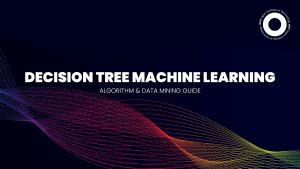
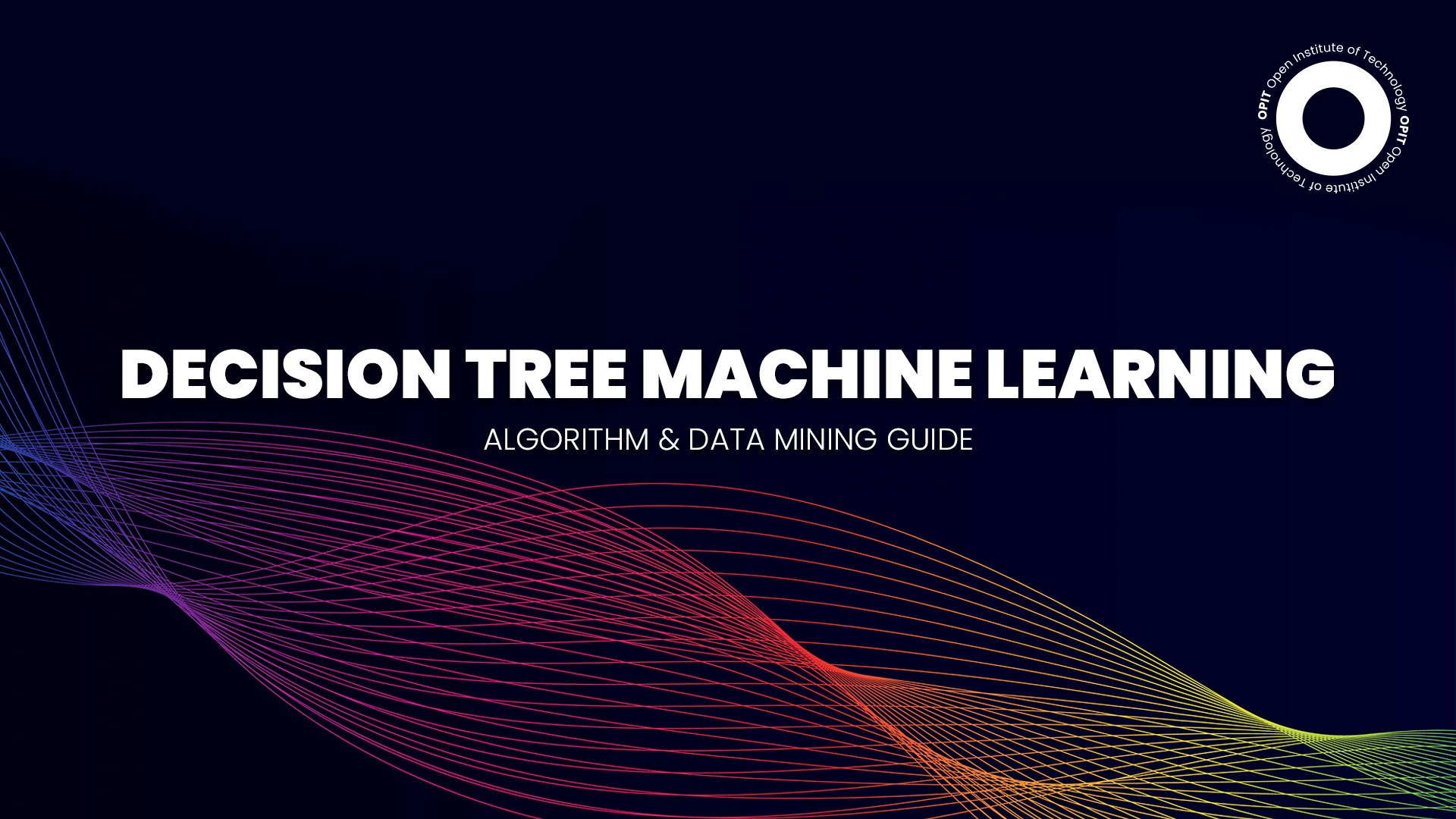
Algorithms are the essence of data mining and machine learning – the two processes 60% of organizations utilize to streamline their operations. Businesses can choose from several algorithms to polish their workflows, but the decision tree algorithm might be the most common.
This algorithm is all about simplicity. It branches out in multiple directions, just like trees, and determines whether something is true or false. In turn, data scientists and machine learning professionals can further dissect the data and help key stakeholders answer various questions.
This only scratches the surface of this algorithm – but it’s time to delve deeper into the concept. Let’s take a closer look at the decision tree machine learning algorithm, its components, types, and applications.
What Is Decision Tree Machine Learning?
The decision tree algorithm in data mining and machine learning may sound relatively simple due to its similarities with standard trees. But like with conventional trees, which consist of leaves, branches, roots, and many other elements, there’s a lot to uncover with this algorithm. We’ll start by defining this concept and listing the main components.
Definition of Decision Tree
If you’re a college student, you learn in two ways – supervised and unsupervised. The same division can be found in algorithms, and the decision tree belongs to the former category. It’s a supervised algorithm you can use to regress or classify data. It relies on training data to predict values or outcomes.
Components of Decision Tree
What’s the first thing you notice when you look at a tree? If you’re like most people, it’s probably the leaves and branches.
The decision tree algorithm has the same elements. Add nodes to the equation, and you have the entire structure of this algorithm right in front of you.
- Nodes – There are several types of nodes in decision trees. The root node is the parent of all nodes, which represents the overriding message. Chance nodes tell you the probability of a certain outcome, whereas decision nodes determine the decisions you should make.
- Branches – Branches connect nodes. Like rivers flowing between two cities, they show your data flow from questions to answers.
- Leaves – Leaves are also known as end nodes. These elements indicate the outcome of your algorithm. No more nodes can spring out of these nodes. They are the cornerstone of effective decision-making.
Types of Decision Trees
When you go to a park, you may notice various tree species: birch, pine, oak, and acacia. By the same token, there are multiple types of decision tree algorithms:
- Classification Trees – These decision trees map observations about particular data by classifying them into smaller groups. The chunks allow machine learning specialists to predict certain values.
- Regression Trees – According to IBM, regression decision trees can help anticipate events by looking at input variables.
Decision Tree Algorithm in Data Mining
Knowing the definition, types, and components of decision trees is useful, but it doesn’t give you a complete picture of this concept. So, buckle your seatbelt and get ready for an in-depth overview of this algorithm.
Overview of Decision Tree Algorithms
Just as there are hierarchies in your family or business, there are hierarchies in any decision tree in data mining. Top-down arrangements start with a problem you need to solve and break it down into smaller chunks until you reach a solution. Bottom-up alternatives sort of wing it – they enable data to flow with some supervision and guide the user to results.
Popular Decision Tree Algorithms
- ID3 (Iterative Dichotomiser 3) – Developed by Ross Quinlan, the ID3 is a versatile algorithm that can solve a multitude of issues. It’s a greedy algorithm (yes, it’s OK to be greedy sometimes), meaning it selects attributes that maximize information output.
- 5 – This is another algorithm created by Ross Quinlan. It generates outcomes according to previously provided data samples. The best thing about this algorithm is that it works great with incomplete information.
- CART (Classification and Regression Trees) – This algorithm drills down on predictions. It describes how you can predict target values based on other, related information.
- CHAID (Chi-squared Automatic Interaction Detection) – If you want to check out how your variables interact with one another, you can use this algorithm. CHAID determines how variables mingle and explain particular outcomes.
Key Concepts in Decision Tree Algorithms
No discussion about decision tree algorithms is complete without looking at the most significant concept from this area:
Entropy
As previously mentioned, decision trees are like trees in many ways. Conventional trees branch out in random directions. Decision trees share this randomness, which is where entropy comes in.
Entropy tells you the degree of randomness (or surprise) of the information in your decision tree.
Information Gain
A decision tree isn’t the same before and after splitting a root node into other nodes. You can use information gain to determine how much it’s changed. This metric indicates how much your data has improved since your last split. It tells you what to do next to make better decisions.
Gini Index
Mistakes can happen, even in the most carefully designed decision tree algorithms. However, you might be able to prevent errors if you calculate their probability.
Enter the Gini index (Gini impurity). It establishes the likelihood of misclassifying an instance when choosing it randomly.
Pruning
You don’t need every branch on your apple or pear tree to get a great yield. Likewise, not all data is necessary for a decision tree algorithm. Pruning is a compression technique that allows you to get rid of this redundant information that keeps you from classifying useful data.
Building a Decision Tree in Data Mining
Growing a tree is straightforward – you plant a seed and water it until it is fully formed. Creating a decision tree is simpler than some other algorithms, but quite a few steps are involved nevertheless.
Data Preparation
Data preparation might be the most important step in creating a decision tree. It’s comprised of three critical operations:
Data Cleaning
Data cleaning is the process of removing unwanted or unnecessary information from your decision trees. It’s similar to pruning, but unlike pruning, it’s essential to the performance of your algorithm. It’s also comprised of several steps, such as normalization, standardization, and imputation.
Feature Selection
Time is money, which especially applies to decision trees. That’s why you need to incorporate feature selection into your building process. It boils down to choosing only those features that are relevant to your data set, depending on the original issue.
Data Splitting
The procedure of splitting your tree nodes into sub-nodes is known as data splitting. Once you split data, you get two data points. One evaluates your information, while the other trains it, which brings us to the next step.
Training the Decision Tree
Now it’s time to train your decision tree. In other words, you need to teach your model how to make predictions by selecting an algorithm, setting parameters, and fitting your model.
Selecting the Best Algorithm
There’s no one-size-fits-all solution when designing decision trees. Users select an algorithm that works best for their application. For example, the Random Forest algorithm is the go-to choice for many companies because it can combine multiple decision trees.
Setting Parameters
How far your tree goes is just one of the parameters you need to set. You also need to choose between entropy and Gini values, set the number of samples when splitting nodes, establish your randomness, and adjust many other aspects.
Fitting the Model
If you’ve fitted your model properly, your data will be more accurate. The outcomes need to match the labeled data closely (but not too close to avoid overfitting) if you want relevant insights to improve your decision-making.
Evaluating the Decision Tree
Don’t put your feet up just yet. Your decision tree might be up and running, but how well does it perform? There are two ways to answer this question: cross-validation and performance metrics.
Cross-Validation
Cross-validation is one of the most common ways of gauging the efficacy of your decision trees. It compares your model to training data, allowing you to determine how well your system generalizes.
Performance Metrics
Several metrics can be used to assess the performance of your decision trees:
Accuracy
This is the proximity of your measurements to the requested values. If your model is accurate, it matches the values established in the training data.
Precision
By contrast, precision tells you how close your output values are to each other. In other words, it shows you how harmonized individual values are.
Recall
Recall is the number of data samples in the desired class. This class is also known as the positive class. Naturally, you want your recall to be as high as possible.
F1 Score
F1 score is the median value of your precision and recall. Most professionals consider an F1 of over 0.9 a very good score. Scores between 0.8 and 0.5 are OK, but anything less than 0.5 is bad. If you get a poor score, it means your data sets are imprecise and imbalanced.
Visualizing the Decision Tree
The final step is to visualize your decision tree. In this stage, you shed light on your findings and make them digestible for non-technical team members using charts or other common methods.
Applications of Decision Tree Machine Learning in Data Mining
The interest in machine learning is on the rise. One of the reasons is that you can apply decision trees in virtually any field:
- Customer Segmentation – Decision trees let you divide customers according to age, gender, or other factors.
- Fraud Detection – Decision trees can easily find fraudulent transactions.
- Medical Diagnosis – This algorithm allows you to classify conditions and other medical data with ease using decision trees.
- Risk Assessment – You can use the system to figure out how much money you stand to lose if you pursue a certain path.
- Recommender Systems – Decision trees help customers find their next product through classification.
Advantages and Disadvantages of Decision Tree Machine Learning
Advantages:
- Easy to Understand and Interpret – Decision trees make decisions almost in the same manner as humans.
- Handles Both Numerical and Categorical Data – The ability to handle different types of data makes them highly versatile.
- Requires Minimal Data Preprocessing – Preparing data for your algorithms doesn’t take much.
Disadvantages:
- Prone to Overfitting – Decision trees often fail to generalize.
- Sensitive to Small Changes in Data – Changing one data point can wreak havoc on the rest of the algorithm.
- May Not Work Well with Large Datasets – Naïve Bayes and some other algorithms outperform decision trees when it comes to large datasets.
Possibilities are Endless With Decision Trees
The decision tree machine learning algorithm is a simple yet powerful algorithm for classifying or regressing data. The convenient structure is perfect for decision-making, as it organizes information in an accessible format. As such, it’s ideal for making data-driven decisions.
If you want to learn more about this fascinating topic, don’t stop your exploration here. Decision tree courses and other resources can bring you one step closer to applying decision trees to your work.
Related posts
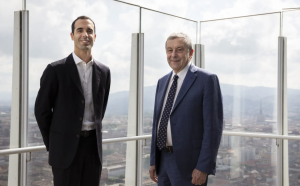
Source:
- EFMD Global, Published on July 12th, 2024.
By Stephanie Mullins
Many people love to read the stories of successful business school graduates to see what they’ve achieved using the lessons, insights and connections from the programmes they’ve studied. We speak to one alumnus, Riccardo Ocleppo, who studied at top business schools including London Business School (LBS) and INSEAD, about the education institution called OPIT which he created after business school.
Please introduce yourself and your career to date.
I am the founder of OPIT — Open Institute of Technology, a fully accredited Higher Education Institution (HEI) under the European Qualification Framework (EQF) by the MFHEA Authority. OPIT also partners with WES (World Education Services), a trusted non-profit providing verified education credential assessments (ECA) in the US and Canada for foreign degrees and certificates.
Prior to founding OPIT, I established Docsity, a global community boasting 15 million registered university students worldwide and partnerships with over 250 Universities and Business Schools. My academic background includes an MSc in Electronics from Politecnico di Torino and an MSc in Management from London Business School.
Why did you decide to create OPIT Open Institute of Technology?
Higher education has a profound impact on people’s futures. Through quality higher education, people can aspire to a better and more fulfilling future.
The mission behind OPIT is to democratise access to high-quality higher education in the fields that will be in high demand in the coming decades: Computer Science, Artificial Intelligence, Data Science, Cybersecurity, and Digital Innovation.
Since launching my first company in the education field, I’ve engaged with countless students, partnered with hundreds of universities, and collaborated with professors and companies. Through these interactions, I’ve observed a gap between traditional university curricula and the skills demanded by today’s job market, particularly in Computer Science and Technology.
I founded OPIT to bridge this gap by modernising education, making it affordable, and enhancing the digital learning experience. By collaborating with international professors and forging solid relationships with global companies, we are creating a dynamic online community and developing high-quality digital learning content. This approach ensures our students benefit from a flexible, cutting-edge, and stress-free learning environment.
Why do you think an education in tech is relevant in today’s business landscape?
As depicted by the World Economic Forum’s “Future of Jobs 2023” report, the demand for skilled tech professionals remains (and will remain) robust across industries, driven by the critical role of advanced technologies in business success.
Today’s companies require individuals who can innovate and execute complex solutions. A degree in fields like computer science, cybersecurity, data science, digital business or AI equips graduates with essential skills to thrive in this dynamic industry.
According to the International Monetary Fund (IMF), the global tech talent shortage will exceed 85 million workers by 2030. The Korn Ferry Institute warns that this gap could result in hundreds of billions in lost revenue across the US, Europe, and Asia.
To address this challenge, OPIT aims to democratise access to technology education. Our competency-based and applied approach, coupled with a flexible online learning experience, empowers students to progress at their own pace, demonstrating their skills as they advance.
Read the full article below:
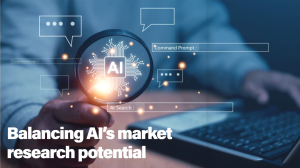
Source:
- The European, Summer 2024 Edition, Page 24
With careful planning, ethical considerations, and ensuring human oversight is maintained, AI can have huge market research benefits, says Lorenzo Livi of the Open Institute of Technology.
By Lorenzo Livi
To market well, you need to get something interesting in front of those who are interested. That takes a lot of thinking, a lot of work, and a whole bunch of research. But what if the bulk of that thinking, work and research could be done for you? What would that mean for marketing as an industry, and market research specifically?
With the recent explosion of AI onto the world stage, big changes are coming in the marketing industry. But will AI be able to do market research as successfully? Simply, the answer is yes. A big, fat, resounding yes. In fact, AI has the potential to revolutionise market research.
Ensuring that people have a clear understanding of what exactly AI is is crucial, given its seismic effect on our world. Common questions that even occur amongst people at the forefront of marketing, such as, “Who invented AI?” or, “Where is the main AI system located?” highlight a widespread misunderstanding about the nature of AI.
As for the notion of a central “main thing” running AI, it’s essential to clarify that AI systems exist in various forms and locations. AI algorithms and models can run on individual computers, servers, or even specialized hardware designed for AI processing, commonly referred to as AI chips. These systems can be distributed across multiple locations, including data centres, cloud platforms, and edge devices. They can also be used anywhere, so long as you have a compatible device and an internet connection.
While the concept of AI may seem abstract or mysterious to some, it’s important to approach it with a clear understanding of its principles and applications. By promoting education and awareness about AI, we can dispel misconceptions and facilitate meaningful conversations about its role in society.
Read the full article below:
- The European, Pages 24 to 26.
Have questions?
Visit our FAQ page or get in touch with us!
Write us at +39 335 576 0263
Get in touch at hello@opit.com
Talk to one of our Study Advisors
We are international
We can speak in: