesting in front of those who are interested. That takes a lot of thinking, a lot of work, and a whole bunch of research. But what if the bulk of that thinking, work and research could be done for you? What would that mean for marketing as an industry, and market research specifically?
With the recent explosion of AI onto the world stage, big changes are coming in the marketing industry. But will AI be able to do market research as successfully? Simply, the answer is yes. A big, fat, resounding yes. In fact, AI has the potential to revolutionise market research.
Ensuring that people have a clear understanding of what exactly AI is is crucial, given its seismic effect on our world. Common questions that even occur amongst people at the forefront of marketing, such as, “Who invented AI?” or, “Where is the main AI system located?” highlight a widespread misunderstanding about the nature of AI.
As for the notion of a central “main thing” running AI, it’s essential to clarify that AI systems exist in various forms and locations. AI algorithms and models can run on individual computers, servers, or even specialized hardware designed for AI processing, commonly referred to as AI chips. These systems can be distributed across multiple locations, including data centres, cloud platforms, and edge devices. They can also be used anywhere, so long as you have a compatible device and an internet connection.
While the concept of AI may seem abstract or mysterious to some, it’s important to approach it with a clear understanding of its principles and applications. By promoting education and awareness about AI, we can dispel misconceptions and facilitate meaningful conversations about its role in society.
Read the full article below:
- The European, Pages 24 to 26.
Have questions?
Visit our FAQ page or get in touch with us!
Write us at +39 335 576 0263
Get in touch at hello@opit.com
Talk to one of our Study Advisors
We are international
We can speak in: