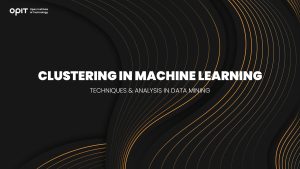
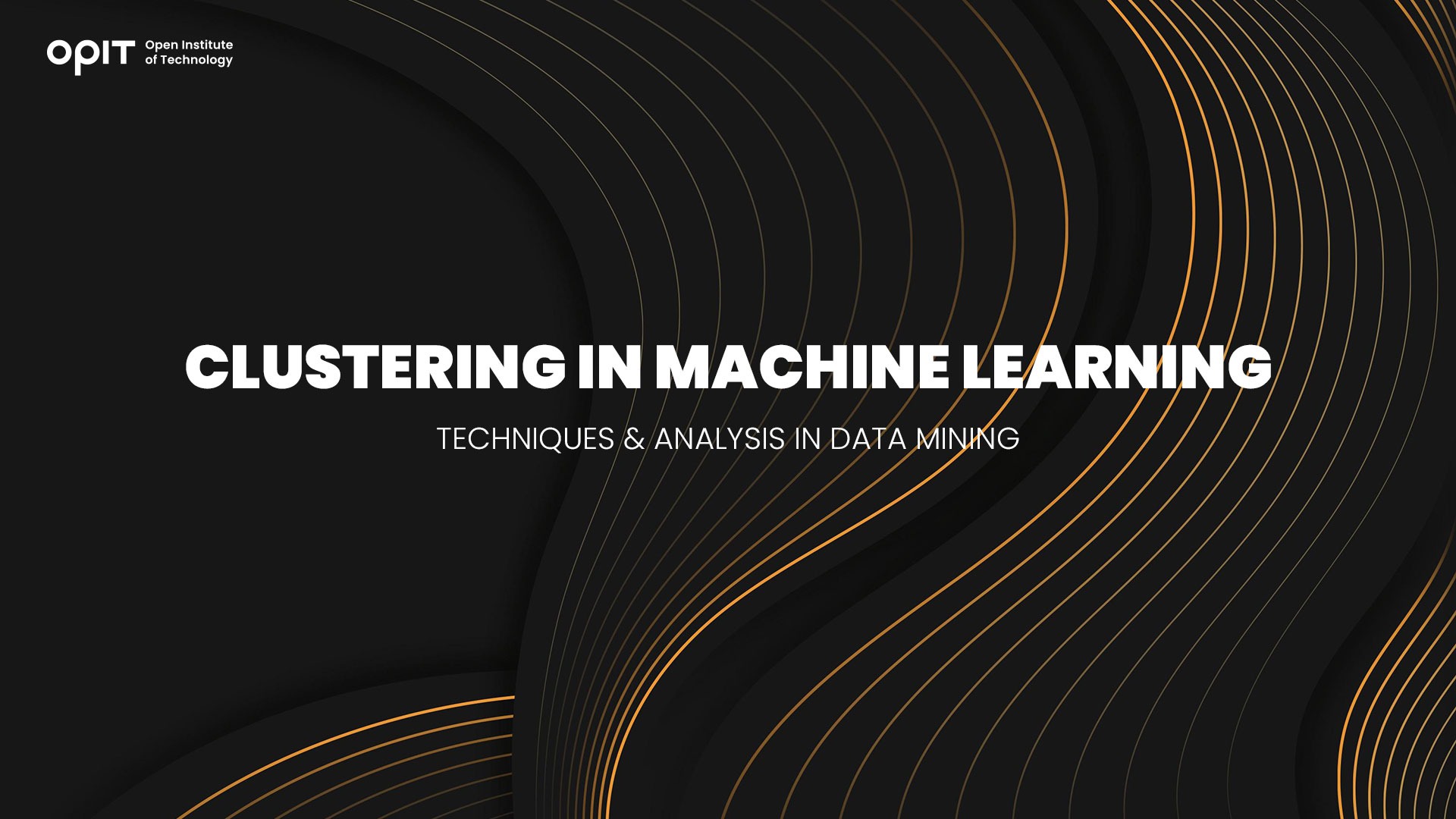
How do machine learning professionals make data readable and accessible? What techniques do they use to dissect raw information?
One of these techniques is clustering. Data clustering is the process of grouping items in a data set together. These items are related, allowing key stakeholders to make critical strategic decisions using the insights.
After preparing data, which is what specialists do 50%-80% of the time, clustering takes center stage. It forms structures other members of the company can understand more easily, even if they lack advanced technical knowledge.
Clustering in machine learning involves many techniques to help accomplish this goal. Here is a detailed overview of those techniques.
Clustering Techniques
Data science is an ever-changing field with lots of variables and fluctuations. However, one thing’s for sure – whether you want to practice clustering in data mining or clustering in machine learning, you can use a wide array of tools to automate your efforts.
Partitioning Methods
The first groups of techniques are the so-called partitioning methods. There are three main sub-types of this model.
K-Means Clustering
K-means clustering is an effective yet straightforward clustering system. To execute this technique, you need to assign clusters in your data sets. From there, define your number K, which tells the program how many centroids (“coordinates” representing the center of your clusters) you need. The machine then recognizes your K and categorizes data points to nearby clusters.
You can look at K-means clustering like finding the center of a triangle. Zeroing in on the center lets you divide the triangle into several areas, allowing you to make additional calculations.
And the name K-means clustering is pretty self-explanatory. It refers to finding the median value of your clusters – centroids.
K-Medoids Clustering
K-means clustering is useful but is prone to so-called “outlier data.” This information is different from other data points and can merge with others. Data miners need a reliable way to deal with this issue.
Enter K-medoids clustering.
It’s similar to K-means clustering, but just like planes overcome gravity, so does K-medoids clustering overcome outliers. It utilizes “medoids” as the reference points – which contain maximum similarities with other data points in your cluster. As a result, no outliers interfere with relevant data points, making this one of the most dependable clustering techniques in data mining.
Fuzzy C-Means Clustering
Fuzzy C-means clustering is all about calculating the distance from the median point to individual data points. If a data point is near the cluster centroid, it’s relevant to the goal you want to accomplish with your data mining. The farther you go from this point, the farther you move the goalpost and decrease relevance.
Hierarchical Methods
Some forms of clustering in machine learning are like textbooks – similar topics are grouped in a chapter and are different from topics in other chapters. That’s precisely what hierarchical clustering aims to accomplish. You can the following methods to create data hierarchies.
Agglomerative Clustering
Agglomerative clustering is one of the simplest forms of hierarchical clustering. It divides your data set into several clusters, making sure data points are similar to other points in the same cluster. By grouping them, you can see the differences between individual clusters.
Before the execution, each data point is a full-fledged cluster. The technique helps you form more clusters, making this a bottom-up strategy.
Divisive Clustering
Divisive clustering lies on the other end of the hierarchical spectrum. Here, you start with just one cluster and create more as you move through your data set. This top-down approach produces as many clusters as necessary until you achieve the requested number of partitions.
Density-Based Methods
Birds of a feather flock together. That’s the basic premise of density-based methods. Data points that are close to each other form high-density clusters, indicating their cohesiveness. The two primary density-based methods of clustering in data mining are DBSCAN and OPTICS.
DBSCAN (Density-Based Spatial Clustering of Applications With Noise)
Related data groups are close to each other, forming high-density areas in your data sets. The DBSCAN method picks up on these areas and groups information accordingly.
OPTICS (Ordering Points to Identify the Clustering Structure)
The OPTICS technique is like DBSCAN, grouping data points according to their density. The only major difference is that OPTICS can identify varying densities in larger groups.
Grid-Based Methods
You can see grids on practically every corner. They can easily be found in your house or your car. They’re also prevalent in clustering.
STING (Statistical Information Grid)
The STING grid method divides a data point into rectangular grills. Afterward, you determine certain parameters for your cells to categorize information.
CLIQUE (Clustering in QUEst)
Agglomerative clustering isn’t the only bottom-up clustering method on our list. There’s also the CLIQUE technique. It detects clusters in your environment and combines them according to your parameters.
Model-Based Methods
Different clustering techniques have different assumptions. The assumption of model-based methods is that a model generates specific data points. Several such models are used here.
Gaussian Mixture Models (GMM)
The aim of Gaussian mixture models is to identify so-called Gaussian distributions. Each distribution is a cluster, and any information within a distribution is related.
Hidden Markov Models (HMM)
Most people use HMM to determine the probability of certain outcomes. Once they calculate the probability, they can figure out the distance between individual data points for clustering purposes.
Spectral Clustering
If you often deal with information organized in graphs, spectral clustering can be your best friend. It finds related groups of notes according to linked edges.
Comparison of Clustering Techniques
It’s hard to say that one algorithm is superior to another because each has a specific purpose. Nevertheless, some clustering techniques might be especially useful in particular contexts:
- OPTICS beats DBSCAN when clustering data points with different densities.
- K-means outperforms divisive clustering when you wish to reduce the distance between a data point and a cluster.
- Spectral clustering is easier to implement than the STING and CLIQUE methods.
Cluster Analysis
You can’t put your feet up after clustering information. The next step is to analyze the groups to extract meaningful information.
Importance of Cluster Analysis in Data Mining
The importance of clustering in data mining can be compared to the importance of sunlight in tree growth. You can’t get valuable insights without analyzing your clusters. In turn, stakeholders wouldn’t be able to make critical decisions about improving their marketing efforts, target audience, and other key aspects.
Steps in Cluster Analysis
Just like the production of cars consists of many steps (e.g., assembling the engine, making the chassis, painting, etc.), cluster analysis is a multi-stage process:
Data Preprocessing
Noise and other issues plague raw information. Data preprocessing solves this issue by making data more understandable.
Feature Selection
You zero in on specific features of a cluster to identify those clusters more easily. Plus, feature selection allows you to store information in a smaller space.
Clustering Algorithm Selection
Choosing the right clustering algorithm is critical. You need to ensure your algorithm is compatible with the end result you wish to achieve. The best way to do so is to determine how you want to establish the relatedness of the information (e.g., determining median distances or densities).
Cluster Validation
In addition to making your data points easily digestible, you also need to verify whether your clustering process is legit. That’s where cluster validation comes in.
Cluster Validation Techniques
There are three main cluster validation techniques when performing clustering in machine learning:
Internal Validation
Internal validation evaluates your clustering based on internal information.
External Validation
External validation assesses a clustering process by referencing external data.
Relative Validation
You can vary your number of clusters or other parameters to evaluate your clustering. This procedure is known as relative validation.
Applications of Clustering in Data Mining
Clustering may sound a bit abstract, but it has numerous applications in data mining.
- Customer Segmentation – This is the most obvious application of clustering. You can group customers according to different factors, like age and interests, for better targeting.
- Anomaly Detection – Detecting anomalies or outliers is essential for many industries, such as healthcare.
- Image Segmentation – You use data clustering if you want to recognize a certain object in an image.
- Document Clustering – Organizing documents is effortless with document clustering.
- Bioinformatics and Gene Expression Analysis – Grouping related genes together is relatively simple with data clustering.
Challenges and Future Directions
- Scalability – One of the biggest challenges of data clustering is expected to be applying the process to larger datasets. Addressing this problem is essential in a world with ever-increasing amounts of information.
- Handling High-Dimensional Data – Future systems may be able to cluster data with thousands of dimensions.
- Dealing with Noise and Outliers – Specialists hope to enhance the ability of their clustering systems to reduce noise and lessen the influence of outliers.
- Dynamic Data and Evolving Clusters – Updates can change entire clusters. Professionals will need to adapt to this environment to retain efficiency.
Elevate Your Data Mining Knowledge
There are a vast number of techniques for clustering in machine learning. From centroid-based solutions to density-focused approaches, you can take many directions when grouping data.
Mastering them is essential for any data miner, as they provide insights into crucial information. On top of that, the data science industry is expected to hit nearly $26 billion by 2026, which is why clustering will become even more prevalent.
Related posts
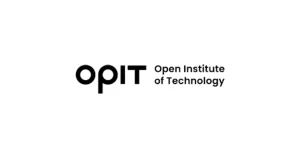
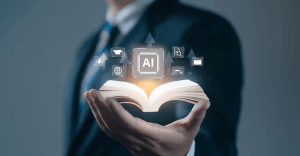
Source:
- Il Sole 24 Ore, published on June 23rd, 2025
At its core is a teaching heritage made up of 131 courses, 3,500 hours of video, 1,800 live sessions
Have questions?
Visit our FAQ page or get in touch with us!
Write us at +39 335 576 0263
Get in touch at hello@opit.com
Talk to one of our Study Advisors
We are international
We can speak in: