Search inside The Magazine
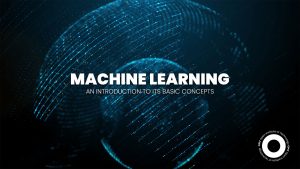
Have you ever played chess or checkers against a computer? If you have, news flash – you’ve watched artificial intelligence at work. But what if the computer could get better at the game on its own just by playing more and analyzing its mistakes? That’s the power of machine learning, a type of AI that lets computers learn and improve from experience.
In fact, machine learning is becoming increasingly important in our daily lives. According to a report by Statista, revenues from the global market for AI software are expected to reach 126 billion by 2025, up from just 10.1 billion in 2018. From personalized recommendations on Netflix to self-driving cars, machine learning is powering some of the most innovative and exciting technologies of our time.
But how does it all work? In this article, we’ll dive into the concepts of machine learning and explore how it’s changing the way we interact with technology.
What is Machine Learning?
Machine learning is a subset of artificial intelligence (AI) that focuses on building algorithms that can learn from data and then make predictions or decisions and recognize patterns. Essentially, it’s all about creating computer programs that can adapt and improve on their own without being explicitly programmed for every possible scenario.
It’s like teaching a computer to see the world through a different lens. From the data, the machine identifies patterns and relationships within it. Based on these patterns, the algorithm can make predictions or decisions about new data it hasn’t seen before.
Because of these qualities, machine learning has plenty of practical applications. We can train computers to make decisions, recognize speech, and even generate art. We can use it in fraud detection in financial transactions or to improve healthcare outcomes through personalized medicine.
Machine learning also plays a large role in fields like computer vision, natural language processing, and robotics, as they require the ability to recognize patterns and make predictions to complete various tasks.
Concepts of Machine Learning
Machine learning might seem magical, but the concepts of machine learning are complex, with many layers of algorithms and techniques working together to get to an end goal.
From supervised and unsupervised learning to deep neural networks and reinforcement learning, there are many base concepts to understand before diving into the world of machine learning. Get ready to explore some machine learning basics!
Supervised Learning
Supervised learning involves training the algorithm to recognize patterns or make predictions using labeled data.
- Classification: Classification is quite straightforward, evident by its name. Its goal is to predict which category or class new data belongs to based on existing data.
- Logistic Regression: Logistic regression aims to predict a binary outcome (i.e., yes or no) based on one or more input variables.
- Support Vector Machines: Support Vector Machines (SVMs) find the best way to separate data points into different categories or classes based on their features or attributes.
- Decision Trees: Decision trees make decisions by dividing data into smaller and smaller subsets from a number of binary decisions. You can think of it like a game of 20 questions where you’re narrowing things down.
- Naive Bayes: Naive Bayes uses Bayes’ theorem to predict how likely it is to end up with a certain result when different input variables are present or absent.
Regression
Regression is a type of machine learning that helps us predict numerical values, like prices or temperatures, based on other data that we have. It looks for patterns in the data to create a mathematical model that can estimate the value we are looking for.
- Linear Regression: Linear regression helps us predict numerical values by fitting a straight line to the data.
- Polynomial Regression: Polynomial regression is similar to linear regression, but instead of fitting a straight line to the data, it fits a curved line (a polynomial) to capture more complex relationships between the variables. Linear regression might be used to predict someone’s salary based on their years of experience, while polynomial regression could be used to predict how fast a car will go based on its engine size.
- Support Vector Regression: Support vector regression finds the best fitting line to the data while minimizing errors and avoiding overfitting (becoming too attuned to the existing data).
- Decision Tree Regression: Decision tree regression uses a tree-like template to make predictions out of a series of decision rules, where each branch represents a decision, and each leaf node represents a prediction.
Unsupervised Learning
Unsupervised learning is where the computer algorithm is given a bunch of data with no labels and has to find patterns or groupings on its own, allowing for discovering hidden insights and relationships.
- Clustering: Clustering groups similar data points together based on their features.
- K-Means: K-Means is a popular clustering algorithm that separates the data into a predetermined number of clusters by finding the average of each group.
- Hierarchical Clustering: Hierarchical clustering is another way of grouping that creates a hierarchy of clusters by either merging smaller clusters into larger ones (agglomerative) or dividing larger clusters into smaller ones (divisive).
- Expectation Maximization: Expectation maximization is quite self-explanatory. It’s a way to find patterns in data that aren’t clearly grouped together by guessing what might be there and refining the guesses over time.
- Association Rule Learning: Association Rule Learning looks to find interesting connections between things in large sets of data, like discovering that people who buy plant pots often also buy juice.
- Apriori: Apriori is an algorithm for association rule learning that finds frequent itemsets (groups of items that appear together often) and makes rules that describe the relationships between them.
- Eclat: Eclat is similar to apriori, but it works by first finding which things appear together most often and then finding frequent itemsets out of those. It’s a method that works better for larger datasets.
Reinforcement Learning
Reinforcement learning is like teaching a computer to play a game by letting it try different actions and rewarding it when it does something good so it learns how to maximize its score over time.
- Q-Learning: Q-Learning helps computers learn how to take actions in an environment by assigning values to each possible action and using those values to make decisions.
- SARSA: SARSA is similar to Q-Learning but takes into account the current state of the environment, making it more useful in situations where actions have immediate consequences.
- DDPG (Deep Deterministic Policy Gradient): DDPG is a more advanced type of reinforcement learning that uses neural networks to learn policies for continuous control tasks, like robotic movement, by mapping what it sees to its next action.
Deep Learning Algorithms
Deep Learning is a powerful type of machine learning that’s inspired by how the human brain works, using artificial neural networks to learn and make decisions from vast amounts of data.
It’s more complex than other types of machine learning because it involves many layers of connections that can learn to recognize complex patterns and relationships in data.
- Neural Networks: Neural networks mimic the structure and function of the human brain, allowing them to learn from and make predictions about complex data.
- Convolutional Neural Networks: Convolutional neural networks are particularly good at image recognition, using specialized layers to detect features like edges, textures, and shapes.
- Recurrent Neural Networks: Recurrent neural networks are known to be good at processing sequential data, like language or music, by keeping track of previous inputs and using that information to make better predictions.
- Generative Adversarial Networks: Generative adversarial networks can generate new, original data by pitting two networks against each other. One tries to create fake data, and the other tries to spot the fakes until the generator network gets really good at making convincing fakes.
Conclusion
As we’ve learned, machine learning is a powerful tool that can help computers learn from data and make predictions, recognize patterns, and even create new things.
With basic concepts like supervised and unsupervised learning, regression and clustering, and advanced techniques like deep learning and neural networks, the possibilities for what we can achieve with machine learning are endless.
So whether you’re new to the subject or deeper down the iceberg, there’s always something new to learn in the exciting field of machine learning!
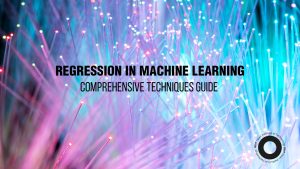
As artificial intelligence and machine learning are becoming present in almost every aspect of life, it’s essential to understand how they work and their common applications. Although machine learning has been around for a while, many still portray it as an enemy. Machine learning can be your friend, but only if you learn to “tame” it.
Regression stands out as one of the most popular machine-learning techniques. It serves as a bridge that connects the past to the present and future. It does so by picking up on different “events” from the past and breaking them apart to analyze them. Based on this analysis, regression can make conclusions about the future and help many plan the next move.
The weather forecast is a basic example. With the regression technique, it’s possible to travel back in time to view average temperatures, humidity, and other variables relevant to the results. Then, you “return” to present and tailor predictions about the weather in the future.
There are different types of regression, and each has unique applications, advantages, and drawbacks. This article will analyze these types.
Linear Regression
Linear regression in machine learning is one of the most common techniques. This simple algorithm got its name because of what it does. It digs deep into the relationship between independent and dependent variables. Based on the findings, linear regression makes predictions about the future.
There are two distinguishable types of linear regression:
- Simple linear regression – There’s only one input variable.
- Multiple linear regression – There are several input variables.
Linear regression has proven useful in various spheres. Its most popular applications are:
- Predicting salaries
- Analyzing trends
- Forecasting traffic ETAs
- Predicting real estate prices
Polynomial Regression
At its core, polynomial regression functions just like linear regression, with one crucial difference – the former works with non-linear datasets.
When there’s a non-linear relationship between variables, you can’t do much with linear regression. In such cases, you send polynomial regression to the rescue. You do this by adding polynomial features to linear regression. Then, you analyze these features using a linear model to get relevant results.
Here’s a real-life example in action. Polynomial regression can analyze the spread rate of infectious diseases, including COVID-19.
Ridge Regression
Ridge regression is a type of linear regression. What’s the difference between the two? You use ridge regression when there’s high colinearity between independent variables. In such cases, you have to add bias to ensure precise long-term results.
This type of regression is also called L2 regularization because it makes the model less complex. As such, ridge regression is suitable for solving problems with more parameters than samples. Due to its characteristics, this regression has an honorary spot in medicine. It’s used to analyze patients’ clinical measures and the presence of specific antigens. Based on the results, the regression establishes trends.
LASSO Regression
No, LASSO regression doesn’t have anything to do with cowboys and catching cattle (although that would be interesting). LASSO is actually an acronym for Least Absolute Shrinkage and Selection Operator.
Like ridge regression, this one also belongs to regularization techniques. What does it regulate? It reduces a model’s complexity by eliminating parameters that aren’t relevant, thus concentrating the selection and guaranteeing better results.
Many choose ridge regression when analyzing a model with numerous true coefficients. When there are only a few of them, use LASSO. Therefore, their applications are similar; the real difference lies in the number of available coefficients.
Elastic Net Regression
Ridge regression is good for analyzing problems involving more parameters than samples. However, it’s not perfect; this regression type doesn’t promise to eliminate irrelevant coefficients from the equation, thus affecting the results’ reliability.
On the other hand, LASSO regression eliminates irrelevant parameters, but it sometimes focuses on far too few samples for high-dimensional data.
As you can see, both regressions are flawed in a way. Elastic net regression is the combination of the best characteristics of these regression techniques. The first phase is finding ridge coefficients, while the second phase involves a LASSO-like shrinkage of these coefficients to get the best results.
Support Vector Regression
Support vector machine (SVM) belongs to supervised learning algorithms and has two important uses:
- Regression
- Classification problems
Let’s try to draw a mental picture of how SVM works. Suppose you have two classes of items (let’s call them red circles and green triangles). Red circles are on the left, while green triangles are on the right. You can separate these two classes by drawing a line between them.
Things get a bit more complicated if you have red circles in the middle and green triangles wrapped around them. In that case, you can’t draw a line to separate the classes. But you can add new dimensions to the mix and create a circle (rectangle, square, or a different shape encompassing just the red circles).
This is what SVM does. It creates a hyperplane and analyzes classes depending on where they belong.
There are a few parameters you need to understand to grasp the reach of SVM fully:
- Kernel – When you can’t find a hyperplane in a dimension, you move to a higher dimension, which is often challenging to navigate. A kernel is like a navigator that helps you find the hyperplane without plummeting computational costs.
- Hyperplane – This is what separates two classes in SVM.
- Decision boundary – Think of this as a line that helps you “decide” the placement of positive and negative examples.
Support vector regression takes a similar approach. It also creates a hyperplane to analyze classes but doesn’t classify them depending on where they belong. Instead, it tries to find a hyperplane that contains a maximum number of data points. At the same time, support vector regression tries to lower the risk of prediction errors.
SVM has various applications. It can be used in finance, bioinformatics, engineering, HR, healthcare, image processing, and other branches.
Decision Tree Regression
This type of supervised learning algorithm can solve both regression and classification issues and work with categorical and numerical datasets.
As its name indicates, decision tree regression deconstructs problems by creating a tree-like structure. In this tree, every node is a test for an attribute, every branch is the result of a test, and every leaf is the final result (decision).
The starting point of (the root) of every tree regression is the parent node. This node splits into two child nodes (data subsets), which are then further divided, thus becoming “parents” to their “children,” and so on.
You can compare a decision tree to a regular tree. If you take care of it and prune the unnecessary branches (those with irrelevant features), you’ll grow a healthy tree (a tree with concise and relevant results).
Due to its versatility and digestibility, decision tree regression can be used in various fields, from finance and healthcare to marketing and education. It offers a unique approach to decision-making by breaking down complex datasets into easy-to-grasp categories.
Random Forest Regression
Random forest regression is essentially decision tree regression but on a much bigger scale. In this case, you have multiple decision trees, each predicting a certain output. Random forest regression analyzes the outputs of every decision tree to come up with the final result.
Keep in mind that the decision trees used in random forest regression are completely independent; there’s no interaction between them until their outputs are analyzed.
Random forest regression is an ensemble learning technique, meaning it combines the results (predictions) of several machine learning algorithms to create one final prediction.
Like decision tree regression, this one can be used in numerous industries.
The Importance of Regression in Machine Learning Is Immeasurable
Regression in machine learning is like a high-tech detective. It travels back in time, identifies valuable clues, and analyzes them thoroughly. Then, it uses the results to predict outcomes with high accuracy and precision. As such, regression found its way to all niches.
You can use it in sales to analyze the customers’ behavior and anticipate their future interests. You can also apply it in finance, whether to discover trends in prices or analyze the stock market. Regression is also used in education, the tech industry, weather forecasting, and many other spheres.
Every regression technique can be valuable, but only if you know how to use it to your advantage. Think of your scenario (variables you want to analyze) and find the best actor (regression technique) who can breathe new life into it.
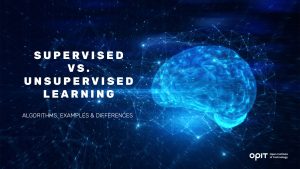
The human brain is among the most complicated organs and one of nature’s most amazing creations. The brain’s capacity is considered limitless; there isn’t a thing it can’t remember. Although many often don’t think about it, the processes that happen in the mind are fascinating.
As technology evolved over the years, scientists figured out a way to make machines think like humans, and this process is called machine learning. Like cars need fuel to operate, machines need data and algorithms. With the application of adequate techniques, machines can learn from this data and even improve their accuracy as time passes.
Two basic machine learning approaches are supervised and unsupervised learning. You can already assume the biggest difference between them based on their names. With supervised learning, you have a “teacher” who shows the machine how to analyze specific data. Unsupervised learning is completely independent, meaning there are no teachers or guides.
This article will talk more about supervised and unsupervised learning, outline their differences, and introduce examples.
Supervised Learning
Imagine a teacher trying to teach their young students to write the letter “A.” The teacher will first set an example by writing the letter on the board, and the students will follow. After some time, the students will be able to write the letter without assistance.
Supervised machine learning is very similar to this situation. In this case, you (the teacher) train the machine using labeled data. Such data already contains the right answer to a particular situation. The machine then uses this training data to learn a pattern and applies it to all new datasets.
Note that the role of a teacher is essential. The provided labeled datasets are the foundation of the machine’s learning process. If you withhold these datasets or don’t label them correctly, you won’t get any (relevant) results.
Supervised learning is complex, but we can understand it through a simple real-life example.
Suppose you have a basket filled with red apples, strawberries, and pears and want to train a machine to identify these fruits. You’ll teach the machine the basic characteristics of each fruit found in the basket, focusing on the color, size, shape, and other relevant features. If you introduce a “new” strawberry to the basket, the machine will analyze its appearance and label it as “strawberry” based on the knowledge it acquired during training.
Types of Supervised Learning
You can divide supervised learning into two types:
- Classification – You can train machines to classify data into categories based on different characteristics. The fruit basket example is the perfect representation of this scenario.
- Regression – You can train machines to use specific data to make future predictions and identify trends.
Supervised Learning Algorithms
Supervised learning uses different algorithms to function:
- Linear regression – It identifies a linear relationship between an independent and a dependent variable.
- Logistic regression – It typically predicts binary outcomes (yes/no, true/false) and is important for classification purposes.
- Support vector machines – They use high-dimensional features to map data that can’t be separated by a linear line.
- Decision trees – They predict outcomes and classify data using tree-like structures.
- Random forests – They analyze several decision trees to come up with a unique prediction/result.
- Neural networks – They process data in a unique way, very similar to the human brain.
Supervised Learning: Examples and Applications
There’s no better way to understand supervised learning than through examples. Let’s dive into the real estate world.
Suppose you’re a real estate agent and need to predict the prices of different properties in your city. The first thing you’ll need to do is feed your machine existing data about available houses in the area. Factors like square footage, amenities, a backyard/garden, the number of rooms, and available furniture, are all relevant factors. Then, you need to “teach” the machine the prices of different properties. The more, the better.
A large dataset will help your machine pick up on seemingly minor but significant trends affecting the price. Once your machine processes this data and you introduce a new property to it, it will be able to cross-reference its features with the existing database and come up with an accurate price prediction.
The applications of supervised learning are vast. Here are the most popular ones:
- Sales – Predicting customers’ purchasing behavior and trends
- Finance – Predicting stock market fluctuations, price changes, expenses, etc.
- Healthcare – Predicting risk of diseases and infections, surgery outcomes, necessary medications, etc.
- Weather forecasts – Predicting temperature, humidity, atmospheric pressure, wind speed, etc.
- Face recognition – Identifying people in photos
Unsupervised Learning
Imagine a family with a baby and a dog. The dog lives inside the house, so the baby is used to it and expresses positive emotions toward it. A month later, a friend comes to visit, and they bring their dog. The baby hasn’t seen the dog before, but she starts smiling as soon as she sees it.
Why?
Because the baby was able to draw her own conclusions based on the new dog’s appearance: two ears, tail, nose, tongue sticking out, and maybe even a specific noise (barking). Since the baby has positive emotions toward the house dog, she also reacts positively to a new, unknown dog.
This is a real-life example of unsupervised learning. Nobody taught the baby about dogs, but she still managed to make accurate conclusions.
With supervised machine learning, you have a teacher who trains the machine. This isn’t the case with unsupervised learning. Here, it’s necessary to give the machine freedom to explore and discover information. Therefore, this machine learning approach deals with unlabeled data.
Types of Unsupervised Learning
There are two types of unsupervised learning:
- Clustering – Grouping uncategorized data based on their common features.
- Dimensionality reduction – Reducing the number of variables, features, or columns to capture the essence of the available information.
Unsupervised Learning Algorithms
Unsupervised learning relies on these algorithms:
- K-means clustering – It identifies similar features and groups them into clusters.
- Hierarchical clustering – It identifies similarities and differences between data and groups them hierarchically.
- Principal component analysis (PCA) – It reduces data dimensionality while boosting interpretability.
- Independent component analysis (ICA) – It separates independent sources from mixed signals.
- T-distributed stochastic neighbor embedding (t-SNE) – It explores and visualizes high-dimensional data.
Unsupervised Learning: Examples and Applications
Let’s see how unsupervised learning is used in customer segmentation.
Suppose you work for a company that wants to learn more about its customers to build more effective marketing campaigns and sell more products. You can use unsupervised machine learning to analyze characteristics like gender, age, education, location, and income. This approach is able to discover who purchases your products more often. After getting the results, you can come up with strategies to push the product more.
Unsupervised learning is often used in the same industries as supervised learning but with different purposes. For example, both approaches are used in sales. Supervised learning can accurately predict prices relying on past data. On the other hand, unsupervised learning analyzes the customers’ behaviors. The combination of the two approaches results in a quality marketing strategy that can attract more buyers and boost sales.
Another example is traffic. Supervised learning can provide an ETA to a destination, while unsupervised learning digs a bit deeper and often looks at the bigger picture. It can analyze a specific area to pinpoint accident-prone locations.
Differences Between Supervised and Unsupervised Learning
These are the crucial differences between the two machine learning approaches:
- Data labeling – Supervised learning uses labeled datasets, while unsupervised learning uses unlabeled, “raw” data. In other words, the former requires training, while the latter works independently to discover information.
- Algorithm complexity – Unsupervised learning requires more complex algorithms and powerful tools that can handle vast amounts of data. This is both a drawback and an advantage. Since it operates on complex algorithms, it’s capable of handling larger, more complicated datasets, which isn’t a characteristic of supervised learning.
- Use cases and applications – The two approaches can be used in the same industries but with different purposes. For example, supervised learning is used in predicting prices, while unsupervised learning is used in detecting customers’ behavior or anomalies.
- Evaluation metrics – Supervised learning tends to be more accurate (at least for now). Machines still require a bit of our input to display accurate results.
Choose Wisely
Do you need to teach your machine different data, or can you trust it to handle the analysis on its own? Think about what you want to analyze. Unsupervised and supervised learning may sound similar, but they have different uses. Choosing an inadequate approach leads to unreliable, irrelevant results.
Supervised learning is still more popular than unsupervised learning because it offers more accurate results. However, this approach can’t handle larger, complex datasets and requires human intervention, which isn’t the case with unsupervised learning. Therefore, we may see a rise in the popularity of the unsupervised approach, especially as the technology evolves and enables more accuracy.
Have questions?
Visit our FAQ page or get in touch with us!
Write us at +39 335 576 0263
Get in touch at hello@opit.com
Talk to one of our Study Advisors
We are international
We can speak in: