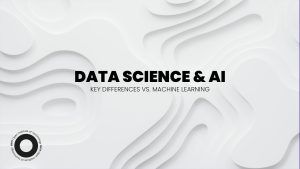
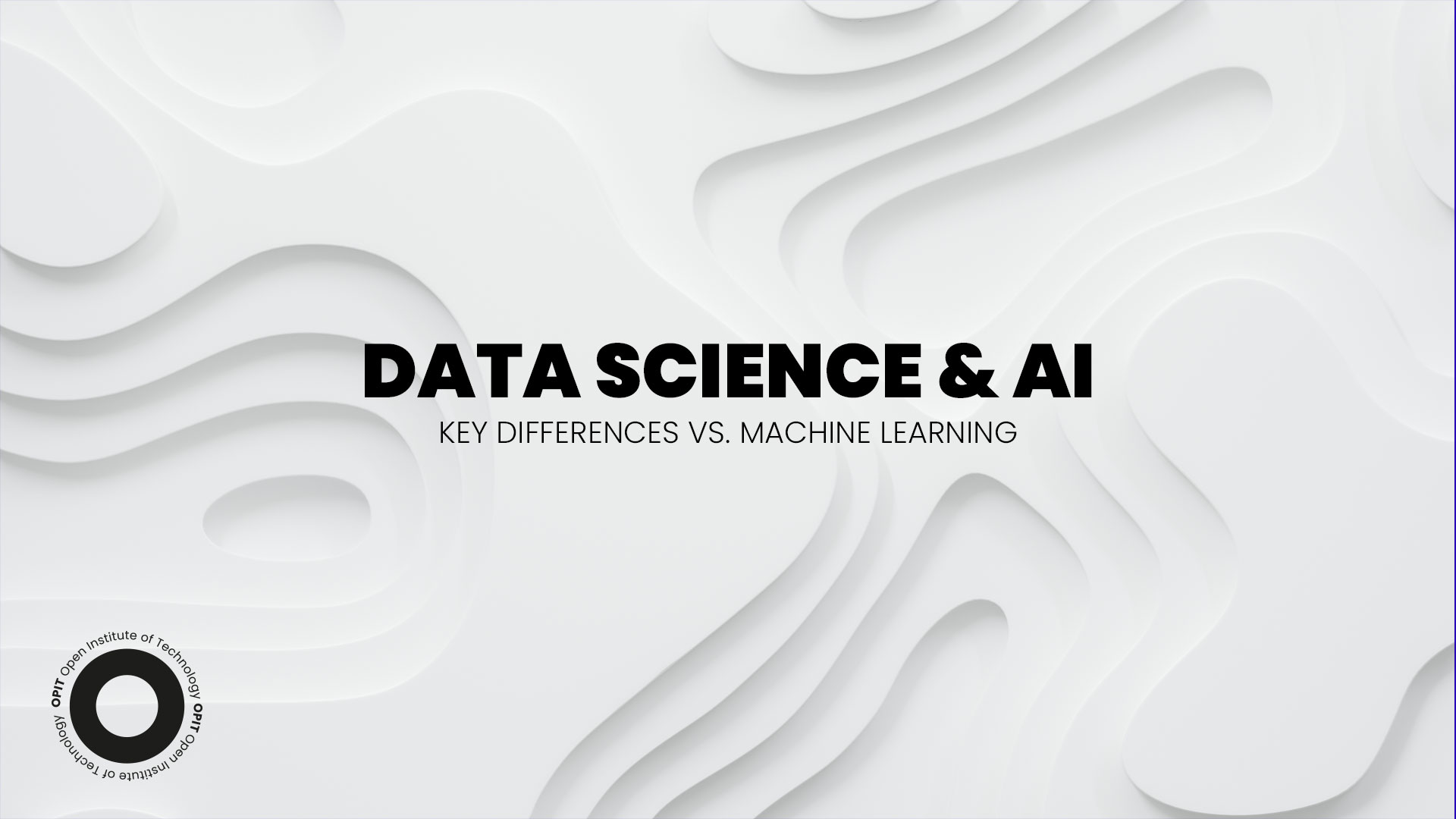
Machine learning, data science, and artificial intelligence are common terms in modern technology. These terms are often used interchangeably but incorrectly, which is understandable.
After all, hundreds of millions of people use the advantages of digital technologies. Yet only a small percentage of those users are experts in the field.
AI, data science, and machine learning represent valuable assets that can be used to great advantage in various industries. However, to use these tools properly, you need to understand what they are. Furthermore, knowing the difference between data science and machine learning, as well as how AI differs from both, can dispel the common misconceptions about these technologies.
Read on to gain a better understanding of the three crucial tech concepts.
Data Science
Data science can be viewed as the foundation of many modern technological solutions. It’s also the stage from which existing solutions can progress and evolve. Let’s define data science in more detail.
Definition and Explanation of Data Science
A scientific discipline with practical applications, data science represents a field of study dedicated to the development of data systems. If this definition sounds too broad, that’s because data science is a broad field by its nature.
Data structure is the primary concern of data science. To produce clean data and conduct analysis, scientists use a range of methods and tools, from manual to automated solutions.
Data science has another crucial task: defining problems that previously didn’t exist or slipped by unnoticed. Through this activity, data scientists can help predict unforeseen issues, improve existing digital tools, and promote the development of new ones.
Key Components of Data Science
Breaking down data science into key components, we get to three essential factors:
- Data collection
- Data analysis
- Predictive modeling
Data collection is pretty much what it sounds like – gathering of data. This aspect of data science also includes preprocessing, which is essentially preparation of raw data for further processing.
During data analysis, data scientists draw conclusions based on the gathered data. They search the data for patterns and potential flaws. The scientists do this to determine weak points and system deficiencies. In data visualization, scientists aim to communicate the conclusions of their investigation through graphics, charts, bullet points, and maps.
Finally, predictive modeling represents one of the ultimate uses of the analyzed data. Here, create models that can help them predict future trends. This component also illustrates the differentiation between data science vs. machine learning. Machine learning is often used in predictive modeling as a tool within the broader field of data science.
Applications and Use Cases of Data Science
Data science finds uses in marketing, banking, finance, logistics, HR, and trading, to name a few. Financial institutions and businesses take advantage of data science to assess and manage risks. The powerful assistance of data science often helps these organizations gain the upper hand in the market.
In marketing, data science can provide valuable information about customers, help marketing departments organize, and launch effective targeted campaigns. When it comes to human resources, extensive data gathering, and analysis allow HR departments to single out the best available talent and create accurate employee performance projections.
Artificial Intelligence (AI)
The term “artificial intelligence” has been somewhat warped by popular culture. Despite the varying interpretations, AI is a concrete technology with a clear definition and purpose, as well as numerous applications.
Definition and Explanation of AI
Artificial intelligence is sometimes called machine intelligence. In its essence, AI represents a machine simulation of human learning and decision-making processes.
AI gives machines the function of empirical learning, i.e., using experiences and observations to gain new knowledge. However, machines can’t acquire new experiences independently. They need to be fed relevant data for the AI process to work.
Furthermore, AI must be able to self-correct so that it can act as an active participant in improving its abilities.
Obviously, AI represents a rather complex technology. We’ll explain its key components in the following section.
Key Components of AI
A branch of computer science, AI includes several components that are either subsets of one another or work in tandem. These are machine learning, deep learning, natural language processing (NLP), computer vision, and robotics.
It’s no coincidence that machine learning popped up at the top spot here. It’s a crucial aspect of AI that does precisely what the name says: enables machines to learn.
We’ll discuss machine learning in a separate section.
Deep learning relates to machine learning. Its aim is essentially to simulate the human brain. To that end, the technology utilizes neural networks alongside complex algorithm structures that allow the machine to make independent decisions.
Natural language processing (NLP) allows machines to comprehend language similarly to humans. Language processing and understanding are the primary tasks of this AI branch.
Somewhat similar to NLP, computer vision allows machines to process visual input and extract useful data from it. And just as NLP enables a computer to understand language, computer vision facilitates a meaningful interpretation of visual information.
Finally, robotics are AI-controlled machines that can replace humans in dangerous or extremely complex tasks. As a branch of AI, robotics differs from robotic engineering, which focuses on the mechanical aspects of building machines.
Applications and Use Cases of AI
The variety of AI components makes the technology suitable for a wide range of applications. Machine and deep learning are extremely useful in data gathering. NLP has seen a massive uptick in popularity lately, especially with tools like ChatGPT and similar chatbots. And robotics has been around for decades, finding use in various industries and services, in addition to military and space applications.
Machine Learning
Machine learning is an AI branch that’s frequently used in data science. Defining what this aspect of AI does will largely clarify its relationship to data science and artificial intelligence.
Definition and Explanation of Machine Learning
Machine learning utilizes advanced algorithms to detect data patterns and interpret their meaning. The most important facets of machine learning include handling various data types, scalability, and high-level automation.
Like AI in general, machine learning also has a level of complexity to it, consisting of several key components.
Key Components of Machine Learning
The main aspects of machine learning are supervised, unsupervised, and reinforcement learning.
Supervised learning trains algorithms for data classification using labeled datasets. Simply put, the data is first labeled and then fed into the machine.
Unsupervised learning relies on algorithms that can make sense of unlabeled datasets. In other words, external intervention isn’t necessary here – the machine can analyze data patterns on its own.
Finally, reinforcement learning is the level of machine learning where the AI can learn to respond to input in an optimal way. The machine learns correct behavior through observation and environmental interactions without human assistance.
Applications and Use Cases of Machine Learning
As mentioned, machine learning is particularly useful in data science. The technology makes processing large volumes of data much easier while producing more accurate results. Supervised and particularly unsupervised learning are especially helpful here.
Reinforcement learning is most efficient in uncertain or unpredictable environments. It finds use in robotics, autonomous driving, and all situations where it’s impossible to pre-program machines with sufficient accuracy.
Perhaps most famously, reinforcement learning is behind AlphaGo, an AI program developed for the Go board game. The game is notorious for its complexity, having about 250 possible moves on each of 150 turns, which is how long a typical game lasts.
Alpha Go managed to defeat the human Go champion by getting better at the game through numerous previous matches.
Key Differences Between Data Science, AI, and Machine Learning
The differences between machine learning, data science, and artificial intelligence are evident in the scope, objectives, techniques, required skill sets, and application.
As a subset of AI and a frequent tool in data science, machine learning has a more closely defined scope. It’s structured differently to data science and artificial intelligence, both massive fields of study with far-reaching objectives.
The objectives of data science are pto gather and analyze data. Machine learning and AI can take that data and utilize it for problem-solving, decision-making, and to simulate the most complex traits of the human brain.
Machine learning has the ultimate goal of achieving high accuracy in pattern comprehension. On the other hand, the main task of AI in general is to ensure success, particularly in emulating specific facets of human behavior.
All three require specific skill sets. In the case of data science vs. machine learning, the sets don’t match. The former requires knowledge of SQL, ETL, and domains, while the latter calls for Python, math, and data-wrangling expertise.
Naturally, machine learning will have overlapping skill sets with AI, since it’s its subset.
Finally, in the application field, data science produces valuable data-driven insights, AI is largely used in virtual assistants, while machine learning powers search engine algorithms.
How Data Science, AI, and Machine Learning Complement Each Other
Data science helps AI and machine learning by providing accurate, valuable data. Machine learning is critical in processing data and functions as a primary component of AI. And artificial intelligence provides novel solutions on all fronts, allowing for more efficient automation and optimal processes.
Through the interaction of data science, AI, and machine learning, all three branches can develop further, bringing improvement to all related industries.
Understanding the Technology of the Future
Understanding the differences and common uses of data science, AI, and machine learning is essential for professionals in the field. However, it can also be valuable for businesses looking to leverage modern and future technologies.
As all three facets of modern tech develop, it will be important to keep an eye on emerging trends and watch for future developments.
Related posts
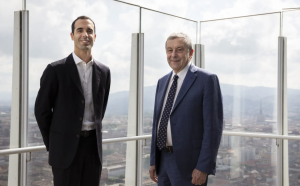
Source:
- EFMD Global, Published on July 12th, 2024.
By Stephanie Mullins
Many people love to read the stories of successful business school graduates to see what they’ve achieved using the lessons, insights and connections from the programmes they’ve studied. We speak to one alumnus, Riccardo Ocleppo, who studied at top business schools including London Business School (LBS) and INSEAD, about the education institution called OPIT which he created after business school.
Please introduce yourself and your career to date.
I am the founder of OPIT — Open Institute of Technology, a fully accredited Higher Education Institution (HEI) under the European Qualification Framework (EQF) by the MFHEA Authority. OPIT also partners with WES (World Education Services), a trusted non-profit providing verified education credential assessments (ECA) in the US and Canada for foreign degrees and certificates.
Prior to founding OPIT, I established Docsity, a global community boasting 15 million registered university students worldwide and partnerships with over 250 Universities and Business Schools. My academic background includes an MSc in Electronics from Politecnico di Torino and an MSc in Management from London Business School.
Why did you decide to create OPIT Open Institute of Technology?
Higher education has a profound impact on people’s futures. Through quality higher education, people can aspire to a better and more fulfilling future.
The mission behind OPIT is to democratise access to high-quality higher education in the fields that will be in high demand in the coming decades: Computer Science, Artificial Intelligence, Data Science, Cybersecurity, and Digital Innovation.
Since launching my first company in the education field, I’ve engaged with countless students, partnered with hundreds of universities, and collaborated with professors and companies. Through these interactions, I’ve observed a gap between traditional university curricula and the skills demanded by today’s job market, particularly in Computer Science and Technology.
I founded OPIT to bridge this gap by modernising education, making it affordable, and enhancing the digital learning experience. By collaborating with international professors and forging solid relationships with global companies, we are creating a dynamic online community and developing high-quality digital learning content. This approach ensures our students benefit from a flexible, cutting-edge, and stress-free learning environment.
Why do you think an education in tech is relevant in today’s business landscape?
As depicted by the World Economic Forum’s “Future of Jobs 2023” report, the demand for skilled tech professionals remains (and will remain) robust across industries, driven by the critical role of advanced technologies in business success.
Today’s companies require individuals who can innovate and execute complex solutions. A degree in fields like computer science, cybersecurity, data science, digital business or AI equips graduates with essential skills to thrive in this dynamic industry.
According to the International Monetary Fund (IMF), the global tech talent shortage will exceed 85 million workers by 2030. The Korn Ferry Institute warns that this gap could result in hundreds of billions in lost revenue across the US, Europe, and Asia.
To address this challenge, OPIT aims to democratise access to technology education. Our competency-based and applied approach, coupled with a flexible online learning experience, empowers students to progress at their own pace, demonstrating their skills as they advance.
Read the full article below:
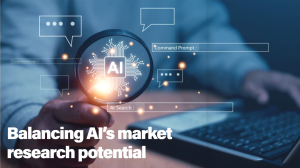
Source:
- The European, Summer 2024 Edition, Page 24
With careful planning, ethical considerations, and ensuring human oversight is maintained, AI can have huge market research benefits, says Lorenzo Livi of the Open Institute of Technology.
By Lorenzo Livi
To market well, you need to get something interesting in front of those who are interested. That takes a lot of thinking, a lot of work, and a whole bunch of research. But what if the bulk of that thinking, work and research could be done for you? What would that mean for marketing as an industry, and market research specifically?
With the recent explosion of AI onto the world stage, big changes are coming in the marketing industry. But will AI be able to do market research as successfully? Simply, the answer is yes. A big, fat, resounding yes. In fact, AI has the potential to revolutionise market research.
Ensuring that people have a clear understanding of what exactly AI is is crucial, given its seismic effect on our world. Common questions that even occur amongst people at the forefront of marketing, such as, “Who invented AI?” or, “Where is the main AI system located?” highlight a widespread misunderstanding about the nature of AI.
As for the notion of a central “main thing” running AI, it’s essential to clarify that AI systems exist in various forms and locations. AI algorithms and models can run on individual computers, servers, or even specialized hardware designed for AI processing, commonly referred to as AI chips. These systems can be distributed across multiple locations, including data centres, cloud platforms, and edge devices. They can also be used anywhere, so long as you have a compatible device and an internet connection.
While the concept of AI may seem abstract or mysterious to some, it’s important to approach it with a clear understanding of its principles and applications. By promoting education and awareness about AI, we can dispel misconceptions and facilitate meaningful conversations about its role in society.
Read the full article below:
- The European, Pages 24 to 26.
Have questions?
Visit our FAQ page or get in touch with us!
Write us at +39 335 576 0263
Get in touch at hello@opit.com
Talk to one of our Study Advisors
We are international
We can speak in: