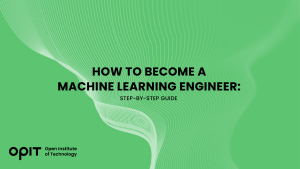
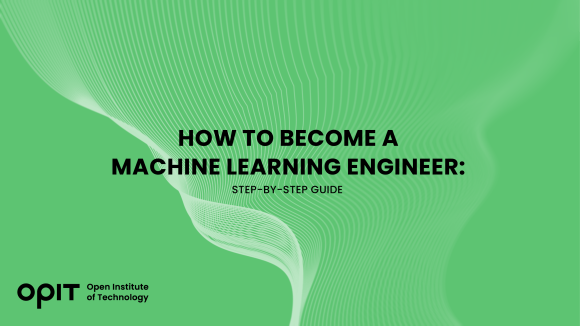
The demand for machine learning engineers is head-spinningly high. If you’re here, you are probably wondering how to become a machine learning engineer, the magic behind machine learning, and the savvy people behind it. In this domain, innovation meets practicality. Let’s unfold what it entails and why the demand has skyrocketed.
What Does a Machine Learning Engineer Do?
A machine learning engineer is the backbone of creating systems that can learn and make decisions with minimal human intervention. As a simple example, you could be teaching a machine to recognize a cat in a video or predict the next big trend in stock markets.
A machine learning engineer must perform a variety of tasks — from designing predictive models and fine-tuning their accuracy to deploying algorithms that can scale. They manipulate massive datasets, extract meaningful insights, and constantly learn to keep up with new advancements in the field.
You might wonder, “What are the machine learning engineer requirements?”
The requirements to become a machine learning engineer aren’t just about having a knack for programming or being great at math. Of course, those skills are necessary, but there’s more to it. You need to be curious, resilient, and eager to solve complex problems. Being able to communicate your findings and work collaboratively with others is just as big of a part of becoming a pro in machine learning. After all, what’s the use of a breakthrough if you can’t share it with others?
Educational Requirements to Become a Machine Learning Engineer
University degrees in computer science, data science, or Artificial Intelligence will give you a solid foundation. They cover everything from the basics of programming to the complexities of algorithms and data structures. Conversely, online or offline certifications might not be quite as comprehensive, but they make up for it by being more focused. Platforms for learning online also give you an in-depth look into machine learning specifics at your own pace.
Comparing the two, degrees offer a broad understanding and are great for foundational knowledge. At the same time, certifications can be seen as a bonus, providing specialized skills and up-to-date industry practices. Both paths have merits, and often, the best thing to do is to blend both. For a more detailed comparison, take a look at the article “Machine Learning Engineer Degree.”
Key Skills for Aspiring Machine Learning Engineers
First, your technical toolkit should include:
- Programming languages like Python or R
- Knowledge of algorithms
- Data modeling
These skills are the bread and butter that let you build and refine machine learning models that can tackle real-world problems.
But something to remember is that being technically adept isn’t enough. How to become a good machine learning engineer also hinges on your soft skills, such as:
- Communication
- Teamwork
- Resilience
- Problem-solving
The ability to communicate complex ideas clearly, work effectively in teams, and stay resilient in the face of debugging nightmares, along with problem-solving skills, are paramount. After all, you’ll be solving new puzzles every day. Also, while all these technical skills make for a terrific mix, you need creativity and curiosity. They fuel your innovations and discoveries in the ever-evolving field.
Building Experience in Machine Learning Engineering
Here are a few avenues to explore when building a machine-learning experience:
- Internships. There’s no substitute for real-world experience, and internships give you exactly that. They bring you face-to-face with the industry’s challenges and learning opportunities under the guidance of experienced mentors.
- Personal projects. If you’ve ever had an idea for a machine learning project, now’s the time to bring it to life. Personal projects are not only a fantastic way to test your skills but also to showcase your creativity and passion to potential employers.
- Open-source projects. Joining open-source projects can be a win-win. You get to contribute to meaningful projects, learn from the community, and make your mark in the field. It’s networking and learning all rolled into one.
Advancing Your Career With Specialized Machine Learning Knowledge
There’s always something new to learn in neural networks and AI. Specializations help you stand out in a field that’s very much in demand, and advanced education programs take you there. Deep learning, natural language processing, computer vision, robotics, reinforcement learning, and AI ethics are just some examples of potential specializations.
OPIT’s Master’s and Bachelor’s Programs are perfect examples of knowledge that’s equally deep and broad:
- MSc in Responsible Artificial Intelligence focuses on the ethical and societal impacts of AI. It prepares you to create technology that’s responsible as it’s advanced.
- BSc in Modern Computer Science provides a comprehensive foundation in computer science with a focus on modern developments, including machine learning.
- MSc in Applied Data Science & AI is for those who want to deepen their expertise in data science and AI, blending theory with practical application.
Enhancing Credibility With Machine Learning Certifications and Networking
Industry-recognized certifications polish your resume and, perhaps more crucially, signal your commitment and expertise to prospective employers, showing that you have the knowledge the industry feels is valuable. And let’s not forget the power of networking. Connecting with peers and mentors can open doors you never knew existed.
Career Prospects for Machine Learning Engineers
The horizon for machine learning engineers is vast and varied. Every sector, from tech giants to startups, is on the lookout for talent that can harness machine learning.
Healthcare, finance, tech, and even agriculture companies are eager to leverage AI to gain an edge. As a machine learning engineer, you could:
- Design algorithms to personalize content on streaming platforms
- Improve patient diagnoses in healthcare
- Predict client spending habits in banking and finances
- Optimize crop yields in agriculture
The variety of roles means there’s room for specialists and generalists alike. From data scientists and AI researchers to ML developers, the career paths are as diverse as the challenges you’ll tackle.
Partnering With OPIT for Your Machine Learning Engineering Journey
The right partner for your education can make all the difference, and OPIT is a beacon for aspiring machine learning engineers. OPIT offers a gateway to the future of tech through the following degrees:
- MSc in Responsible Artificial Intelligence that teaches you about AI ethics implications and social responsibilities and emphasizes real-world application.
- BSc in Modern Computer Science gives you a solid base in computing, opening doors to machine learning.
- MSc in Applied Data Science and AI is a program with hands-on projects that meet cutting-edge theory.
OPIT’s edge is in bridging in-depth learning and practical experience, minus the heavy-handedness of traditional schools and final exams.
Why Should You Become a Machine Learning Engineer?
The path to becoming a machine learning engineer is as exciting as it is rewarding, financially and professionally. As you learn, you’ll be coding in Python, untangling data, and figuring out how to make machines smarter. Yet, none of this would be enough without “softer” leadership, problem-solving, and communication skills.
With OPIT by your side and its master’s degrees in Responsible Artificial Intelligence, Modern Computer Science, and Applied Data Science and AI, you’re ready to take the future by storm.
Related posts
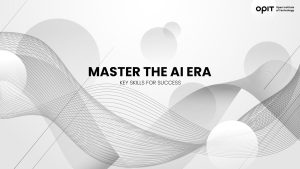
The world is rapidly changing. New technologies such as artificial intelligence (AI) are transforming our lives and work, redefining the definition of “essential office skills.”
So what essential skills do today’s workers need to thrive in a business world undergoing a major digital transformation? It’s a question that Alan Lerner, director at Toptal and lecturer at the Open Institute of Technology (OPIT), addressed in his recent online masterclass.
In a broad overview of the new office landscape, Lerner shares the essential skills leaders need to manage – including artificial intelligence – to keep abreast of trends.
Here are eight essential capabilities business leaders in the AI era need, according to Lerner, which he also detailed in OPIT’s recent Master’s in Digital Business and Innovation webinar.
An Adapting Professional Environment
Lerner started his discussion by quoting naturalist Charles Darwin.
“It is not the strongest of the species that survives, nor the most intelligent that survives. It is the one that is the most adaptable to change.”
The quote serves to highlight the level of change that we are currently seeing in the professional world, said Lerner.
According to the World Economic Forum’s The Future of Jobs Report 2025, over the next five years 22% of the labor market will be affected by structural change – including job creation and destruction – and much of that change will be enabled by new technologies such as AI and robotics. They expect the displacement of 92 million existing jobs and the creation of 170 million new jobs by 2030.
While there will be significant growth in frontline jobs – such as delivery drivers, construction workers, and care workers – the fastest-growing jobs will be tech-related roles, including big data specialists, FinTech engineers, and AI and machine learning specialists, while the greatest decline will be in clerical and secretarial roles. The report also predicts that most workers can anticipate that 39% of their existing skill set will be transformed or outdated in five years.
Lerner also highlighted key findings in the Accenture Life Trends 2025 Report, which explores behaviors and attitudes related to business, technology, and social shifts. The report noted five key trends:
- Cost of Hesitation – People are becoming more wary of the information they receive online.
- The Parent Trap – Parents and governments are increasingly concerned with helping the younger generation shape a safe relationship with digital technology.
- Impatience Economy – People are looking for quick solutions over traditional methods to achieve their health and financial goals.
- The Dignity of Work – Employees desire to feel inspired, to be entrusted with agency, and to achieve a work-life balance.
- Social Rewilding – People seek to disconnect and focus on satisfying activities and meaningful interactions.
These are consumer and employee demands representing opportunities for change in the modern business landscape.
Key Capabilities for the AI Era
Businesses are using a variety of strategies to adapt, though not always strategically. According to McClean & Company’s HR Trends Report 2025, 42% of respondents said they are currently implementing AI solutions, but only 7% have a documented AI implementation strategy.
This approach reflects the newness of the technology, with many still unsure of the best way to leverage AI, but also feeling the pressure to adopt and adapt, experiment, and fail forward.
So, what skills do leaders need to lead in an environment with both transformation and uncertainty? Lerner highlighted eight essential capabilities, independent of technology.
Capability 1: Manage Complexity
Leaders need to be able to solve problems and make decisions under fast-changing conditions. This requires:
- Being able to look at and understand organizations as complex social-technical systems
- Keeping a continuous eye on change and adopting an “outside-in” vision of their organization
- Moving fast and fixing things faster
- Embracing digital literacy and technological capabilities
Capability 2: Leverage Networks
Leaders need to develop networks systematically to achieve organizational goals because it is no longer possible to work within silos. Leaders should:
- Use networks to gain insights into complex problems
- Create networks to enhance influence
- Treat networks as mutually rewarding relationships
- Develop a robust profile that can be adapted for different networks
Capability 3: Think and Act “Global”
Leaders should benchmark using global best practices but adapt them to local challenges and the needs of their organization. This requires:
- Identifying what great companies are achieving and seeking data to understand underlying patterns
- Developing perspectives to craft global strategies that incorporate regional and local tactics
- Learning how to navigate culturally complex and nuanced business solutions
Capability 4: Inspire Engagement
Leaders must foster a culture that creates meaningful connections between employees and organizational values. This means:
- Understanding individual values and needs
- Shaping projects and assignments to meet different values and needs
- Fostering an inclusive work environment with plenty of psychological safety
- Developing meaningful conversations and both providing and receiving feedback
- Sharing advice and asking for help when needed
Capability 5: Communicate Strategically
Leaders should develop crisp, clear messaging adaptable to various audiences and focus on active listening. Achieving this involves:
- Creating their communication style and finding their unique voice
- Developing storytelling skills
- Utilizing a data-centric and fact-based approach to communication
- Continual practice and asking for feedback
Capability 6: Foster Innovation
Leaders should collaborate with experts to build a reliable innovation process and a creative environment where new ideas thrive. Essential steps include:
- Developing or enhancing structures that best support innovation
- Documenting and refreshing innovation systems, processes, and practices
- Encouraging people to discover new ways of working
- Aiming to think outside the box and develop a growth mindset
- Trying to be as “tech-savvy” as possible
Capability 7: Cultivate Learning Agility
Leaders should always seek out and learn new things and not be afraid to ask questions. This involves:
- Adopting a lifelong learning mindset
- Seeking opportunities to discover new approaches and skills
- Enhancing problem-solving skills
- Reviewing both successful and unsuccessful case studies
Capability 8: Develop Personal Adaptability
Leaders should be focused on being effective when facing uncertainty and adapting to change with vigor. Therefore, leaders should:
- Be flexible about their approach to facing challenging situations
- Build resilience by effectively managing stress, time, and energy
- Recognize when past approaches do not work in current situations
- Learn from and capitalize on mistakes
Curiosity and Adaptability
With the eight key capabilities in mind, Lerner suggests that curiosity and adaptability are the key skills that everyone needs to thrive in the current environment.
He also advocates for lifelong learning and teaches several key courses at OPIT which can lead to a Bachelor’s Degree in Digital Business.
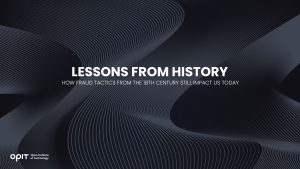
Many people treat cyber threats and digital fraud as a new phenomenon that only appeared with the development of the internet. But fraud – intentional deceit to manipulate a victim – has always existed; it is just the tools that have changed.
In a recent online course for the Open Institute of Technology (OPIT), AI & Cybersecurity Strategist Tom Vazdar, chair of OPIT’s Master’s Degree in Enterprise Cybersecurity, demonstrated the striking parallels between some of the famous fraud cases of the 18th century and modern cyber fraud.
Why does the history of fraud matter?
Primarily because the psychology and fraud tactics have remained consistent over the centuries. While cybersecurity is a tool that can combat modern digital fraud threats, no defense strategy will be successful without addressing the underlying psychology and tactics.
These historical fraud cases Vazdar addresses offer valuable lessons for current and future cybersecurity approaches.
The South Sea Bubble (1720)
The South Sea Bubble was one of the first stock market crashes in history. While it may not have had the same far-reaching consequences as the Black Thursday crash of 1929 or the 2008 crash, it shows how fraud can lead to stock market bubbles and advantages for insider traders.
The South Sea Company was a British company that emerged to monopolize trade with the Spanish colonies in South America. The company promised investors significant returns but provided no evidence of its activities. This saw the stock prices grow from £100 to £1,000 in a matter of months, then crash when the company’s weakness was revealed.
Many people lost a significant amount of money, including Sir Isaac Newton, prompting the statement, “I can calculate the movement of the stars, but not the madness of men.“
Investors often have no way to verify a company’s claim, making stock markets a fertile ground for manipulation and fraud since their inception. When one party has more information than another, it creates the opportunity for fraud. This can be seen today in Ponzi schemes, tech stock bubbles driven by manipulative media coverage, and initial cryptocurrency offerings.
The Diamond Necklace Affair (1784-1785)
The Diamond Necklace Affair is an infamous incident of fraud linked to the French Revolution. An early example of identity theft, it also demonstrates that the harm caused by such a crime can go far beyond financial.
A French aristocrat named Jeanne de la Mont convinced Cardinal Louis-René-Édouard, Prince de Rohan into thinking that he was buying a valuable diamond necklace on behalf of Queen Marie Antoinette. De la Mont forged letters from the queen and even had someone impersonate her for a meeting, all while convincing the cardinal of the need for secrecy. The cardinal overlooked several questionable issues because he believed he would gain political benefit from the transaction.
When the scheme finally exposed, it damaged Marie Antoinette’s reputation, despite her lack of involvement in the deception. The story reinforced the public perception of her as a frivolous aristocrat living off the labor of the people. This contributed to the overall resentment of the aristocracy that erupted in the French Revolution and likely played a role in Marie Antoinette’s death. Had she not been seen as frivolous, she might have been allowed to live after her husband’s death.
Today, impersonation scams work in similar ways. For example, a fraudster might forge communication from a CEO to convince employees to release funds or take some other action. The risk of this is only increasing with improved technology such as deepfakes.
Spanish Prisoner Scam (Late 1700s)
The Spanish Prisoner Scam will probably sound very familiar to anyone who received a “Nigerian prince” email in the early 2000s.
Victims received letters from a “wealthy Spanish prisoner” who needed their help to access his fortune. If they sent money to facilitate his escape and travel, he would reward them with greater riches when he regained his fortune. This was only one of many similar scams in the 1700s, often involving follow-up requests for additional payments before the scammer disappeared.
While the “Nigerian prince” scam received enough publicity that it became almost unbelievable that people could fall for it, if done well, these can be psychologically sophisticated scams. The stories play on people’s emotions, get them invested in the person, and enamor them with the idea of being someone helpful and important. A compelling narrative can diminish someone’s critical thinking and cause them to ignore red flags.
Today, these scams are more likely to take the form of inheritance fraud or a lottery scam, where, again, a person has to pay an advance fee to unlock a much bigger reward, playing on the common desire for easy money.
Evolution of Fraud
These examples make it clear that fraud is nothing new and that effective tactics have thrived over the centuries. Technology simply opens up new opportunities for fraud.
While 18th-century scammers had to rely on face-to-face contact and fraudulent letters, in the 19th century they could leverage the telegraph for “urgent” communication and newspaper ads to reach broader audiences. In the 20th century, there were telephones and television ads. Today, there are email, social media, and deepfakes, with new technologies emerging daily.
Rather than quack doctors offering miracle cures, we see online health scams selling diet pills and antiaging products. Rather than impersonating real people, we see fake social media accounts and catfishing. Fraudulent sites convince people to enter their bank details rather than asking them to send money. The anonymity of the digital world protects perpetrators.
But despite the technology changing, the underlying psychology that makes scams successful remains the same:
- Greed and the desire for easy money
- Fear of missing out and the belief that a response is urgent
- Social pressure to “keep up with the Joneses” and the “Bandwagon Effect”
- Trust in authority without verification
Therefore, the best protection against scams remains the same: critical thinking and skepticism, not technology.
Responding to Fraud
In conclusion, Vazdar shared a series of steps that people should take to protect themselves against fraud:
- Think before you click.
- Beware of secrecy and urgency.
- Verify identities.
- If it seems too good to be true, be skeptical.
- Use available security tools.
Those security tools have changed over time and will continue to change, but the underlying steps for identifying and preventing fraud remain the same.
For more insights from Vazdar and other experts in the field, consider enrolling in highly specialized and comprehensive programs like OPIT’s Enterprise Security Master’s program.
Have questions?
Visit our FAQ page or get in touch with us!
Write us at +39 335 576 0263
Get in touch at hello@opit.com
Talk to one of our Study Advisors
We are international
We can speak in: