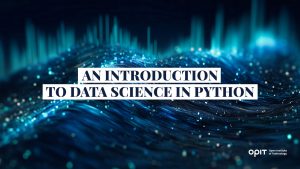
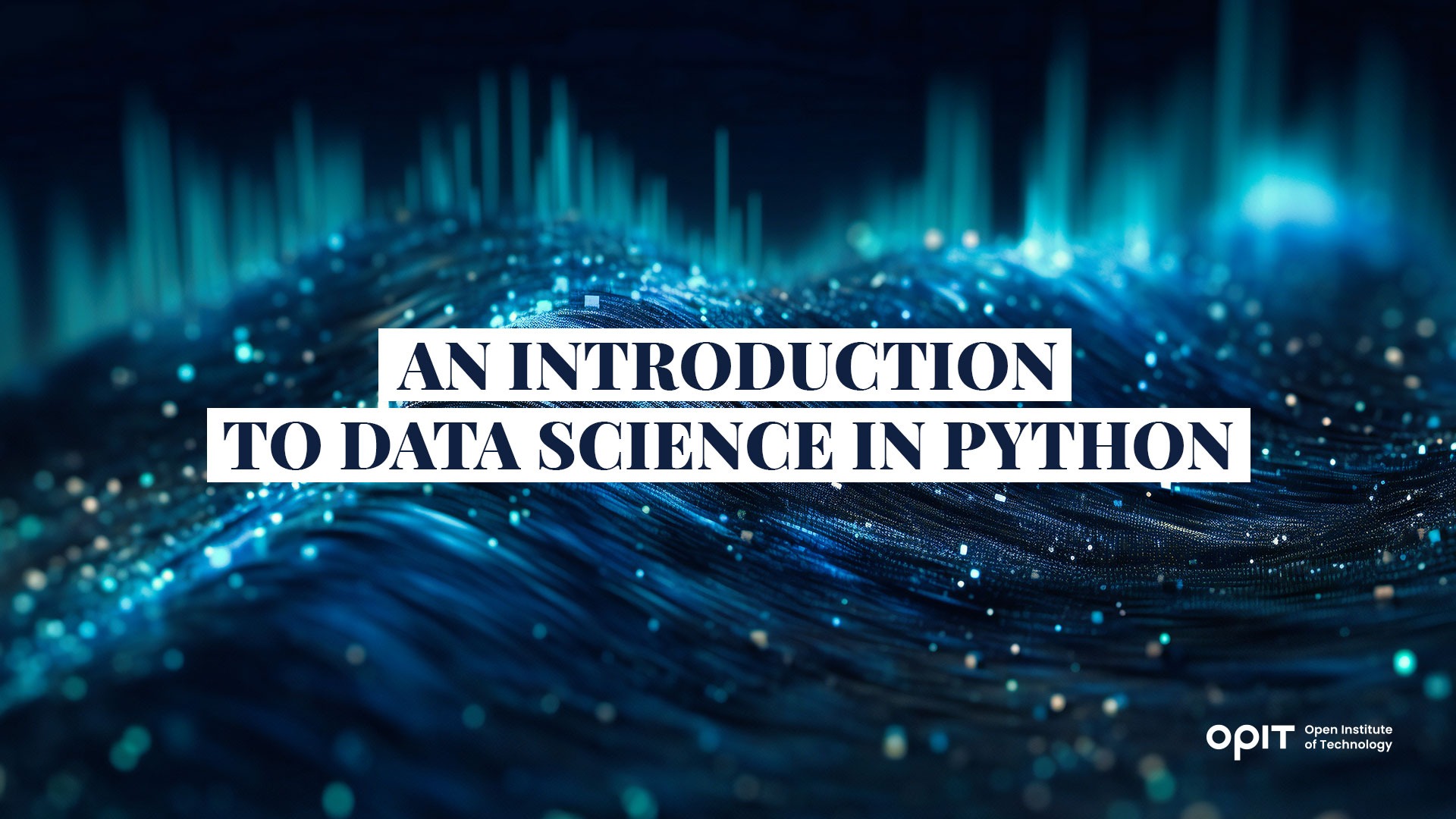
As one of the world’s fastest-growing industries, with a predicted compound annual growth rate of 16.43% anticipated between 2022 and 2030, data science is the ideal choice for your career. Jobs will be plentiful. Opportunities for career advancement will come thick and fast. And even at the most junior level, you’ll enjoy a salary that comfortably sits in the mid-five figures.
Studying for a career in this field involves learning the basics (and then the complexities) of programming languages including C+, Java, and Python. The latter is particularly important, both due to its popularity among programmers and the versatility that Python brings to the table. Here, we explore the importance of Python for data science and how you’re likely to use it in the real world.
Why Python for Data Science?
We can distill the reasons for learning Python for data science into the following five benefits.
Popularity and Community Support
Statista’s survey of the most widely-used programming languages in 2022 tells us that 48.07% of programmers use Python to some degree. Leftronic digs deeper into those numbers, telling us that there are 8.2 million Python developers in the world. As a prospective developer yourself, these numbers tell you two things – Python is in demand and there’s a huge community of fellow developers who can support you as you build your skills.
Easy to Learn and Use
You can think of Python as a primer for almost any other programming language, as it takes the fundamental concepts of programming and turns them into something practical. Getting to grips with concepts like functions and variables is simpler in Python than in many other languages. Python eventually opens up from its simplistic use cases to demonstrate enough complexity for use in many areas of data science.
Extensive Libraries and Tools
Given that Python was first introduced in 1991, it has over 30 years of support behind it. That, combined with its continued popularity, means that novice programmers can access a huge number of tools and libraries for their work. Libraries are especially important, as they act like repositories of functions and modules that save time by allowing you to benefit from other people’s work.
Integration With Other Programming Languages
The entire script for Python is written in C, meaning support for C is built into the language. While that enables easy integration between these particular languages, solutions exist to link Python with the likes of C++ and Java, with Python often being capable of serving as the “glue” that binds different languages together.
Versatility and Flexibility
If you can think it, you can usually do it in Python. Its clever modular structure, which allows you to define functions, modules, and entire scripts in different files to call as needed, makes Python one of the most flexible programming languages around.
Setting Up Python for Data Science
Installing Python onto your system of choice is simple enough. You can download the language from the Python.org website, with options available for everything from major operating systems (Windows, macOS, and Linux) to more obscure devices.
However, you need an integrated development environment (IDE) installed to start coding in Python. The following are three IDEs that are popular with those who use Python for data science:
- Jupyter Notebook – As a web-based application, Jupyter easily allows you to code, configure your workflows, and even access various libraries that can enhance your Python code. Think of it like a one-stop shop for your Python needs, with extensions being available to extend its functionality. It’s also free, which is never a bad thing.
- PyCharm – Where Jupyter is an open-source IDE for several languages, PyCharm is for Python only. Beyond serving as a coding tool, it offers automated code checking and completion, allowing you to quickly catch errors and write common code.
- Visual Studio Code – Though Visual Studio Code alone isn’t compatible with Python, it has an extension that allows you to edit Python code on any operating system. Its “Linting” feature is great for catching errors in your code, and it comes with an integrated debugger that allows you to test executables without physically running them.
Setting up your Python virtual environment is as simple as downloading and installing Python itself, and then choosing an IDE in which to work. Think of Python as the materials you use to build a house, with your IDE being both the blueprint and the tools you’ll need to patch those materials together.
Essential Python Libraries for Data Science
Just as you’ll go to a real-world library to check out books, you can use Python libraries to “check out” code that you can use in your own programs. It’s actually better than that because you don’t need to return libraries when you’re done with them. You get to keep them, along with all of their built-in modules and functions, to call upon whenever you need them. In Python for data science, the following are some essential libraries:
- NumPy – We spoke about integration earlier, and NumPy is ideal for that. It brings concepts of functionality from Fortran and C into Python. By expanding Python with powerful array and numerical computing tools, it helps transform it into a data science powerhouse.
- pandas – Manipulating and analyzing data lies at the heart of data sciences, and pandas give you a library full of tools to allow both. It offers modules for cleaning data, plotting, finding correlations, and simply reading CSV and JSON files.
- Matplotlib – Some people can look at reams of data and see patterns form within the numbers. Others need visualization tools, which is where Matplotlib excels. It helps you create interactive visual representations of your data for use in presentations or if you simply prefer to “see” your data rather than read it.
- Scikit-learn – The emerging (some would say “exploding) field of machine learning is critical to the AI-driven future we’re seemingly heading toward. Scikit-learn is a library that offers tools for predictive data analysis, built on what’s available in the NumPy and Matplotlib libraries.
- TensorFlow and Keras – Much like Scikit-learn, both TensorFlow and Keras offer rich libraries of tools related to machine learning. They’re essential if your data science projects take you into the realms of neural networks and deep learning.
Data Science Workflow in Python
A Python programmer without a workflow is like a ship’s captain without a compass. You can sail blindly onward, and you may even get lucky and reach your destination, but the odds are you’re going to get lost in the vastness of the programming sea. For those who want to use Python for data science, the following workflow brings structure and direction to your efforts.
Step 1 – Data Collection and Preprocessing
You need to collect, organize, and import your data into Python (as well as clean it) before you can draw any conclusions from it. That’s why the first step in any data science workflow is to prepare the data for use (hint – the pandas library is perfect for this task).
Step 2 – Exploratory Data Analysis (EDA)
Just because you have clean data, that doesn’t mean you’re ready to investigate what that data tells you. It’s like washing ingredients before you make a dish – you need to have a “recipe” that tells you how to put everything together. Data scientists use EDA as this recipe, allowing them to combine data visualization (remember – the Matplotlib library) with descriptive statistics that show them what they’re looking at.
Step 3 – Feature Engineering
This is where you dig into the “whats” and “hows” of your Python program. You’ll select features for the code, which define what it does with the data you import and how it’ll deliver outcomes. Scaling is a key part of this process, with scope creep (i.e., constantly adding features as you get deeper into a project) being the key thing to avoid.
Step 4 – Model Selection and Training
Decision trees, linear regression, logistic regression, neural networks, and support vector machines. These are all models (with their own algorithms) you can use for your data science project. This step is all about selecting the right model for the job (your intended features are important here) and training that model so it produces accurate outputs.
Step 5 – Model Evaluation and Optimization
Like a puppy that hasn’t been house trained, an unevaluated model isn’t ready for release into the real world. Classification metrics, such as a confusion matrix and classification report, help you to evaluate your model’s predictions against real-world results. You also need to tune the hyperparameters built into your model, similar to how a mechanic may tune the nuts and bolts in a car, to get everything working as efficiently as possible.
Step 6 – Deployment and Maintenance
You’ve officially deployed your Python for data science model when you release it into the wild and let it start predicting outcomes. But the work doesn’t end at deployment, as constant monitoring of what your model does, outputs, and predicts is needed to tell you if you need to make tweaks or if the model is going off the rails.
Real-World Data Science Projects in Python
There are many examples of Python for data science in the real world, some of which are simple while others delve into some pretty complex datasets. For instance, you can use a simple Python program to scrap live stock prices from a source like Yahoo! Finance, allowing you to create a virtual ticker of stock price changes for investors.
Alternatively, why not create a chatbot that uses natural language processing to classify and respond to text? For that project, you’ll tokenize sentences, essentially breaking them down into constituent words called “tokens,” and tag those tokens with meanings that you could use to prompt your program toward specific responses.
There are plenty of ideas to play around with, and Python is versatile enough to enable most, so consider what you’d like to do with your program and then go on the hunt for datasets. Great (and free) resources include The Boston House Price Dataset, ImageNet, and IMDB’s movie review database.
Try Python for Data Science Projects
By combining its own versatility with integrations and an ease of use that makes it welcoming to beginners, Python has become one of the world’s most popular programming languages. In this introduction to data science in Python, you’ve discovered some of the libraries that can help you to apply Python for data science. Plus, you have a workflow that lends structure to your efforts, as well as some ideas for projects to try. Experiment, play, and tweak models. Every minute you spend applying Python to data science is a minute spent learning a popular programming language in the context of a rapidly-growing industry.
Related posts
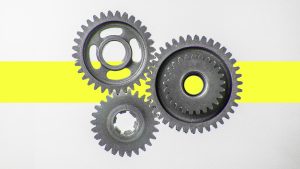
Source:
- IE University – Insights, Published on October 15th, 2024.
By Francesco Derchi
Purpose is a strategic tool for driving innovation, competitive advantage, and addressing AI challenges, writes Francesco Derchi.
Since the early 2000s, technology has dominated discussions among scholars and professionals about global development and economic trends, with the first wave of research regarding the internet’s impact on firms and society focusing on the enabling potential of technologies. The concept of “digital revolution,” as popularized by Nicholas Negroponte, became the new paradigm for broader considerations about the development of the firm’s macro environment, and how businesses could leverage it as an asset for creating competitive advantage. The following wave focused on the convergence of different technologies, such as manufacturing, and included the dynamics of coexistence between humans and machines. From the management side, the major challenges are related to defining effective digital transformation practices that could help to migrate organizations and exploit this new paradigm.
The current technological focus builds on these previous trends, particularly on artificial intelligence and more recently on the emergence of generative AI. The Age of AI is characterized by technology’s power to reshape business and society on a variety of levels. While AI’s pervasive impact is not new for firms, the mainstream adoption of ChatGPT for business purposes and the response to this ready adoption from big tech players like Microsoft, Google, and more recently Apple, shows how AI is reshaping and influencing companies’ strategic priorities.
From a research perspective, AI’s societal impact is inspiring new studies in the field of ethics. Luciano Floridi, now of Yale University, has identified several challenges for AI, characterizing them by global magnitudes like its environmental impact and has identified several challenges for AI security, including intellectual property, privacy, transparency, and accountability. In his work, Floridi underlines the importance of philosophy in defining problems and designing solutions – but it is equally important to consider how these challenges can be addressed at the firm level. What are the tools for managers?
Part of the answer may lie in the increasing and recent focus of management studies around “corporate purpose” and “brand purpose.” This trend represents an important attempt to deepen our understanding of “why to act” (purpose framing) and “how to act” (purpose formalizing and internalizing), while technology management studies address the “what to act” (purpose impacting) question. Furthermore, studies show that corporate purpose is critical for both digital native firms as well as traditional companies undergoing a digital transformation, serving as an important growth engine through purpose-driven innovation. It is therefore fair to ask: can purpose help in addressing any of the AI challenges previously mentioned?
Purpose concepts are not exclusively “cause-related” like CSR and environmental impact. Other types have emerged, such as “competence” (the function of the product) and “culture” (the intent that drives the business). This broadens the consideration of impact types that can help address specific challenges in the age of AI.
Purpose-driven organizations are not new. Take Tesla’s direction “to accelerate the world’s transition to sustainable energy” – it explicitly addresses environmental challenges while defining a business direction that requires constant innovation and leverages multiple converging technologies. The key is to have the purpose formalized and internalized within the company as a concrete drive for growth.
Due to its characteristics, the MTP plays a key role in digital transformation. This necessarily ambitious and long-term vision or goal – the Massive Transformative Purpose – requires firms, particularly those focused on exponential growth, to address emerging accelerating technologies with a purpose-first transformation logic. P&G’s Global Business Services division was able to improve market leadership and gain a competitive advantage over various start-ups and potential disruptors through its “Free up the employee, for free” MTP. This served as a north star for every employee, encouraging them to contribute ideas and best practices to overcome bulky processes and limitations.
My research on MTPs in AI-era firms explores their role in driving innovation to address specific challenges. Results show that the MTP impacts the organization across four dimensions, requiring commitment and synergy from management. Let’s consider these four dimensions by looking at Airbnb:
- Internal Impact: The MTP acts as the organization’s genetic code and guiding philosophy. It is key for leveraging employee motivation, with a strong relationship between purpose, organizational culture, and firm values. Airbnb’s culture of belonging highlights this, with its various purpose-shaping practices, starting with culture-fit interviews delivered during the recruitment process.
- Brand and Market Influence: The MTP contributes directly to building a strong brand and influencing the market. It allows firms to extend beyond functional and symbolic benefits to make the impact of the company on society visible. This involves addressing market demand coherently and consistently. Airbnb’s “Bélo” symbol visually represents this concept of belonging while their MTP features in campaigns like “Wall and Chain: A Story of Breaking Down Walls.”
- Competitive Advantage and Growth: The MTP drives innovation and can lead to superior stock market performance. In digital firms, it’s key in the creation of ecosystems that aggregate leveraged assets and third parties for value creation. The company’s “belong anywhere transformation journey” is a strategic initiative that formalized and interiorized the MTP through various touchpoints for all the different ecosystem members. As Leigh Gallagher details in her 2016 Fortune feature about the company, “When travellers leave their homes, they feel alone. They reach their Airbnb, and they feel accepted and taken care of by their host. They then feel safe to be the same kind of person they are when they’re at home.”
- Core Organization Identity: The MTP is considered part of the core dimension of the organization. More than a goal or business strategy, it is a strategic issue that generates a sense of direction and purpose that affects every part of the organization: internal, external, personality, and expression. This dimension also involves the role of the founder(s) and their personality in shaping the business. At Airbnb, the MTP is often used as a shortcut to explain the firm’s mission and vision. The founders’ approach is pragmatic, and instead of debating differences, time should be spent on execution. At the same time, the personalities of the three founders, Chesky, Gebbia, and Blecharcyzk, are the identity of the firm. They were the first hosts for the platform. Their credibility is key for making Airbnb a trustworthy and coherent proposal in a crowded market.
Executives and leaders of business in the current AI era should embrace three key principles. Be true: Purpose is an essential strategic tool that enables firms to identify and connect with their original selves, decoding their reason for being and embedding it into their identity. Be ambitious: The MTP allows for global impact, confronting major challenges by synthesizing business values and guiding innovation paths to address AI-related issues. Be generous: Purpose allows firms to explicitly address environmental and social issues, taking action on values-based challenges such as transparency, respect for intellectual property, and accountability. By following these principles, organizations and their leaders can maintain their direction and continue to advance in the AI era.
Read the full article below:
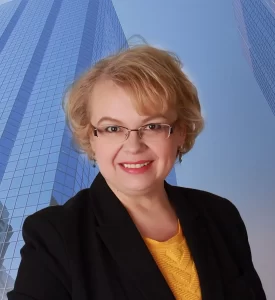
Source:
- Authority Magazine Medium, Published on September 15th, 2024.
Gaining hands-on experience through projects, internships, and collaborations is vital for understanding how to apply AI in various industries and domains. Use Kaggle or get a free cloud account and start experimenting. You will have projects to discuss at your next interviews.
By David Leichner, CMO at Cybellum
14 min read
Artificial Intelligence is now the leading edge of technology, driving unprecedented advancements across sectors. From healthcare to finance, education to environment, the AI industry is witnessing a skyrocketing demand for professionals. However, the path to creating a successful career in AI is multifaceted and constantly evolving. What does it take and what does one need in order to create a highly successful career in AI?
In this interview series, we are talking to successful AI professionals, AI founders, AI CEOs, educators in the field, AI researchers, HR managers in tech companies, and anyone who holds authority in the realm of Artificial Intelligence to inspire and guide those who are eager to embark on this exciting career path.
As part of this series, we had the pleasure of interviewing Zorina Alliata.
Zorina Alliata is an expert in AI, with over 20 years of experience in tech, and over 10 years in AI itself. As an educator, Zorina Alliata is passionate about learning, access to education and about creating the career you want. She implores us to learn more about ethics in AI, and not to fear AI, but to embrace it.
Thank you so much for joining us in this interview series! Before we dive in, our readers would like to learn a bit about your origin story. Can you share with us a bit about your childhood and how you grew up?
I was born in Romania, and grew up during communism, a very dark period in our history. I was a curious child and my parents, both teachers, encouraged me to learn new things all the time. Unfortunately, in communism, there was not a lot to do for a kid who wanted to learn: there was no TV, very few books and only ones that were approved by the state, and generally very few activities outside of school. Being an “intellectual” was a bad thing in the eyes of the government. They preferred people who did not read or think too much. I found great relief in writing, I have been writing stories and poetry since I was about ten years old. I was published with my first poem at 16 years old, in a national literature magazine.
Can you share with us the ‘backstory’ of how you decided to pursue a career path in AI?
I studied Computer Science at university. By then, communism had fallen and we actually had received brand new PCs at the university, and learned several programming languages. The last year, the fifth year of study, was equivalent with a Master’s degree, and was spent preparing your thesis. That’s when I learned about neural networks. We had a tiny, 5-node neural network and we spent the year trying to teach it to recognize the written letter “A”.
We had only a few computers in the lab running Windows NT, so really the technology was not there for such an ambitious project. We did not achieve a lot that year, but I was fascinated by the idea of a neural network learning by itself, without any programming. When I graduated, there were no jobs in AI at all, it was what we now call “the AI winter”. So I went and worked as a programmer, then moved into management and project management. You can imagine my happiness when, about ten years ago, AI came back to life in the form of Machine Learning (ML).
I immediately went and took every class possible to learn about it. I spent that Christmas holiday coding. The paradigm had changed from when I was in college, when we were trying to replicate the entire human brain. ML was focused on solving one specific problem, optimizing one specific output, and that’s where businesses everywhere saw a benefit. I then joined a Data Science team at GEICO, moved to Capital One as a Delivery lead for their Center for Machine Learning, and then went to Amazon in their AI/ML team.
Can you tell our readers about the most interesting projects you are working on now?
While I can’t discuss work projects due to confidentiality, there are some things I can mention! In the last five years, I worked with global companies to establish an AI strategy and to introduce AI and ML in their organizations. Some of my customers included large farming associations, who used ML to predict when to plant their crops for optimal results; water management companies who used ML for predictive maintenance to maintain their underground pipes; construction companies that used AI for visual inspections of their buildings, and to identify any possible defects and hospitals who used Digital Twins technology to improve patient outcomes and health. It is amazing to see how much AI and ML are already part of our everyday lives, and to recognize some of it in the mundane around us.
None of us are able to achieve success without some help along the way. Is there a particular person who you are grateful for who helped get you to where you are? Can you share a story about that?
When you are young, there are so many people who step up and help you along the way. I have had great luck with several professors who have encouraged me in school, and an uncle who worked in computers who would take me to his office and let me play around with his machines. I now try to give back and mentor several young people, especially women who are trying to get into the field. I volunteer with AnitaB and Zonta, as well as taking on mentees where I work.
As with any career path, the AI industry comes with its own set of challenges. Could you elaborate on some of the significant challenges you faced in your AI career and how you managed to overcome them?
I think one major challenge in AI is the speed of change. I remember after spending my Christmas holiday learning and coding in R, when I joined the Data Science team at GEICO, I realized the world had moved on and everyone was now coding in Python. So, I had to learn Python very fast, in order to understand what was going on.
It’s the same with research — I try to work on one subject, and four new papers are published every week that move the goal posts. It is very challenging to keep up, but you just have to adapt to continuously learn and let go of what becomes obsolete.
Ok, let’s now move to the main part of our interview about AI. What are the 3 things that most excite you about the AI industry now? Why?
1. Creativity
Generative AI brought us the ability to create amazing images based on simple text descriptions. Entire videos are now possible, and soon, maybe entire movies. I have been working in AI for several years and I never thought creative jobs will be the first to be achieved by AI. I am amazed at the capacity of an algorithms to create images, and to observe the artificial creativity we now see for the first time.
2. Abstraction
I think with the success and immediate mainstream adoption of Generative AI, we saw the great appetite out there for automation and abstraction. No one wants to do boring work and summarizing documents; no one wants to read long websites, they just want the gist of it. If I drive a car, I don’t need to know how the engine works and every equation that the engineers used to build it — I just want my car to drive. The same level of abstraction is now expected in AI. There is a lot of opportunity here in creating these abstractions for the future.
3. Opportunity
I like that we are in the beginning of AI, so there is a lot of opportunity to jump in. Most people who are passionate about it can learn all about AI fully online, in places like Open Institute of Technology. Or they can get experience working on small projects, and then they can apply for jobs. It is great because it gives people access to good jobs and stability in the future.
What are the 3 things that concern you about the AI industry? Why? What should be done to address and alleviate those concerns?
1. Fairness
The large companies that build LLMs spend a lot of energy and money into making them fair. But it is not easy. Us, as humans, are often not fair ourselves. We even have problems agreeing what fairness even means. So, how can we teach the machines to be fair? I think the responsibility stays with us. We can’t simply say “AI did this bad thing.”
2. Regulation
There are some regulations popping up but most are not coordinated or discussed widely. There is controversy, such as regarding the new California bill SB1047, where scientists take different sides of the debate. We need to find better ways to regulate the use and creation of AI, working together as a society, not just in small groups of politicians.
3. Awareness
I wish everyone understood the basics of AI. There is denial, fear, hatred that is created by doomsday misinformation. I wish AI was taught from a young age, through appropriate means, so everyone gets the fundamental principles and understands how to use this great tool in their lives.
For a young person who would like to eventually make a career in AI, which skills and subjects do they need to learn?
I think maybe the right question is: what are you passionate about? Do that, and see how you can use AI to make your job better and more exciting! I think AI will work alongside people in most jobs, as it develops and matures.
But for those who are looking to work in AI, they can choose from a variety of roles as well. We have technical roles like data scientist or machine learning engineer, which require very specialized knowledge and degrees. They learn computing, software engineering, programming, data analysis, data engineering. There are also business roles, for people who understand the technology well but are not writing code. Instead, they define strategies, design solutions for companies, or write implementation plans for AI products and services. There is also a robust AI research domain, where lots of scientists are measuring and analyzing new technology developments.
With Generative AI, new roles appeared, such as Prompt Engineer. We can now talk with the machines in natural language, so speaking good English is all that’s required to find the right conversation.
With these many possible roles, I think if you work in AI, some basic subjects where you can start are:
- Analytics — understand data and how it is stored and governed, and how we get insights from it.
- Logic — understand both mathematical and philosophical logic.
- Fundamentals of AI — read about the history and philosophy of AI, models of thinking, and major developments.
As you know, there are not that many women in the AI industry. Can you advise what is needed to engage more women in the AI industry?
Engaging more women in the AI industry is absolutely crucial if you want to build any successful AI products. In my twenty years career, I have seen changes in the tech industry to address this gender discrepancy. For example, we do well in school with STEM programs and similar efforts that encourage girls to code. We also created mentorship organizations such as AnitaB.org who allow women to connect and collaborate. One place where I think we still lag behind is in the workplace. When I came to the US in my twenties, I was the only woman programmer in my team. Now, I see more women at work, but still not enough. We say we create inclusive work environments, but we still have a long way to go to encourage more women to stay in tech. Policies that support flexible hours and parental leave are necessary, and other adjustments that account for the different lives that women have compared to men. Bias training and challenging stereotypes are also necessary, and many times these are implemented shoddily in organizations.
Ethical AI development is a pressing concern in the industry. How do you approach the ethical implications of AI, and what steps do you believe individuals and organizations should take to ensure responsible and fair AI practices?
Machine Learning and AI learn from data. Unfortunately, lot of our historical data shows strong biases. For example, for a long time, it was perfectly legal to only offer mortgages to white people. The data shows that. If we use this data to train a new model to enhance the mortgage application process, then the model will learn that mortgages should only be offered to white men. That is a bias that we had in the past, but we do not want to learn and amplify in the future.
Generative AI has introduced a new set of fresh risks, the most famous being the “hallucinations.” Generative AI will create new content based on chunks of text it finds in its training data, without an understanding of what the content means. It could repeat something it learned from one Reddit user ten years ago, that could be factually incorrect. Is that piece of information unbiased and fair?
There are many ways we fight for fairness in AI. There are technical tools we can use to offer interpretability and explainability of the actual models used. There are business constraints we can create, such as guardrails or knowledge bases, where we can lead the AI towards ethical answers. We also advise anyone who build AI to use a diverse team of builders. If you look around the table and you see the same type of guys who went to the schools, you will get exactly one original idea from them. If you add different genders, different ages, different tenures, different backgrounds, then you will get ten innovative ideas for your product, and you will have addressed biases you’ve never even thought of.
Read the full article below:
Have questions?
Visit our FAQ page or get in touch with us!
Write us at +39 335 576 0263
Get in touch at hello@opit.com
Talk to one of our Study Advisors
We are international
We can speak in: