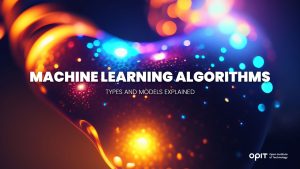
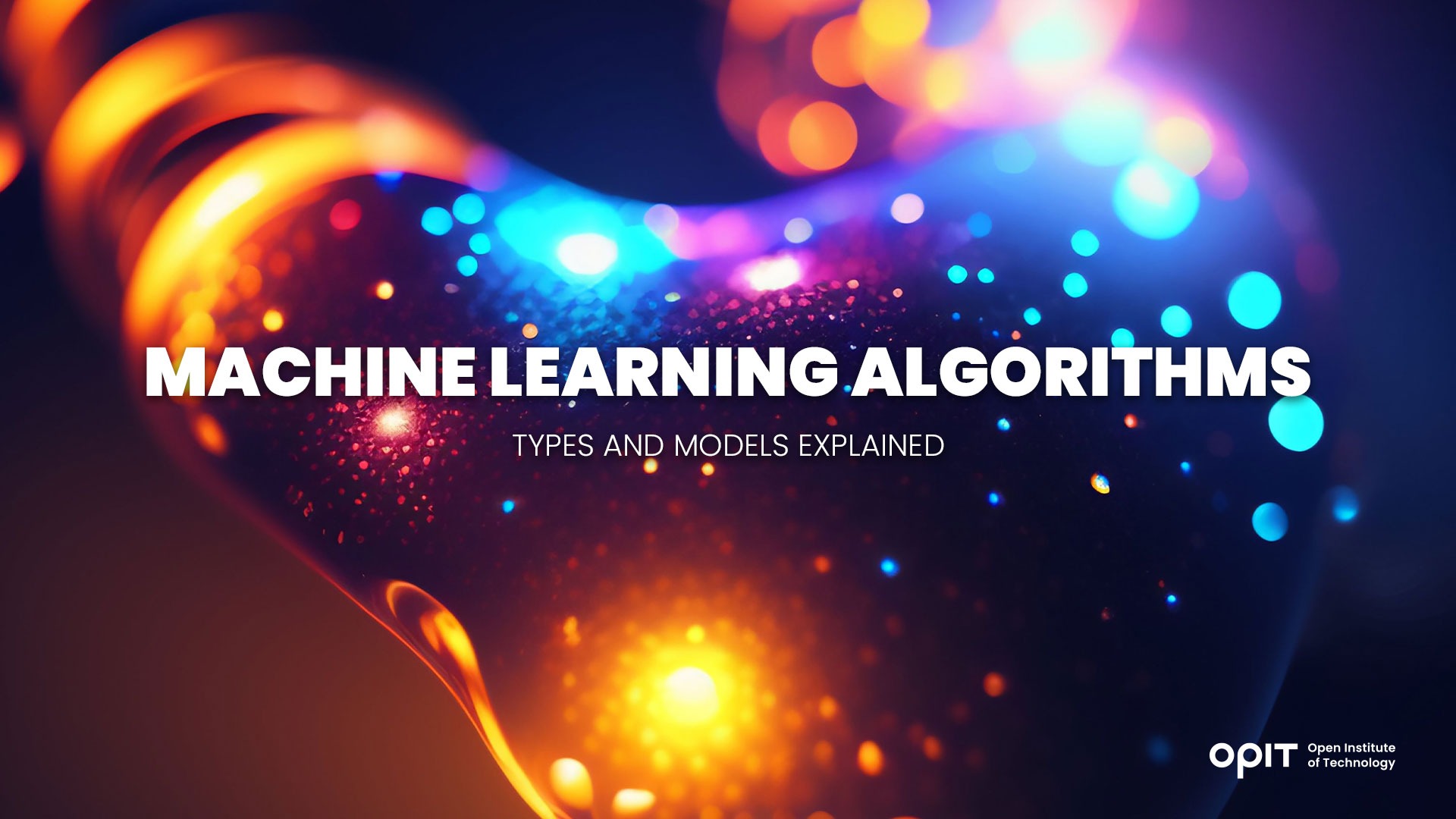
Any tendency or behavior of a consumer in the purchasing process in a certain period is known as customer behavior. For example, the last two years saw an unprecedented rise in online shopping. Such trends must be analyzed, but this is a nightmare for companies that try to take on the task manually. They need a way to speed up the project and make it more accurate.
Enter machine learning algorithms. Machine learning algorithms are methods AI programs use to complete a particular task. In most cases, they predict outcomes based on the provided information.
Without machine learning algorithms, customer behavior analyses would be a shot in the dark. These models are essential because they help enterprises segment their markets, develop new offerings, and perform time-sensitive operations without making wild guesses.
We’ve covered the definition and significance of machine learning, which only scratches the surface of this concept. The following is a detailed overview of the different types, models, and challenges of machine learning algorithms.
Types of Machine Learning Algorithms
A natural way to kick our discussion into motion is to dissect the most common types of machine learning algorithms. Here’s a brief explanation of each model, along with a few real-life examples and applications.
Supervised Learning
You can come across “supervised learning” at every corner of the machine learning realm. But what is it about, and where is it used?
Definition and Examples
Supervised machine learning is like supervised classroom learning. A teacher provides instructions, based on which students perform requested tasks.
In a supervised algorithm, the teacher is replaced by a user who feeds the system with input data. The system draws on this data to make predictions or discover trends, depending on the purpose of the program.
There are many supervised learning algorithms, as illustrated by the following examples:
- Decision trees
- Linear regression
- Gaussian Naïve Bayes
Applications in Various Industries
When supervised machine learning models were invented, it was like discovering the Holy Grail. The technology is incredibly flexible since it permeates a range of industries. For example, supervised algorithms can:
- Detect spam in emails
- Scan biometrics for security enterprises
- Recognize speech for developers of speech synthesis tools
Unsupervised Learning
On the other end of the spectrum of machine learning lies unsupervised learning. You can probably already guess the difference from the previous type, so let’s confirm your assumption.
Definition and Examples
Unsupervised learning is a model that requires no training data. The algorithm performs various tasks intuitively, reducing the need for your input.
Machine learning professionals can tap into many different unsupervised algorithms:
- K-means clustering
- Hierarchical clustering
- Gaussian Mixture Models
Applications in Various Industries
Unsupervised learning models are widespread across a range of industries. Like supervised solutions, they can accomplish virtually anything:
- Segment target audiences for marketing firms
- Grouping DNA characteristics for biology research organizations
- Detecting anomalies and fraud for banks and other financial enterprises
Reinforcement Learning
How many times have your teachers rewarded you for a job well done? By doing so, they reinforced your learning and encouraged you to keep going.
That’s precisely how reinforcement learning works.
Definition and Examples
Reinforcement learning is a model where an algorithm learns through experimentation. If its action yields a positive outcome, it receives an award and aims to repeat the action. Acts that result in negative outcomes are ignored.
If you want to spearhead the development of a reinforcement learning-based app, you can choose from the following algorithms:
- Markov Decision Process
- Bellman Equations
- Dynamic programming
Applications in Various Industries
Reinforcement learning goes hand in hand with a large number of industries. Take a look at the most common applications:
- Ad optimization for marketing businesses
- Image processing for graphic design
- Traffic control for government bodies
Deep Learning
When talking about machine learning algorithms, you also need to go through deep learning.
Definition and Examples
Surprising as it may sound, deep learning operates similarly to your brain. It’s comprised of at least three layers of linked nodes that carry out different operations. The idea of linked nodes may remind you of something. That’s right – your brain cells.
You can find numerous deep learning models out there, including these:
- Recurrent neural networks
- Deep belief networks
- Multilayer perceptrons
Applications in Various Industries
If you’re looking for a flexible algorithm, look no further than deep learning models. Their ability to help businesses take off is second-to-none:
- Creating 3D characters in video gaming and movie industries
- Visual recognition in telecommunications
- CT scans in healthcare
Popular Machine Learning Algorithms
Our guide has already listed some of the most popular machine-learning algorithms. However, don’t think that’s the end of the story. There are many other algorithms you should keep in mind if you want to gain a better understanding of this technology.
Linear Regression
Linear regression is a form of supervised learning. It’s a simple yet highly effective algorithm that can help polish any business operation in a heartbeat.
Definition and Examples
Linear regression aims to predict a value based on provided input. The trajectory of the prediction path is linear, meaning it has no interruptions. The two main types of this algorithm are:
- Simple linear regression
- Multiple linear regression
Applications in Various Industries
Machine learning algorithms have proved to be a real cash cow for many industries. That especially holds for linear regression models:
- Stock analysis for financial firms
- Anticipating sports outcomes
- Exploring the relationships of different elements to lower pollution
Logistic Regression
Next comes logistic regression. This is another type of supervised learning and is fairly easy to grasp.
Definition and Examples
Logistic regression models are also geared toward predicting certain outcomes. Two classes are at play here: a positive class and a negative class. If the model arrives at the positive class, it logically excludes the negative option, and vice versa.
A great thing about logistic regression algorithms is that they don’t restrict you to just one method of analysis – you get three of these:
- Binary
- Multinomial
- Ordinal
Applications in Various Industries
Logistic regression is a staple of many organizations’ efforts to ramp up their operations and strike a chord with their target audience:
- Providing reliable credit scores for banks
- Identifying diseases using genes
- Optimizing booking practices for hotels
Decision Trees
You need only look out the window at a tree in your backyard to understand decision trees. The principle is straightforward, but the possibilities are endless.
Definition and Examples
A decision tree consists of internal nodes, branches, and leaf nodes. Internal nodes specify the feature or outcome you want to test, whereas branches tell you whether the outcome is possible. Leaf nodes are the so-called end outcome in this system.
The four most common decision tree algorithms are:
- Reduction in variance
- Chi-Square
- ID3
- Cart
Applications in Various Industries
Many companies are in the gutter and on the verge of bankruptcy because they failed to raise their services to the expected standards. However, their luck may turn around if they apply decision trees for different purposes:
- Improving logistics to reach desired goals
- Finding clients by analyzing demographics
- Evaluating growth opportunities
Support Vector Machines
What if you’re looking for an alternative to decision trees? Support vector machines might be an excellent choice.
Definition and Examples
Support vector machines separate your data with surgically accurate lines. These lines divide the information into points close to and far away from the desired values. Based on their proximity to the lines, you can determine the outliers or desired outcomes.
There are as many support vector machines as there are specks of sand on Copacabana Beach (not quite, but the number is still considerable):
- Anova kernel
- RBF kernel
- Linear support vector machines
- Non-linear support vector machines
- Sigmoid kernel
Applications in Various Industries
Here’s what you can do with support vector machines in the business world:
- Recognize handwriting
- Classify images
- Categorize text
Neural Networks
The above deep learning discussion lets you segue into neural networks effortlessly.
Definition and Examples
Neural networks are groups of interconnected nodes that analyze training data previously provided by the user. Here are a few of the most popular neural networks:
- Perceptrons
- Convolutional neural networks
- Multilayer perceptrons
- Recurrent neural networks
Applications in Various Industries
Is your imagination running wild? That’s good news if you master neural networks. You’ll be able to utilize them in countless ways:
- Voice recognition
- CT scans
- Commanding unmanned vehicles
- Social media monitoring
K-means Clustering
The name “K-means” clustering may sound daunting, but no worries – we’ll break down the components of this algorithm into bite-sized pieces.
Definition and Examples
K-means clustering is an algorithm that categorizes data into a K-number of clusters. The information that ends up in the same cluster is considered related. Anything that falls beyond the limit of a cluster is considered an outlier.
These are the most widely used K-means clustering algorithms:
- Hierarchical clustering
- Centroid-based clustering
- Density-based clustering
- Distribution-based clustering
Applications in Various Industries
A bunch of industries can benefit from K-means clustering algorithms:
- Finding optimal transportation routes
- Analyzing calls
- Preventing fraud
- Criminal profiling
Principal Component Analysis
Some algorithms start from certain building blocks. These building blocks are sometimes referred to as principal components. Enter principal component analysis.
Definition and Examples
Principal component analysis is a great way to lower the number of features in your data set. Think of it like downsizing – you reduce the number of individual elements you need to manage to streamline overall management.
The domain of principal component analysis is broad, encompassing many types of this algorithm:
- Sparse analysis
- Logistic analysis
- Robust analysis
- Zero-inflated dimensionality reduction
Applications in Various Industries
Principal component analysis seems useful, but what exactly can you do with it? Here are a few implementations:
- Finding patterns in healthcare records
- Resizing images
- Forecasting ROI
Challenges and Limitations of Machine Learning Algorithms
No computer science field comes without drawbacks. Machine learning algorithms also have their fair share of shortcomings:
- Overfitting and underfitting – Overfitted applications fail to generalize training data properly, whereas under-fitted algorithms can’t map the link between training data and desired outcomes.
- Bias and variance – Bias causes an algorithm to oversimplify data, whereas variance makes it memorize training information and fail to learn from it.
- Data quality and quantity – Poor quality, too much, or too little data can render an algorithm useless.
- Computational complexity – Some computers may not have what it takes to run complex algorithms.
- Ethical considerations – Sourcing training data inevitably triggers privacy and ethical concerns.
Future Trends in Machine Learning Algorithms
If we had a crystal ball, it might say that future of machine learning algorithms looks like this:
- Integration with other technologies – Machine learning may be harmonized with other technologies to propel space missions and other hi-tech achievements.
- Development of new algorithms and techniques – As the amount of data grows, expect more algorithms to spring up.
- Increasing adoption in various industries – Witnessing the efficacy of machine learning in various industries should encourage all other industries to follow in their footsteps.
- Addressing ethical and social concerns – Machine learning developers may find a way to source information safely without jeopardizing someone’s privacy.
Machine Learning Can Expand Your Horizons
Machine learning algorithms have saved the day for many enterprises. By polishing customer segmentation, strategic decision-making, and security, they’ve allowed countless businesses to thrive.
With more machine learning breakthroughs in the offing, expect the impact of this technology to magnify. So, hit the books and learn more about the subject to prepare for new advancements.
Related posts
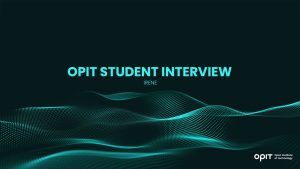
During the Open Institute of Technology’s (OPIT) 2025 graduation day, the OPIT team interviewed graduating student Irene about her experience with the MSc in Applied Data Science and AI. The interview focused on how Irene juggled working full-time with her study commitments and the value of the final Capstone project, which is part of all OPIT’s master’s programs.
Irene, a senior developer at ReActive, said she chose to study at OPIT to update her skills for the current and future job market.
OPIT’s MSc in Applied Data Science and AI
In her interview, Irene said she appreciated how OPIT’s course did not focus purely on the hard mathematics behind technologies such as AI and cloud computing, but also on how these technologies can be applied to real business challenges.
She said she appreciated how the course gave her the skills to explain to stakeholders with limited technical knowledge how technology can be leveraged to solve business problems, but it also equipped her to engage with technical teams using their language and jargon. These skills help graduates bridge the gap between management and technology to drive innovation and transformation.
Irene chose to continue working full-time while studying and appreciated how her course advisor helped her plan her study workload around her work commitments “down to the minute” so that she never missed a deadline or was overcome by excessive stress.
She said she would recommend the program to people at any stage in their career who want to adapt to the current job market. She also praised the international nature of the program, in terms of both the faculty and the cohort, as working beyond borders promises to be another major business trend in the coming years.
Capstone Project
Irene described the most fulfilling part of the program as the final Capstone project, which allowed her to apply what she had learned to a real-life challenge.
The Capstone Project and Dissertation, also called the MSc Thesis, is a significant project aimed at consolidating skills acquired during the program through a long-term research project.
Students, with the help of an OPIT supervisor, develop and realize a project proposal as part of the final term of their master’s journey, investigating methodological and practical aspects in program domains. Internships with industrial partners to deliver the project are encouraged and facilitated by OPIT’s staff.
The Capstone project allows students to demonstrate their mastery of their field and the skills they’ve learned when talking to employers as part of the hiring process.
Capstone Project: AI Meets Art
Irene’s Capstone project, “Call Me VasarAI: An AI-Powered Framework for Artwork Recognition and Storytelling,” focused on using AI to bridge the gap between art and artificial intelligence over time, enhancing meaning through contextualization. She developed an AI-powered platform that allows users to upload a work of art and discover the style (e.g. Expressionism), the name of the artist, and a description of the artwork within an art historical context.
Irene commented on how her supervisor helped her fine-tune her ideas into a stronger project and offered continuous guidance throughout the process with weekly progress updates. After defending her thesis in January, she noted how the examiners did not just assess her work but guided her on what could be next.
Other Example Capstone Projects
Irene’s success is just one example of a completed OPIT Capstone project. Below are further examples of both successful projects and projects currently underway.
Elina delivered her Capstone project on predictive modeling of natural disasters using data science and machine learning techniques to analyze global trends in natural disasters and their relationships with climate change-related and socio-economic factors.
According to Elina: “This hands-on experience has reinforced my theoretical and practical abilities in data science and AI. I appreciate the versatility of these skills, which are valuable across many domains. This project has been challenging yet rewarding, showcasing the real-world impact of my academic learning and the interdisciplinary nature of data science and AI.”
For his Capstone project, Musa worked on finding the optimal pipeline to fine-tune a language learning model (LLM) based on the specific language and model, considering EU laws on technological topics such as GDPR, DSA, DME, and the AI Act, which are translated into several languages.
Musa stated: “This Capstone project topic aligns perfectly with my initial interests when applying to OPIT. I am deeply committed to developing a pipeline in the field of EU law, an area that has not been extensively explored yet.”
Tamas worked with industry partner Solergy on his Capstone project, working with generative AI to supercharge lead generation, boost SEO performance, and deliver data-driven marketing insights in the realm of renewable energy.
OPIT’s Master’s Courses
All of OPIT’s master’s courses include a final Capstone project to be completed over one 13-week term in the 90 ECTS program and over two terms in the 120 ECTS program.
The MSc in Digital Business and Innovation is designed for professionals who want to drive digital innovation in both established companies and new digital-native contexts. It covers digital business foundations and the applications of new technologies in business contexts. It emphasizes the use of AI to drive innovation and covers digital entrepreneurship, digital product management, and growth hacking.
The MSc in Responsible Artificial Intelligence combines technical expertise with a focus on the ethical implications of modern AI. It focuses on real-world applications in areas like natural language processing and industry automation, with a focus on sustainable AI systems and environmental impact.
The MSc in Enterprise Cybersecurity prepares students to fulfill the market need for versatile cybersecurity solutions, emphasizing hands-on experience and soft-skills development.
The MSc in Applied Data Science and AI focuses on the intersection between management and technology. It covers the underlying fundamentals, methodologies and tools needed to solve real-life business problems that can be approached using data science and AI.
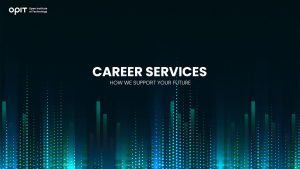
In May 2025, Greta Maiocchi, Head of Marketing and Administration at the Open Institute of Technology (OPIT), went online with Stefania Tabi, OPIT Career Services Counselor, to discuss how OPIT helps students translate their studies into a career.
You can access OPIT Career Services throughout your course of study to help with making the transition from student to professional. Stefania specifically discussed what companies and businesses are looking for and how OPIT Career Services can help you stand out and find a desirable career with your degree.
What Companies Want
OPIT degrees are tailored to a wide range of individuals, with bachelor’s degrees for those looking to establish a career and master’s degrees for experienced professionals hoping to elevate their skills to meet the current market demand.
OPIT’s degrees establish the foundation of the key technological skills that are set to reshape industries shortly, in particular artificial intelligence (AI), big data, cloud computing, and cybersecurity.
Stefania shared how companies recruiting tech talent are looking for three types of skills:
- Builders – These are the superstars of the industry today, capable of developing the technologies that will transform the industry. These roles include AI engineers, cloud architects, and web developers.
- Protectors – Cybercrime is expected to cost the world $10.5 trillion by the end of 2025, which means companies place a high value on cybersecurity professionals capable of protecting their investment, data, and intellectual property (IP).
- Decoders – Industry is producing more data than ever before, with global data storage projected to exceed 200 zettabytes this year. Businesses seek professionals who can extract value from that data, such as data scientists and data strategists.
Growing Demand
Stefania also shared statistics about the growing demand for these roles. According to the World Economic Forum, there will be a 30-35% greater demand for roles such as data analysts and scientists, big data specialists, business intelligence analysts, data engineers, and database and network professionals by 2027.
The U.S. Bureau of Labor Statistics, meanwhile, predicts that by 2032, the demand for information security will increase by 33.8%, by 21.5% for software developers, by 10.4% for computer network architects, and by 9.9% for computer system analysts. Finally, the McKinsey Global Institute predicts a similar 15-25% increase in demand for technology professionals in the business services sector.
How Career Support Makes a Difference
Next, Stefania explained that while learning essential skills is vital to accessing this growing job market, high demand does not guarantee entry. Today, professionals looking for jobs in the technology field must stand out from the hundreds of applicants for each position with high-level skills.
Applicants demonstrate technical expertise in relevant fields by completing OPIT’s courses. They also need to prove that they can deliver results, demonstrating not just what they know but how they have applied what they know to transform or benefit a business. Professionals also need adaptability, adaptive problem-solving skills, and a commitment to continuous learning. OPIT’s final Capstone projects can be an excellent way to demonstrate the value of newly acquired skills.
Each OPIT program prepares students for future careers by providing dedicated support and academic guidance at every step.
What Kind of Support Does Career Services Offer?
Career Services is specifically focused on assisting students in making the transition to the job market, and you can make an appointment with them at any time during your studies. Stefania gave some specific examples of how Career Services can support students on their journey into the career market.
Stefania said she begins by talking with students and discussing what they truly value to help them discover the type of career that aligns with their strengths. With students who are still undecided on how to start to build their careers, she helps them craft a tailored job and internship search plan.
Stefania has also worked with students who want to stand out during the job application process among the hundreds of applicants. This includes hands-on help in reframing resumes, tailoring LinkedIn profiles, and developing cover letters that tell a unique story.
Finally, Stefania has assisted students in preparing for interviews, helping them research the company, develop intelligent questions about the role to ask the interviewer and engage in mock interviews with an experienced recruiter.
Connecting With Employers
OPIT Career Services also offers students exposure to a wide range of employers and the opportunity to build relationships through masterclasses, career talks, and industry roundtables. The office also helps students build career-ready skills through interactive, hands-on workshops and hosts virtual career fairs with top recruiters.
Career Services also plays an integral role in connecting students with companies for their Capstone project in the final phase of their master’s program. So far, students have worked with companies including Sintica, Cosmica, Cisco, PayPal, Morgan Stanley, AWS, Dylog, and Accenture. Projects have included developing predictive modeling for natural disasters and fine-tuning AI to answer questions about EU tech laws in multiple languages.
What Kinds of Jobs Have OPIT Graduates Secured?
Stefania capped off her talk by sharing some of the positions that OPIT graduates have now fulfilled, including:
- Chief Information Security Officer at MOMO for MTN mobile services in Nigeria
- Data Analyst at ISX Financial in Cyprus
- Head of Sustainability Office at Banca Popolare di Sondrio in Italy
- Data Analyst at Numisma Group in Cyprus
- Senior Software Engineer at Neaform in Italy
OPIT Courses
OPIT offers both foundational bachelor’s degrees and advanced master’s courses, which are both accessible with any bachelor’s degree (it does not have to be in the field of computer science).
Choose between a BSc in Modern Computer Science for a strong technical base or a BSc in Digital Business to focus on applications.
Meanwhile, courses that involve a final Capstone project include an MSc in Applied Data Science and AI, Digital Business and Innovation, Enterprise Cybersecurity, and Responsible Artificial Intelligence.
Have questions?
Visit our FAQ page or get in touch with us!
Write us at +39 335 576 0263
Get in touch at hello@opit.com
Talk to one of our Study Advisors
We are international
We can speak in: