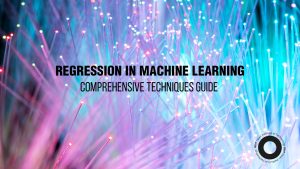
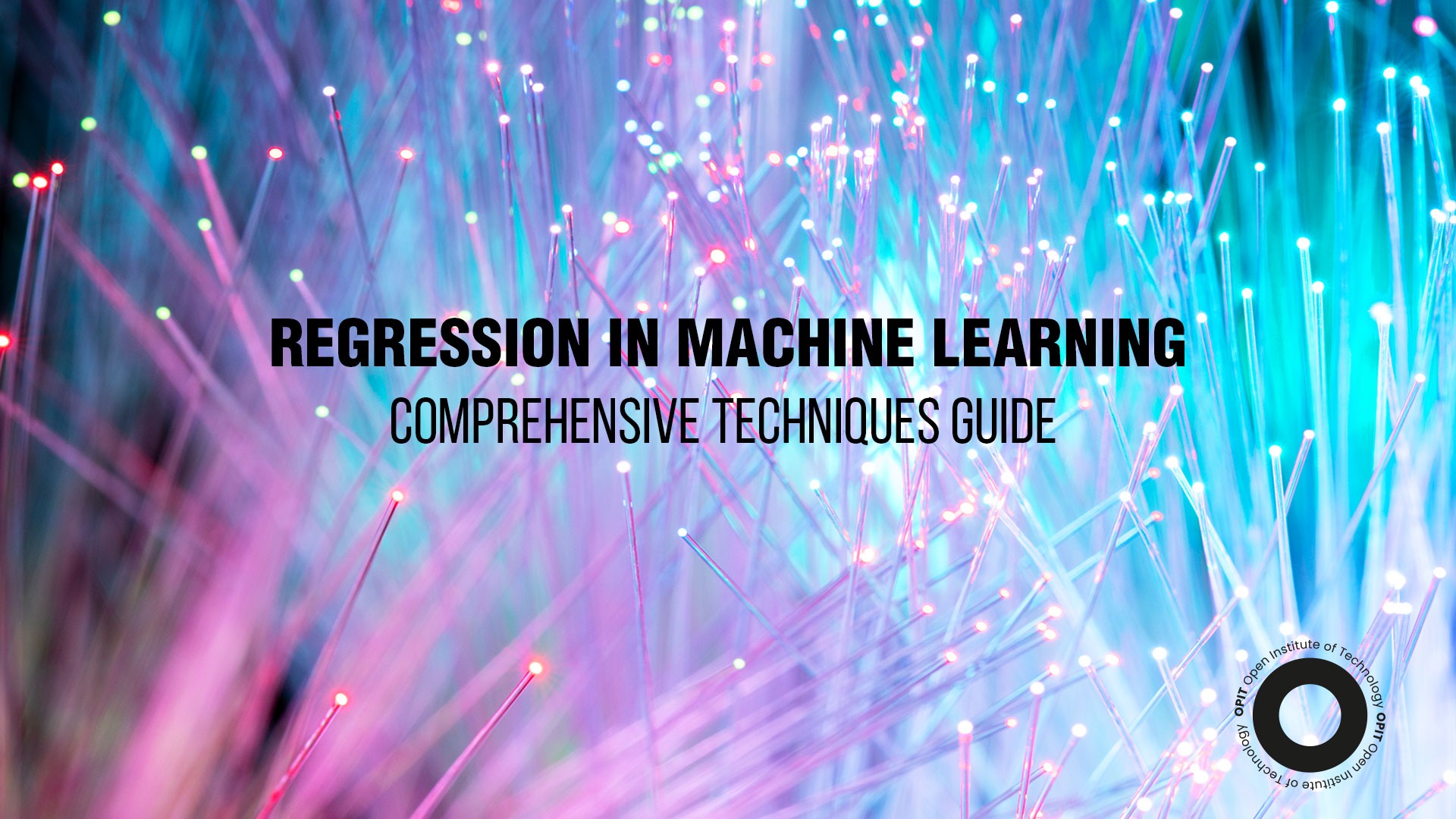
As artificial intelligence and machine learning are becoming present in almost every aspect of life, it’s essential to understand how they work and their common applications. Although machine learning has been around for a while, many still portray it as an enemy. Machine learning can be your friend, but only if you learn to “tame” it.
Regression stands out as one of the most popular machine-learning techniques. It serves as a bridge that connects the past to the present and future. It does so by picking up on different “events” from the past and breaking them apart to analyze them. Based on this analysis, regression can make conclusions about the future and help many plan the next move.
The weather forecast is a basic example. With the regression technique, it’s possible to travel back in time to view average temperatures, humidity, and other variables relevant to the results. Then, you “return” to present and tailor predictions about the weather in the future.
There are different types of regression, and each has unique applications, advantages, and drawbacks. This article will analyze these types.
Linear Regression
Linear regression in machine learning is one of the most common techniques. This simple algorithm got its name because of what it does. It digs deep into the relationship between independent and dependent variables. Based on the findings, linear regression makes predictions about the future.
There are two distinguishable types of linear regression:
- Simple linear regression – There’s only one input variable.
- Multiple linear regression – There are several input variables.
Linear regression has proven useful in various spheres. Its most popular applications are:
- Predicting salaries
- Analyzing trends
- Forecasting traffic ETAs
- Predicting real estate prices
Polynomial Regression
At its core, polynomial regression functions just like linear regression, with one crucial difference – the former works with non-linear datasets.
When there’s a non-linear relationship between variables, you can’t do much with linear regression. In such cases, you send polynomial regression to the rescue. You do this by adding polynomial features to linear regression. Then, you analyze these features using a linear model to get relevant results.
Here’s a real-life example in action. Polynomial regression can analyze the spread rate of infectious diseases, including COVID-19.
Ridge Regression
Ridge regression is a type of linear regression. What’s the difference between the two? You use ridge regression when there’s high colinearity between independent variables. In such cases, you have to add bias to ensure precise long-term results.
This type of regression is also called L2 regularization because it makes the model less complex. As such, ridge regression is suitable for solving problems with more parameters than samples. Due to its characteristics, this regression has an honorary spot in medicine. It’s used to analyze patients’ clinical measures and the presence of specific antigens. Based on the results, the regression establishes trends.
LASSO Regression
No, LASSO regression doesn’t have anything to do with cowboys and catching cattle (although that would be interesting). LASSO is actually an acronym for Least Absolute Shrinkage and Selection Operator.
Like ridge regression, this one also belongs to regularization techniques. What does it regulate? It reduces a model’s complexity by eliminating parameters that aren’t relevant, thus concentrating the selection and guaranteeing better results.
Many choose ridge regression when analyzing a model with numerous true coefficients. When there are only a few of them, use LASSO. Therefore, their applications are similar; the real difference lies in the number of available coefficients.
Elastic Net Regression
Ridge regression is good for analyzing problems involving more parameters than samples. However, it’s not perfect; this regression type doesn’t promise to eliminate irrelevant coefficients from the equation, thus affecting the results’ reliability.
On the other hand, LASSO regression eliminates irrelevant parameters, but it sometimes focuses on far too few samples for high-dimensional data.
As you can see, both regressions are flawed in a way. Elastic net regression is the combination of the best characteristics of these regression techniques. The first phase is finding ridge coefficients, while the second phase involves a LASSO-like shrinkage of these coefficients to get the best results.
Support Vector Regression
Support vector machine (SVM) belongs to supervised learning algorithms and has two important uses:
- Regression
- Classification problems
Let’s try to draw a mental picture of how SVM works. Suppose you have two classes of items (let’s call them red circles and green triangles). Red circles are on the left, while green triangles are on the right. You can separate these two classes by drawing a line between them.
Things get a bit more complicated if you have red circles in the middle and green triangles wrapped around them. In that case, you can’t draw a line to separate the classes. But you can add new dimensions to the mix and create a circle (rectangle, square, or a different shape encompassing just the red circles).
This is what SVM does. It creates a hyperplane and analyzes classes depending on where they belong.
There are a few parameters you need to understand to grasp the reach of SVM fully:
- Kernel – When you can’t find a hyperplane in a dimension, you move to a higher dimension, which is often challenging to navigate. A kernel is like a navigator that helps you find the hyperplane without plummeting computational costs.
- Hyperplane – This is what separates two classes in SVM.
- Decision boundary – Think of this as a line that helps you “decide” the placement of positive and negative examples.
Support vector regression takes a similar approach. It also creates a hyperplane to analyze classes but doesn’t classify them depending on where they belong. Instead, it tries to find a hyperplane that contains a maximum number of data points. At the same time, support vector regression tries to lower the risk of prediction errors.
SVM has various applications. It can be used in finance, bioinformatics, engineering, HR, healthcare, image processing, and other branches.
Decision Tree Regression
This type of supervised learning algorithm can solve both regression and classification issues and work with categorical and numerical datasets.
As its name indicates, decision tree regression deconstructs problems by creating a tree-like structure. In this tree, every node is a test for an attribute, every branch is the result of a test, and every leaf is the final result (decision).
The starting point of (the root) of every tree regression is the parent node. This node splits into two child nodes (data subsets), which are then further divided, thus becoming “parents” to their “children,” and so on.
You can compare a decision tree to a regular tree. If you take care of it and prune the unnecessary branches (those with irrelevant features), you’ll grow a healthy tree (a tree with concise and relevant results).
Due to its versatility and digestibility, decision tree regression can be used in various fields, from finance and healthcare to marketing and education. It offers a unique approach to decision-making by breaking down complex datasets into easy-to-grasp categories.
Random Forest Regression
Random forest regression is essentially decision tree regression but on a much bigger scale. In this case, you have multiple decision trees, each predicting a certain output. Random forest regression analyzes the outputs of every decision tree to come up with the final result.
Keep in mind that the decision trees used in random forest regression are completely independent; there’s no interaction between them until their outputs are analyzed.
Random forest regression is an ensemble learning technique, meaning it combines the results (predictions) of several machine learning algorithms to create one final prediction.
Like decision tree regression, this one can be used in numerous industries.
The Importance of Regression in Machine Learning Is Immeasurable
Regression in machine learning is like a high-tech detective. It travels back in time, identifies valuable clues, and analyzes them thoroughly. Then, it uses the results to predict outcomes with high accuracy and precision. As such, regression found its way to all niches.
You can use it in sales to analyze the customers’ behavior and anticipate their future interests. You can also apply it in finance, whether to discover trends in prices or analyze the stock market. Regression is also used in education, the tech industry, weather forecasting, and many other spheres.
Every regression technique can be valuable, but only if you know how to use it to your advantage. Think of your scenario (variables you want to analyze) and find the best actor (regression technique) who can breathe new life into it.
Related posts
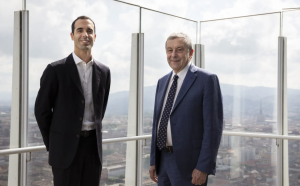
Source:
- EFMD Global, Published on July 12th, 2024.
By Stephanie Mullins
Many people love to read the stories of successful business school graduates to see what they’ve achieved using the lessons, insights and connections from the programmes they’ve studied. We speak to one alumnus, Riccardo Ocleppo, who studied at top business schools including London Business School (LBS) and INSEAD, about the education institution called OPIT which he created after business school.
Please introduce yourself and your career to date.
I am the founder of OPIT — Open Institute of Technology, a fully accredited Higher Education Institution (HEI) under the European Qualification Framework (EQF) by the MFHEA Authority. OPIT also partners with WES (World Education Services), a trusted non-profit providing verified education credential assessments (ECA) in the US and Canada for foreign degrees and certificates.
Prior to founding OPIT, I established Docsity, a global community boasting 15 million registered university students worldwide and partnerships with over 250 Universities and Business Schools. My academic background includes an MSc in Electronics from Politecnico di Torino and an MSc in Management from London Business School.
Why did you decide to create OPIT Open Institute of Technology?
Higher education has a profound impact on people’s futures. Through quality higher education, people can aspire to a better and more fulfilling future.
The mission behind OPIT is to democratise access to high-quality higher education in the fields that will be in high demand in the coming decades: Computer Science, Artificial Intelligence, Data Science, Cybersecurity, and Digital Innovation.
Since launching my first company in the education field, I’ve engaged with countless students, partnered with hundreds of universities, and collaborated with professors and companies. Through these interactions, I’ve observed a gap between traditional university curricula and the skills demanded by today’s job market, particularly in Computer Science and Technology.
I founded OPIT to bridge this gap by modernising education, making it affordable, and enhancing the digital learning experience. By collaborating with international professors and forging solid relationships with global companies, we are creating a dynamic online community and developing high-quality digital learning content. This approach ensures our students benefit from a flexible, cutting-edge, and stress-free learning environment.
Why do you think an education in tech is relevant in today’s business landscape?
As depicted by the World Economic Forum’s “Future of Jobs 2023” report, the demand for skilled tech professionals remains (and will remain) robust across industries, driven by the critical role of advanced technologies in business success.
Today’s companies require individuals who can innovate and execute complex solutions. A degree in fields like computer science, cybersecurity, data science, digital business or AI equips graduates with essential skills to thrive in this dynamic industry.
According to the International Monetary Fund (IMF), the global tech talent shortage will exceed 85 million workers by 2030. The Korn Ferry Institute warns that this gap could result in hundreds of billions in lost revenue across the US, Europe, and Asia.
To address this challenge, OPIT aims to democratise access to technology education. Our competency-based and applied approach, coupled with a flexible online learning experience, empowers students to progress at their own pace, demonstrating their skills as they advance.
Read the full article below:
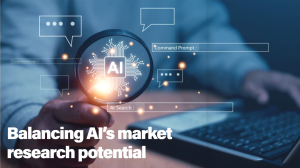
Source:
- The European, Summer 2024 Edition, Page 24
With careful planning, ethical considerations, and ensuring human oversight is maintained, AI can have huge market research benefits, says Lorenzo Livi of the Open Institute of Technology.
By Lorenzo Livi
To market well, you need to get something interesting in front of those who are interested. That takes a lot of thinking, a lot of work, and a whole bunch of research. But what if the bulk of that thinking, work and research could be done for you? What would that mean for marketing as an industry, and market research specifically?
With the recent explosion of AI onto the world stage, big changes are coming in the marketing industry. But will AI be able to do market research as successfully? Simply, the answer is yes. A big, fat, resounding yes. In fact, AI has the potential to revolutionise market research.
Ensuring that people have a clear understanding of what exactly AI is is crucial, given its seismic effect on our world. Common questions that even occur amongst people at the forefront of marketing, such as, “Who invented AI?” or, “Where is the main AI system located?” highlight a widespread misunderstanding about the nature of AI.
As for the notion of a central “main thing” running AI, it’s essential to clarify that AI systems exist in various forms and locations. AI algorithms and models can run on individual computers, servers, or even specialized hardware designed for AI processing, commonly referred to as AI chips. These systems can be distributed across multiple locations, including data centres, cloud platforms, and edge devices. They can also be used anywhere, so long as you have a compatible device and an internet connection.
While the concept of AI may seem abstract or mysterious to some, it’s important to approach it with a clear understanding of its principles and applications. By promoting education and awareness about AI, we can dispel misconceptions and facilitate meaningful conversations about its role in society.
Read the full article below:
- The European, Pages 24 to 26.
Have questions?
Visit our FAQ page or get in touch with us!
Write us at +39 335 576 0263
Get in touch at hello@opit.com
Talk to one of our Study Advisors
We are international
We can speak in: