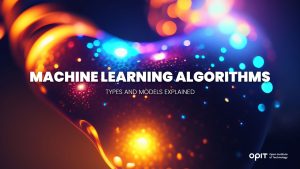
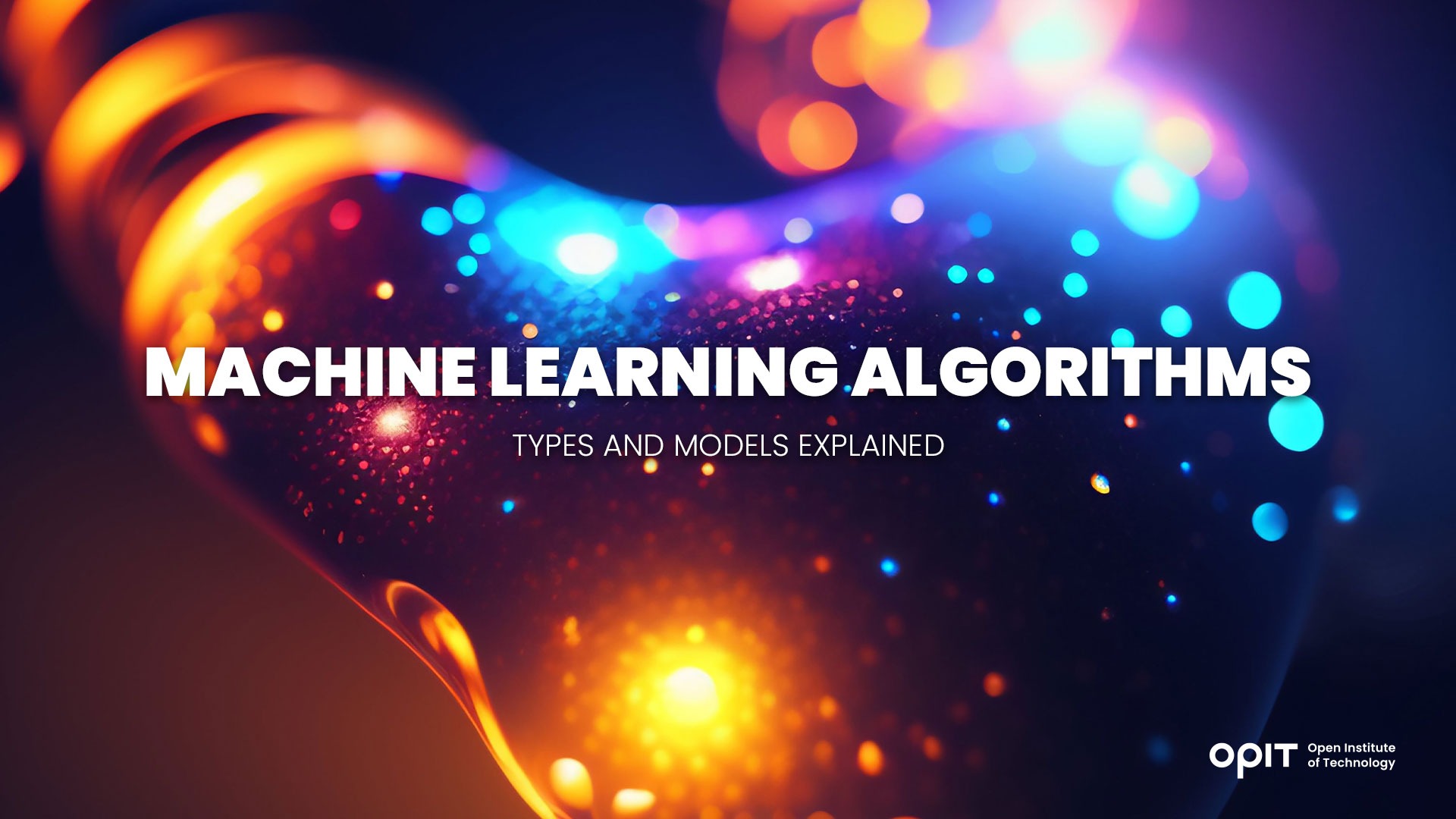
Any tendency or behavior of a consumer in the purchasing process in a certain period is known as customer behavior. For example, the last two years saw an unprecedented rise in online shopping. Such trends must be analyzed, but this is a nightmare for companies that try to take on the task manually. They need a way to speed up the project and make it more accurate.
Enter machine learning algorithms. Machine learning algorithms are methods AI programs use to complete a particular task. In most cases, they predict outcomes based on the provided information.
Without machine learning algorithms, customer behavior analyses would be a shot in the dark. These models are essential because they help enterprises segment their markets, develop new offerings, and perform time-sensitive operations without making wild guesses.
We’ve covered the definition and significance of machine learning, which only scratches the surface of this concept. The following is a detailed overview of the different types, models, and challenges of machine learning algorithms.
Types of Machine Learning Algorithms
A natural way to kick our discussion into motion is to dissect the most common types of machine learning algorithms. Here’s a brief explanation of each model, along with a few real-life examples and applications.
Supervised Learning
You can come across “supervised learning” at every corner of the machine learning realm. But what is it about, and where is it used?
Definition and Examples
Supervised machine learning is like supervised classroom learning. A teacher provides instructions, based on which students perform requested tasks.
In a supervised algorithm, the teacher is replaced by a user who feeds the system with input data. The system draws on this data to make predictions or discover trends, depending on the purpose of the program.
There are many supervised learning algorithms, as illustrated by the following examples:
- Decision trees
- Linear regression
- Gaussian Naïve Bayes
Applications in Various Industries
When supervised machine learning models were invented, it was like discovering the Holy Grail. The technology is incredibly flexible since it permeates a range of industries. For example, supervised algorithms can:
- Detect spam in emails
- Scan biometrics for security enterprises
- Recognize speech for developers of speech synthesis tools
Unsupervised Learning
On the other end of the spectrum of machine learning lies unsupervised learning. You can probably already guess the difference from the previous type, so let’s confirm your assumption.
Definition and Examples
Unsupervised learning is a model that requires no training data. The algorithm performs various tasks intuitively, reducing the need for your input.
Machine learning professionals can tap into many different unsupervised algorithms:
- K-means clustering
- Hierarchical clustering
- Gaussian Mixture Models
Applications in Various Industries
Unsupervised learning models are widespread across a range of industries. Like supervised solutions, they can accomplish virtually anything:
- Segment target audiences for marketing firms
- Grouping DNA characteristics for biology research organizations
- Detecting anomalies and fraud for banks and other financial enterprises
Reinforcement Learning
How many times have your teachers rewarded you for a job well done? By doing so, they reinforced your learning and encouraged you to keep going.
That’s precisely how reinforcement learning works.
Definition and Examples
Reinforcement learning is a model where an algorithm learns through experimentation. If its action yields a positive outcome, it receives an award and aims to repeat the action. Acts that result in negative outcomes are ignored.
If you want to spearhead the development of a reinforcement learning-based app, you can choose from the following algorithms:
- Markov Decision Process
- Bellman Equations
- Dynamic programming
Applications in Various Industries
Reinforcement learning goes hand in hand with a large number of industries. Take a look at the most common applications:
- Ad optimization for marketing businesses
- Image processing for graphic design
- Traffic control for government bodies
Deep Learning
When talking about machine learning algorithms, you also need to go through deep learning.
Definition and Examples
Surprising as it may sound, deep learning operates similarly to your brain. It’s comprised of at least three layers of linked nodes that carry out different operations. The idea of linked nodes may remind you of something. That’s right – your brain cells.
You can find numerous deep learning models out there, including these:
- Recurrent neural networks
- Deep belief networks
- Multilayer perceptrons
Applications in Various Industries
If you’re looking for a flexible algorithm, look no further than deep learning models. Their ability to help businesses take off is second-to-none:
- Creating 3D characters in video gaming and movie industries
- Visual recognition in telecommunications
- CT scans in healthcare
Popular Machine Learning Algorithms
Our guide has already listed some of the most popular machine-learning algorithms. However, don’t think that’s the end of the story. There are many other algorithms you should keep in mind if you want to gain a better understanding of this technology.
Linear Regression
Linear regression is a form of supervised learning. It’s a simple yet highly effective algorithm that can help polish any business operation in a heartbeat.
Definition and Examples
Linear regression aims to predict a value based on provided input. The trajectory of the prediction path is linear, meaning it has no interruptions. The two main types of this algorithm are:
- Simple linear regression
- Multiple linear regression
Applications in Various Industries
Machine learning algorithms have proved to be a real cash cow for many industries. That especially holds for linear regression models:
- Stock analysis for financial firms
- Anticipating sports outcomes
- Exploring the relationships of different elements to lower pollution
Logistic Regression
Next comes logistic regression. This is another type of supervised learning and is fairly easy to grasp.
Definition and Examples
Logistic regression models are also geared toward predicting certain outcomes. Two classes are at play here: a positive class and a negative class. If the model arrives at the positive class, it logically excludes the negative option, and vice versa.
A great thing about logistic regression algorithms is that they don’t restrict you to just one method of analysis – you get three of these:
- Binary
- Multinomial
- Ordinal
Applications in Various Industries
Logistic regression is a staple of many organizations’ efforts to ramp up their operations and strike a chord with their target audience:
- Providing reliable credit scores for banks
- Identifying diseases using genes
- Optimizing booking practices for hotels
Decision Trees
You need only look out the window at a tree in your backyard to understand decision trees. The principle is straightforward, but the possibilities are endless.
Definition and Examples
A decision tree consists of internal nodes, branches, and leaf nodes. Internal nodes specify the feature or outcome you want to test, whereas branches tell you whether the outcome is possible. Leaf nodes are the so-called end outcome in this system.
The four most common decision tree algorithms are:
- Reduction in variance
- Chi-Square
- ID3
- Cart
Applications in Various Industries
Many companies are in the gutter and on the verge of bankruptcy because they failed to raise their services to the expected standards. However, their luck may turn around if they apply decision trees for different purposes:
- Improving logistics to reach desired goals
- Finding clients by analyzing demographics
- Evaluating growth opportunities
Support Vector Machines
What if you’re looking for an alternative to decision trees? Support vector machines might be an excellent choice.
Definition and Examples
Support vector machines separate your data with surgically accurate lines. These lines divide the information into points close to and far away from the desired values. Based on their proximity to the lines, you can determine the outliers or desired outcomes.
There are as many support vector machines as there are specks of sand on Copacabana Beach (not quite, but the number is still considerable):
- Anova kernel
- RBF kernel
- Linear support vector machines
- Non-linear support vector machines
- Sigmoid kernel
Applications in Various Industries
Here’s what you can do with support vector machines in the business world:
- Recognize handwriting
- Classify images
- Categorize text
Neural Networks
The above deep learning discussion lets you segue into neural networks effortlessly.
Definition and Examples
Neural networks are groups of interconnected nodes that analyze training data previously provided by the user. Here are a few of the most popular neural networks:
- Perceptrons
- Convolutional neural networks
- Multilayer perceptrons
- Recurrent neural networks
Applications in Various Industries
Is your imagination running wild? That’s good news if you master neural networks. You’ll be able to utilize them in countless ways:
- Voice recognition
- CT scans
- Commanding unmanned vehicles
- Social media monitoring
K-means Clustering
The name “K-means” clustering may sound daunting, but no worries – we’ll break down the components of this algorithm into bite-sized pieces.
Definition and Examples
K-means clustering is an algorithm that categorizes data into a K-number of clusters. The information that ends up in the same cluster is considered related. Anything that falls beyond the limit of a cluster is considered an outlier.
These are the most widely used K-means clustering algorithms:
- Hierarchical clustering
- Centroid-based clustering
- Density-based clustering
- Distribution-based clustering
Applications in Various Industries
A bunch of industries can benefit from K-means clustering algorithms:
- Finding optimal transportation routes
- Analyzing calls
- Preventing fraud
- Criminal profiling
Principal Component Analysis
Some algorithms start from certain building blocks. These building blocks are sometimes referred to as principal components. Enter principal component analysis.
Definition and Examples
Principal component analysis is a great way to lower the number of features in your data set. Think of it like downsizing – you reduce the number of individual elements you need to manage to streamline overall management.
The domain of principal component analysis is broad, encompassing many types of this algorithm:
- Sparse analysis
- Logistic analysis
- Robust analysis
- Zero-inflated dimensionality reduction
Applications in Various Industries
Principal component analysis seems useful, but what exactly can you do with it? Here are a few implementations:
- Finding patterns in healthcare records
- Resizing images
- Forecasting ROI
Challenges and Limitations of Machine Learning Algorithms
No computer science field comes without drawbacks. Machine learning algorithms also have their fair share of shortcomings:
- Overfitting and underfitting – Overfitted applications fail to generalize training data properly, whereas under-fitted algorithms can’t map the link between training data and desired outcomes.
- Bias and variance – Bias causes an algorithm to oversimplify data, whereas variance makes it memorize training information and fail to learn from it.
- Data quality and quantity – Poor quality, too much, or too little data can render an algorithm useless.
- Computational complexity – Some computers may not have what it takes to run complex algorithms.
- Ethical considerations – Sourcing training data inevitably triggers privacy and ethical concerns.
Future Trends in Machine Learning Algorithms
If we had a crystal ball, it might say that future of machine learning algorithms looks like this:
- Integration with other technologies – Machine learning may be harmonized with other technologies to propel space missions and other hi-tech achievements.
- Development of new algorithms and techniques – As the amount of data grows, expect more algorithms to spring up.
- Increasing adoption in various industries – Witnessing the efficacy of machine learning in various industries should encourage all other industries to follow in their footsteps.
- Addressing ethical and social concerns – Machine learning developers may find a way to source information safely without jeopardizing someone’s privacy.
Machine Learning Can Expand Your Horizons
Machine learning algorithms have saved the day for many enterprises. By polishing customer segmentation, strategic decision-making, and security, they’ve allowed countless businesses to thrive.
With more machine learning breakthroughs in the offing, expect the impact of this technology to magnify. So, hit the books and learn more about the subject to prepare for new advancements.
Related posts
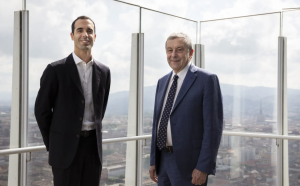
Source:
- EFMD Global, Published on July 12th, 2024.
By Stephanie Mullins
Many people love to read the stories of successful business school graduates to see what they’ve achieved using the lessons, insights and connections from the programmes they’ve studied. We speak to one alumnus, Riccardo Ocleppo, who studied at top business schools including London Business School (LBS) and INSEAD, about the education institution called OPIT which he created after business school.
Please introduce yourself and your career to date.
I am the founder of OPIT — Open Institute of Technology, a fully accredited Higher Education Institution (HEI) under the European Qualification Framework (EQF) by the MFHEA Authority. OPIT also partners with WES (World Education Services), a trusted non-profit providing verified education credential assessments (ECA) in the US and Canada for foreign degrees and certificates.
Prior to founding OPIT, I established Docsity, a global community boasting 15 million registered university students worldwide and partnerships with over 250 Universities and Business Schools. My academic background includes an MSc in Electronics from Politecnico di Torino and an MSc in Management from London Business School.
Why did you decide to create OPIT Open Institute of Technology?
Higher education has a profound impact on people’s futures. Through quality higher education, people can aspire to a better and more fulfilling future.
The mission behind OPIT is to democratise access to high-quality higher education in the fields that will be in high demand in the coming decades: Computer Science, Artificial Intelligence, Data Science, Cybersecurity, and Digital Innovation.
Since launching my first company in the education field, I’ve engaged with countless students, partnered with hundreds of universities, and collaborated with professors and companies. Through these interactions, I’ve observed a gap between traditional university curricula and the skills demanded by today’s job market, particularly in Computer Science and Technology.
I founded OPIT to bridge this gap by modernising education, making it affordable, and enhancing the digital learning experience. By collaborating with international professors and forging solid relationships with global companies, we are creating a dynamic online community and developing high-quality digital learning content. This approach ensures our students benefit from a flexible, cutting-edge, and stress-free learning environment.
Why do you think an education in tech is relevant in today’s business landscape?
As depicted by the World Economic Forum’s “Future of Jobs 2023” report, the demand for skilled tech professionals remains (and will remain) robust across industries, driven by the critical role of advanced technologies in business success.
Today’s companies require individuals who can innovate and execute complex solutions. A degree in fields like computer science, cybersecurity, data science, digital business or AI equips graduates with essential skills to thrive in this dynamic industry.
According to the International Monetary Fund (IMF), the global tech talent shortage will exceed 85 million workers by 2030. The Korn Ferry Institute warns that this gap could result in hundreds of billions in lost revenue across the US, Europe, and Asia.
To address this challenge, OPIT aims to democratise access to technology education. Our competency-based and applied approach, coupled with a flexible online learning experience, empowers students to progress at their own pace, demonstrating their skills as they advance.
Read the full article below:
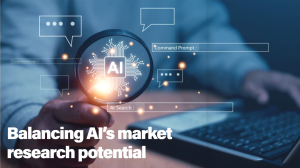
Source:
- The European, Summer 2024 Edition, Page 24
With careful planning, ethical considerations, and ensuring human oversight is maintained, AI can have huge market research benefits, says Lorenzo Livi of the Open Institute of Technology.
By Lorenzo Livi
To market well, you need to get something interesting in front of those who are interested. That takes a lot of thinking, a lot of work, and a whole bunch of research. But what if the bulk of that thinking, work and research could be done for you? What would that mean for marketing as an industry, and market research specifically?
With the recent explosion of AI onto the world stage, big changes are coming in the marketing industry. But will AI be able to do market research as successfully? Simply, the answer is yes. A big, fat, resounding yes. In fact, AI has the potential to revolutionise market research.
Ensuring that people have a clear understanding of what exactly AI is is crucial, given its seismic effect on our world. Common questions that even occur amongst people at the forefront of marketing, such as, “Who invented AI?” or, “Where is the main AI system located?” highlight a widespread misunderstanding about the nature of AI.
As for the notion of a central “main thing” running AI, it’s essential to clarify that AI systems exist in various forms and locations. AI algorithms and models can run on individual computers, servers, or even specialized hardware designed for AI processing, commonly referred to as AI chips. These systems can be distributed across multiple locations, including data centres, cloud platforms, and edge devices. They can also be used anywhere, so long as you have a compatible device and an internet connection.
While the concept of AI may seem abstract or mysterious to some, it’s important to approach it with a clear understanding of its principles and applications. By promoting education and awareness about AI, we can dispel misconceptions and facilitate meaningful conversations about its role in society.
Read the full article below:
- The European, Pages 24 to 26.
Have questions?
Visit our FAQ page or get in touch with us!
Write us at +39 335 576 0263
Get in touch at hello@opit.com
Talk to one of our Study Advisors
We are international
We can speak in: