AI investment has become a must in the business world, and companies from all over the globe are embracing this trend. Nearly 90% of organizations plan to put more money into AI by 2025.
One of the main areas of investment is deep learning. The World Economic Forum approves of this initiative, as the cutting-edge technology can boost productivity, optimize cybersecurity, and enhance decision-making.
Knowing that deep learning is making waves is great, but it doesn’t mean much if you don’t understand the basics. Read on for deep learning applications and the most common examples.
Artificial Neural Networks
Once you scratch the surface of deep learning, you’ll see that it’s underpinned by artificial neural networks. That’s why many people refer to deep learning as deep neural networking and deep neural learning.
There are different types of artificial neural networks.
Perceptron
Perceptrons are the most basic form of neural networks. These artificial neurons were originally used for calculating business intelligence or input data capabilities. Nowadays, it’s a linear algorithm that supervises the learning of binary classifiers.
Convolutional Neural Networks
Convolutional neural network machine learning is another common type of deep learning network. It combines input data with learned features before allowing this architecture to analyze images or other 2D data.
The most significant benefit of convolutional neural networks is that they automate feature extraction. As a result, you don’t have to recognize features on your own when classifying pictures or other visuals – the networks extract them directly from the source.
Recurrent Neural Networks
Recurrent neural networks use time series or sequential information. You can find them in many areas, such as natural language processing, image captioning, and language translation. Google Translate, Siri, and many other applications have adopted this technology.
Generative Adversarial Networks
Generative adversarial networks are architecture with two sub-types. The generator model produces new examples, whereas the discriminated model determines if the examples generated are real or fake.
These networks work like so-called game theory scenarios, where generator networks come face-to-face with their adversaries. They generate examples directly, while the adversary (discriminator) tries to tell the difference between these examples and those obtained from training information.
Deep Learning Applications
Deep learning helps take a multitude of technologies to a whole new level.
Computer Vision
The feature that allows computers to obtain useful data from videos and pictures is known as computer vision. An already sophisticated process, deep learning can enhance the technology further.
For instance, you can utilize deep learning to enable machines to understand visuals like humans. They can be trained to automatically filter adult content to make it child-friendly. Likewise, deep learning can enable computers to recognize critical image information, such as logos and food brands.
Natural Language Processing
Artificial intelligence deep learning algorithms spearhead the development and optimization of natural language processing. They automate various processes and platforms, including virtual agents, the analysis of business documents, key phrase indexing, and article summarization.
Speech Recognition
Human speech differs greatly in language, accent, tone, and other key characteristics. This doesn’t stop deep learning from polishing speech recognition software. For instance, Siri is a deep learning-based virtual assistant that can automatically make and recognize calls. Other deep learning programs can transcribe meeting recordings and translate movies to reach wider audiences.
Robotics
Robots are invented to simplify certain tasks (i.e., reduce human input). Deep learning models are perfect for this purpose, as they help manufacturers build advanced robots that replicate human activity. These machines receive timely updates to plan their movements and overcome any obstacles on their way. That’s why they’re common in warehouses, healthcare centers, and manufacturing facilities.
Some of the most famous deep learning-enabled robots are those produced by Boston Dynamics. For example, their robot Atlas is highly agile due to its deep learning architecture. It can move seamlessly and perform dynamic interactions that are common in people.
Autonomous Driving
Self-driving cars are all the rage these days. The autonomous driving industry is expected to generate over $300 billion in revenue by 2035, and most of the credits will go to deep learning.
The producers of these vehicles use deep learning to train cars to respond to real-life traffic scenarios and improve safety. They incorporate different technologies that allow cars to calculate the distance to the nearest objects and navigate crowded streets. The vehicles come with ultra-sensitive cameras and sensors, all of which are powered by deep learning.
Passengers aren’t the only group who will benefit from deep learning-supported self-driving cars. The technology is expected to revolutionize emergency and food delivery services as well.
Deep Learning Algorithms
Numerous deep learning algorithms power the above technologies. Here are the four most common examples.
Backpropagation
Backpropagation is commonly used in neural network training. It starts from so-called “forward propagation,” analyzing its error rate. It feeds the error backward through various network layers, allowing you to optimize the weights (parameters that transform input data within hidden layers).
Stochastic Gradient Descent
The primary purpose of the stochastic gradient descent algorithm is to locate the parameters that allow other machine learning algorithms to operate at their peak efficiency. It’s generally combined with other algorithms, such as backpropagation, to enhance neural network training.
Reinforcement Learning
The reinforcement learning algorithm is trained to resolve multi-layer problems. It experiments with different solutions until it finds the right one. This method draws its decisions from real-life situations.
The reason it’s called reinforcement learning is that it operates on a reward/penalty basis. It aims to maximize rewards to reinforce further training.
Transfer Learning
Transfer learning boils down to recycling pre-configured models to solve new issues. The algorithm uses previously obtained knowledge to make generalizations when facing another problem.
For instance, many deep learning experts use transfer learning to train the system to recognize images. A classifier can use this algorithm to identify pictures of trucks if it’s already analyzed car photos.
Deep Learning Tools
Deep learning tools are platforms that enable you to develop software that lets machines mimic human activity by processing information carefully before making a decision. You can choose from a wide range of such tools.
TensorFlow
Developed in CUDA and C++, TensorFlow is a highly advanced deep learning tool. Google launched this open-source solution to facilitate various deep learning platforms.
Despite being advanced, it can also be used by beginners due to its relatively straightforward interface. It’s perfect for creating cloud, desktop, and mobile machine learning models.
Keras
The Keras API is a Python-based tool with several features for solving machine learning issues. It works with TensorFlow, Thenao, and other tools to optimize your deep learning environment and create robust models.
In most cases, prototyping with Keras is fast and scalable. The API is compatible with convolutional and recurrent networks.
PyTorch
PyTorch is another Python-based tool. It’s also a machine learning library and scripting language that allows you to create neural networks through sophisticated algorithms. You can use the tool on virtually any cloud software, and it delivers distributed training to speed up peer-to-peer updates.
Caffe
Caffe’s framework was launched by Berkeley as an open-source platform. It features an expressive design, which is perfect for propagating cutting-edge applications. Startups, academic institutions, and industries are just some environments where this tool is common.
Theano
Python makes yet another appearance in deep learning tools. Here, it powers Theano, enabling the tool to assess complex mathematical tasks. The software can solve issues that require tremendous computing power and vast quantities of information.
Deep Learning Examples
Deep learning is the go-to solution for creating and maintaining the following technologies.
Image Recognition
Image recognition programs are systems that can recognize specific items, people, or activities in digital photos. Deep learning is the method that enables this functionality. The most well-known example of the use of deep learning for image recognition is in healthcare settings. Radiologists and other professionals can rely on it to analyze and evaluate large numbers of images faster.
Text Generation
There are several subtypes of natural language processing, including text generation. Underpinned by deep learning, it leverages AI to produce different text forms. Examples include machine translations and automatic summarizations.
Self-Driving Cars
As previously mentioned, deep learning is largely responsible for the development of self-driving cars. AutoX might be the most renowned manufacturer of these vehicles.
The Future Lies in Deep Learning
Many up-and-coming technologies will be based on deep learning AI. It’s no surprise, therefore, that nearly 50% of enterprises already use deep learning as the driving force of their products and services. If you want to expand your knowledge about this topic, consider taking a deep learning course. You’ll improve your employment opportunities and further demystify the concept.
Related posts
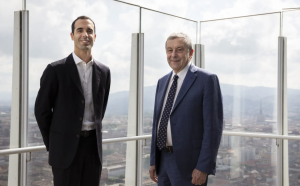
Source:
- EFMD Global, Published on July 12th, 2024.
By Stephanie Mullins
Many people love to read the stories of successful business school graduates to see what they’ve achieved using the lessons, insights and connections from the programmes they’ve studied. We speak to one alumnus, Riccardo Ocleppo, who studied at top business schools including London Business School (LBS) and INSEAD, about the education institution called OPIT which he created after business school.
Please introduce yourself and your career to date.
I am the founder of OPIT — Open Institute of Technology, a fully accredited Higher Education Institution (HEI) under the European Qualification Framework (EQF) by the MFHEA Authority. OPIT also partners with WES (World Education Services), a trusted non-profit providing verified education credential assessments (ECA) in the US and Canada for foreign degrees and certificates.
Prior to founding OPIT, I established Docsity, a global community boasting 15 million registered university students worldwide and partnerships with over 250 Universities and Business Schools. My academic background includes an MSc in Electronics from Politecnico di Torino and an MSc in Management from London Business School.
Why did you decide to create OPIT Open Institute of Technology?
Higher education has a profound impact on people’s futures. Through quality higher education, people can aspire to a better and more fulfilling future.
The mission behind OPIT is to democratise access to high-quality higher education in the fields that will be in high demand in the coming decades: Computer Science, Artificial Intelligence, Data Science, Cybersecurity, and Digital Innovation.
Since launching my first company in the education field, I’ve engaged with countless students, partnered with hundreds of universities, and collaborated with professors and companies. Through these interactions, I’ve observed a gap between traditional university curricula and the skills demanded by today’s job market, particularly in Computer Science and Technology.
I founded OPIT to bridge this gap by modernising education, making it affordable, and enhancing the digital learning experience. By collaborating with international professors and forging solid relationships with global companies, we are creating a dynamic online community and developing high-quality digital learning content. This approach ensures our students benefit from a flexible, cutting-edge, and stress-free learning environment.
Why do you think an education in tech is relevant in today’s business landscape?
As depicted by the World Economic Forum’s “Future of Jobs 2023” report, the demand for skilled tech professionals remains (and will remain) robust across industries, driven by the critical role of advanced technologies in business success.
Today’s companies require individuals who can innovate and execute complex solutions. A degree in fields like computer science, cybersecurity, data science, digital business or AI equips graduates with essential skills to thrive in this dynamic industry.
According to the International Monetary Fund (IMF), the global tech talent shortage will exceed 85 million workers by 2030. The Korn Ferry Institute warns that this gap could result in hundreds of billions in lost revenue across the US, Europe, and Asia.
To address this challenge, OPIT aims to democratise access to technology education. Our competency-based and applied approach, coupled with a flexible online learning experience, empowers students to progress at their own pace, demonstrating their skills as they advance.
Read the full article below:
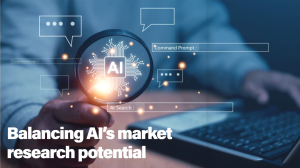
Source:
- The European, Summer 2024 Edition, Page 24
With careful planning, ethical considerations, and ensuring human oversight is maintained, AI can have huge market research benefits, says Lorenzo Livi of the Open Institute of Technology.
By Lorenzo Livi
To market well, you need to get something interesting in front of those who are interested. That takes a lot of thinking, a lot of work, and a whole bunch of research. But what if the bulk of that thinking, work and research could be done for you? What would that mean for marketing as an industry, and market research specifically?
With the recent explosion of AI onto the world stage, big changes are coming in the marketing industry. But will AI be able to do market research as successfully? Simply, the answer is yes. A big, fat, resounding yes. In fact, AI has the potential to revolutionise market research.
Ensuring that people have a clear understanding of what exactly AI is is crucial, given its seismic effect on our world. Common questions that even occur amongst people at the forefront of marketing, such as, “Who invented AI?” or, “Where is the main AI system located?” highlight a widespread misunderstanding about the nature of AI.
As for the notion of a central “main thing” running AI, it’s essential to clarify that AI systems exist in various forms and locations. AI algorithms and models can run on individual computers, servers, or even specialized hardware designed for AI processing, commonly referred to as AI chips. These systems can be distributed across multiple locations, including data centres, cloud platforms, and edge devices. They can also be used anywhere, so long as you have a compatible device and an internet connection.
While the concept of AI may seem abstract or mysterious to some, it’s important to approach it with a clear understanding of its principles and applications. By promoting education and awareness about AI, we can dispel misconceptions and facilitate meaningful conversations about its role in society.
Read the full article below:
- The European, Pages 24 to 26.
Have questions?
Visit our FAQ page or get in touch with us!
Write us at +39 335 576 0263
Get in touch at hello@opit.com
Talk to one of our Study Advisors
We are international
We can speak in: