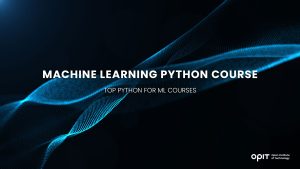
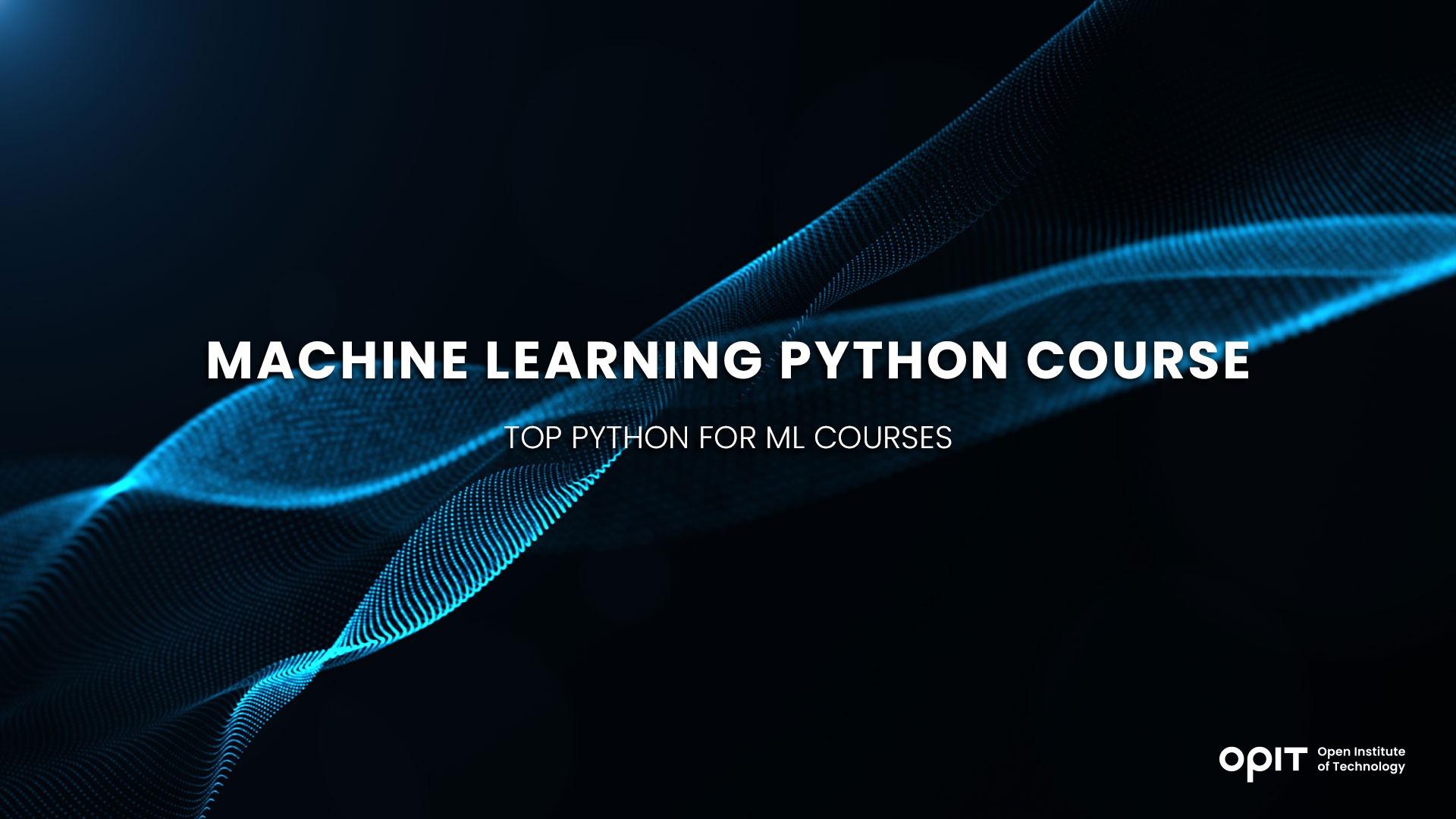
Machine learning (ML) is a branch of artificial intelligence (AI) that focuses on enabling computers to “think” for themselves. Of course, they owe this thinking to humans (data scientists and ML engineers) who continuously supervise ML algorithms and models.
So, there’s no AI takeover (for now at least), just incredible ways to propel several industries forward by automating repetitive tasks, extracting valuable insights from data, and improving decision-making processes.
But how do humans precisely communicate with computers in machine learning?
The answer is through programming languages.
One programming language stands out among the rest for its simplicity and versatility. By the title of this guide, you can already guess we’re talking about Python.
This beloved programming language is all over the machine learning field, so mastering it gives you a great head start in the industry.
With this in mind, let’s examine how you can learn Python for machine learning courses. If you already have some basic knowledge of this programming language, don’t worry. We’ll also mention a great machine learning Python course to take your knowledge to the next level.
Factors to Consider When Choosing a Python for ML Course
Do a Google search for “machine learning Python course,” and you’ll be met with dozens of web pages that promise a sound understanding of this programming language. However, you’ll find the best course for your needs if you can identify those needs first.
Course Content and Curriculum
Your chosen course’s curriculum is arguably the most important factor for selecting the perfect machine learning Python course. One look at the listed topics, and you’ll know whether the course is right for you.
Let’s take your previous experience with Python as an example. If you have none, a course that jumps straight into machine learning algorithms without covering the Python basics will obviously not work for you.
Instructor’s Expertise and Experience
What bridges the gap between struggling to comprehend a complex subject and feeling that nothing can stop you in your learning journey? The answer is simple – a good instructor.
Before committing to a course, check who teaches it. Find out the instructor’s background with Python and whether they have enough expertise to guide you through this programming language’s intricacies.
If their bio checks all the boxes, watch at least one of their lectures. It doesn’t hurt to check whether their teaching style and voice suit you, as these can also make or break your learning experience.
Course Duration and Flexibility
Most online courses are self-paced, allowing you to create your own schedule. Fixed-timing courses also have their benefits, though. They’re usually instructor-led, so you can use the opportunity to ask questions and receive clarification as you learn the material.
As for duration, the course’s description typically indicates how long the course lasts and the recommended pace. Before starting, make sure you can commit to the course from beginning to end. Otherwise, you’re just wasting time and gaining incomplete knowledge.
Hands-On Projects and Real-World Applications
Programming languages are inherently practical, so ensure that your chosen course features hands-on projects and practical examples. Sticking solely to theory will do little to prepare you for what’s waiting in the real world.
Course Reviews and Ratings
You probably check reviews before going to a new restaurant, renting an Airbnb, or purchasing clothes online. So why should shopping for online courses be any different? When a course piques your interest, check how other learners have rated it. But don’t stop at glancing at the average rating. Read through some reviews to ensure they aren’t fake and to get a better picture of the course’s quality.
Pricing and Value for Money
There are plenty of free machine learning resources online. But the more advanced courses and certificates usually come with a fee. And that’s perfectly understandable. What’s not understandable or acceptable are courses that charge ridiculously high fees yet offer little value. To avoid wasting money (and probably time), check whether the course’s price is justifiable by its duration, level, type, and provided support.
Top Python for ML Courses Reviewed
Here are our favorite Python courses primarily focused on machine learning. We’re positive you’ll find the perfect machine learning Python course, whether this is the first time you use this programming language or want to master this skill.
Python for Machine Learning
The Python for Machine Learning course on Great Learning is a great place to start your Python-learning journey. This course is beginner-friendly and relatively short, so you won’t get overwhelmed from the get-go.
This course focuses on three Python libraries: NumPy, Pandas, and Matplotlib. It guides you through the basic concepts (arrays, intersection, loading, etc.) and then moves on to more complex functions. At the end of the course, you take a quiz. Pass the quiz, and you’ll get a certificate of completion.
Applying for this course is free. Not only that, but you’ll also receive free lifetime access, so you can revisit the course whenever you’d like. Although, some learners believe that there’s little to revisit. In total, this course lasts for 90 minutes. Those who are serious about Python learning will probably need more than this.
Still, you can view this course as a beginner’s guide and move to more advanced lessons afterward. To apply, you only need to create an account on the platform and send an enrollment request.
Machine Learning A-Z: AI, Python & R
If you want to start with the basics but cover the more advanced stuff within the same course, this Udemy’s gem is for you. It covers another programming language besides Python, R. However, this won’t be an issue, as you can focus solely on Python.
The course is broken into 10 parts, with over 40 hours of on-demand videos. Each section (and even the lessons within them) is separate, so you can choose to complete the ones that will benefit you now. Start with data preprocessing, and work toward machine learning model selection.
Those seeking practical exercises in Python will love this course. However, you might need to research some notions independently, as not all lecture sections are explained in great detail.
You can purchase lifetime access to this course for $89.99 (a little over €83). The price includes a certificate of completion and several additional learning materials (articles and downloadable resources). Complete the purchase to apply for this course.
Machine Learning With Python by IBM
IBM is one of the leading companies in the machine learning field, so you should take advantage of every chance to learn from its experts. If you’re just gaining your footing in machine learning, you’ll cover all your bases with this offering.
It will take approximately 12 hours over four weeks to complete the coursework. After each lesson, you’ll get a chance to put your newly-learned knowledge to the test.
One thing to keep in mind is that this course focuses more on machine learning using Python than the programming language itself. So, if you’ve never worked with Python, an additional resource or two might come in handy.
You can use Coursera’s 7-day trial to enroll in this course. Afterward, you’ll be charged $39 (approximately €36) a month. The same fee is a must if you want to receive a certificate.
The Complete Machine Learning Course With Python
Are you a data scientist in the making looking to build a solid portfolio with Python? If yes, you’ll love this course. You can find it on Udemy, just like millions of learners before you. This number might surprise you at first. But once you see that one of the founders of this course is Andrew Ng, a thought leader in machine learning, it will make much more sense.
In 18 hours, this course covers all the basics of machine learning with Python. But there’s a catch. You’ll need at least basic Python programming knowledge to keep up.
If this isn’t an issue, create an Udemy account and pay the $59.99 (around €55.50) fee to apply. Lifetime access and a certificate of completion are included.
Programming for Everybody (Getting Started With Python)
While not focused on machine learning per se, this course is necessary for anyone who has yet to work with Python. Pair it with one of the other courses on our list, and your success is guaranteed.
As the name implies, this course covers all the basics. It is designed to allow virtually anyone to follow, regardless of their skills. The simplest math is all you need.
You’ll also need 19 hours to complete this course offered by the University of Michigan. However, the instructor snuck a couple of non-Python-related stories into those 19 hours, which some learners didn’t like.
If you don’t mind a break here and there, join this course on Coursera for free or $49 (a little over €45) if you want a certificate.
Additional Resources for Learning Python for Machine Learning
Perhaps you can’t get enough of learning about Python. Or you find Python for machine learning courses lacking information. Whatever the case, you can find additional resources (both online and offline) to help you master this programming language. Check out some of our favorites:
- Books and e-books: “Python for Data Science, for Dummies,” “Introduction to Machine Learning with Python: A Guide for Data Scientists,” “Python Data Science Handbook: Essential Tools for Working with Data”
- Blogs: Planet Python, Real Python
- YouTube channels: IBM Technology, Google Career Certificates, techTFQ
- Community forums and discussion groups: Kaggle Discussions, Reddit (r/learnpython)
The Path to Python
As you can see, there’s no shortage of Python for machine learning courses, even hosted by some of the biggest names in the industry. Take one of the listed courses or combine them; the choice is all yours. All that matters is that you ultimately master this programming language and crush any data science career you choose.
If these courses aren’t enough to quench your thirst for knowledge, a Bachelor’s in Modern Computer Science will definitely do the trick. With it, you can learn all the ins and outs of Python and machine learning in general.
Related posts
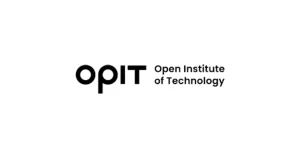
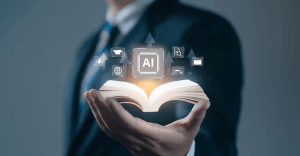
Source:
- Il Sole 24 Ore, published on June 23rd, 2025
At its core is a teaching heritage made up of 131 courses, 3,500 hours of video, 1,800 live sessions
Have questions?
Visit our FAQ page or get in touch with us!
Write us at +39 335 576 0263
Get in touch at hello@opit.com
Talk to one of our Study Advisors
We are international
We can speak in: